Clever Firm Predicts Patients Most at Risk, Then Tries to Intervene Before They Get Sicker
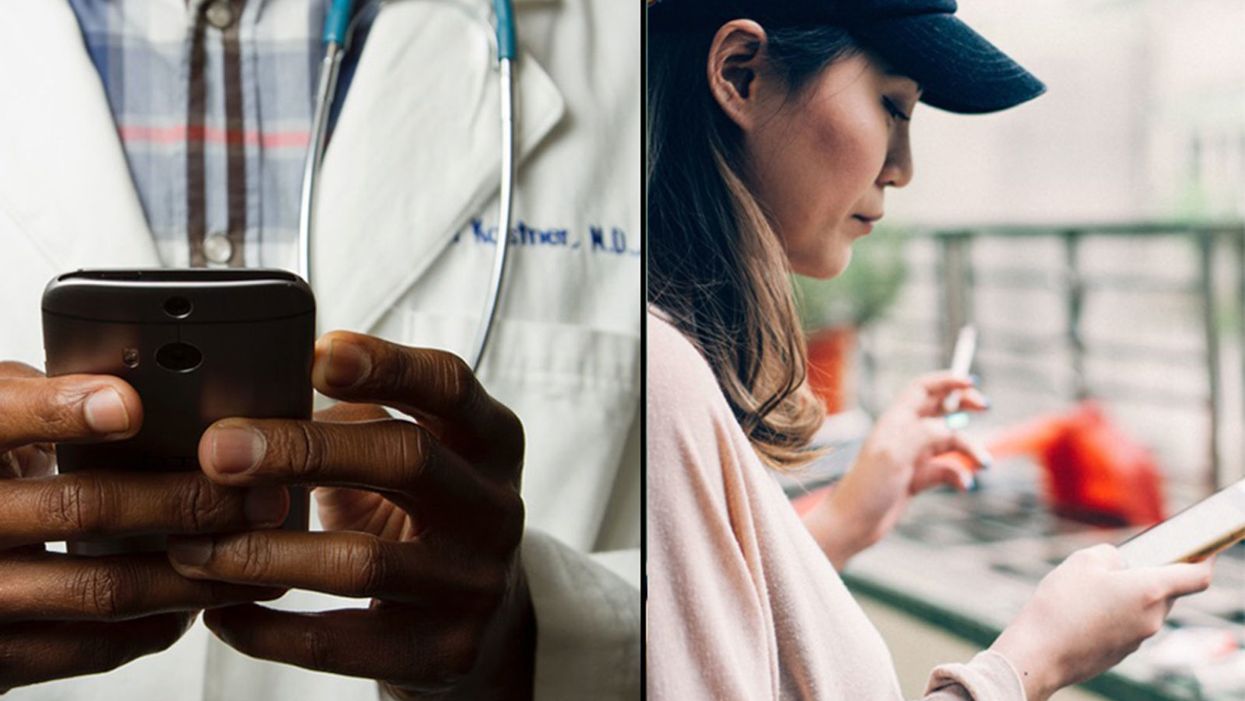
Health firm Populytics tracks and analyzes patient data, and makes care suggestions based on that data.
The diabetic patient hit the danger zone.
Ideally, blood sugar, measured by an A1C test, rests at 5.9 or less. A 7 is elevated, according to the Diabetes Council. Over 10, and you're into the extreme danger zone, at risk of every diabetic crisis from kidney failure to blindness.
In three months of working with a case manager, Jen's blood sugar had dropped to 7.2, a much safer range.
This patient's A1C was 10. Let's call her Jen for the sake of this story. (Although the facts of her case are real, the patient's actual name wasn't released due to privacy laws.).
Jen happens to live in Pennsylvania's Lehigh Valley, home of the nonprofit Lehigh Valley Health Network, which has eight hospital campuses and various clinics and other services. This network has invested more than $1 billion in IT infrastructure and founded Populytics, a spin-off firm that tracks and analyzes patient data, and makes care suggestions based on that data.
When Jen left the doctor's office, the Populytics data machine started churning, analyzing her data compared to a wealth of information about future likely hospital visits if she did not comply with recommendations, as well as the potential positive impacts of outreach and early intervention.
About a month after Jen received the dangerous blood test results, a community outreach specialist with psychological training called her. She was on a list generated by Populytics of follow-up patients to contact.
"It's a very gentle conversation," says Cathryn Kelly, who manages a care coordination team at Populytics. "The case manager provides them understanding and support and coaching." The goal, in this case, was small behavioral changes that would actually stick, like dietary ones.
In three months of working with a case manager, Jen's blood sugar had dropped to 7.2, a much safer range. The odds of her cycling back to the hospital ER or veering into kidney failure, or worse, had dropped significantly.
While the health network is extremely localized to one area of one state, using data to inform precise medical decision-making appears to be the wave of the future, says Ann Mongovern, the associate director of Health Care Ethics at the Markkula Center for Applied Ethics at Santa Clara University in California.
"Many hospitals and hospital systems don't yet try to do this at all, which is striking given where we're at in terms of our general technical ability in this society," Mongovern says.
How It Happened
While many hospitals make money by filling beds, the Lehigh Valley Health Network, as a nonprofit, accepts many patients on Medicaid and other government insurances that don't cover some of the costs of a hospitalization. The area's population is both poorer and older than national averages, according to the U.S. Census data, meaning more people with higher medical needs that may not have the support to care for themselves. They end up in the ER, or worse, again and again.
In the early 2000s, LVHN CEO Dr. Brian Nester started wondering if his health network could develop a way to predict who is most likely to land themselves a pricey ICU stay -- and offer support before those people end up needing serious care.
Embracing data use in such specific ways also brings up issues of data security and patient safety.
"There was an early understanding, even if you go back to the (federal) balanced budget act of 1997, that we were just kicking the can down the road to having a functional financial model to deliver healthcare to everyone with a reasonable price," Nester says. "We've got a lot of people living longer without more of an investment in the healthcare trust."
Popultyics, founded in 2013, was the result of years of planning and agonizing over those population numbers and cost concerns.
"We looked at our own health plan," Nester says. Out of all the employees and dependants on the LVHN's own insurance network, "roughly 1.5 percent of our 25,000 people — under 400 people — drove $30 million of our $130 million on insurance costs -- about 25 percent."
"You don't have to boil the ocean to take cost out of the system," he says. "You just have to focus on that 1.5%."
Take Jen, the diabetic patient. High blood sugar can lead to kidney failure, which can mean weekly expensive dialysis for 20 years. Investing in the data and staff to reach patients, he says, is "pennies compared to $100 bills."
For most doctors, "there's no awareness for providers to know who they should be seeing vs. who they are seeing. There's no incentive, because the incentive is to see as many patients as you can," he says.
To change that, first the LVHN invested in the popular medical management system, Epic. Then, they negotiated with the top 18 insurance companies that cover patients in the region to allow access to their patient care data, which means they have reams of patient history to feed the analytics machine in order to make predictions about outcomes. Nester admits not every hospital could do that -- with 52 percent of the market share, LVHN had a very strong negotiating position.
Third party services take that data and churn out analytics that feeds models and care management plans. All identifying information is stripped from the data.
"We can do predictive modeling in patients," says Populytics President and CEO Gregory Kile. "We can identify care gaps. Those care gaps are noted as alerts when the patient presents at the office."
Kile uses himself as a hypothetical patient.
"I pull up Gregory Kile, and boom, I see a flag or an alert. I see he hasn't been in for his last blood test. There is a care gap there we need to complete."
"There's just so much more you can do with that information," he says, envisioning a future where follow-up for, say, knee replacement surgery and outcomes could be tracked, and either validated or changed.
Ethical Issues at the Forefront
Of course, embracing data use in such specific ways also brings up issues of security and patient safety. For example, says medical ethicist Mongovern, there are many touchpoints where breaches could occur. The public has a growing awareness of how data used to personalize their experiences, such as social media analytics, can also be monetized and sold in ways that benefit a company, but not the user. That's not to say data supporting medical decisions is a bad thing, she says, just one with potential for public distrust if not handled thoughtfully.
"You're going to need to do this to stay competitive," she says. "But there's obviously big challenges, not the least of which is patient trust."
So far, a majority of the patients targeted – 62 percent -- appear to embrace the effort.
Among the ways the LVHN uses the data is monthly reports they call registries, which include patients who have just come in contact with the health network, either through the hospital or a doctor that works with them. The community outreach team members at Populytics take the names from the list, pull their records, and start calling. So far, a majority of the patients targeted – 62 percent -- appear to embrace the effort.
Says Nester: "Most of these are vulnerable people who are thrilled to have someone care about them. So they engage, and when a person engages in their care, they take their insulin shots. It's not rocket science. The rocket science is in identifying who the people are — the delivery of care is easy."
A new type of cancer therapy is shrinking deadly brain tumors with just one treatment
MRI scans after a new kind of immunotherapy for brain cancer show remarkable progress in one patient just days after the first treatment.
Few cancers are deadlier than glioblastomas—aggressive and lethal tumors that originate in the brain or spinal cord. Five years after diagnosis, less than five percent of glioblastoma patients are still alive—and more often, glioblastoma patients live just 14 months on average after receiving a diagnosis.
But an ongoing clinical trial at Mass General Cancer Center is giving new hope to glioblastoma patients and their families. The trial, called INCIPIENT, is meant to evaluate the effects of a special type of immune cell, called CAR-T cells, on patients with recurrent glioblastoma.
How CAR-T cell therapy works
CAR-T cell therapy is a type of cancer treatment called immunotherapy, where doctors modify a patient’s own immune system specifically to find and destroy cancer cells. In CAR-T cell therapy, doctors extract the patient’s T-cells, which are immune system cells that help fight off disease—particularly cancer. These T-cells are harvested from the patient and then genetically modified in a lab to produce proteins on their surface called chimeric antigen receptors (thus becoming CAR-T cells), which makes them able to bind to a specific protein on the patient’s cancer cells. Once modified, these CAR-T cells are grown in the lab for several weeks so that they can multiply into an army of millions. When enough cells have been grown, these super-charged T-cells are infused back into the patient where they can then seek out cancer cells, bind to them, and destroy them. CAR-T cell therapies have been approved by the US Food and Drug Administration (FDA) to treat certain types of lymphomas and leukemias, as well as multiple myeloma, but haven’t been approved to treat glioblastomas—yet.
CAR-T cell therapies don’t always work against solid tumors, such as glioblastomas. Because solid tumors contain different kinds of cancer cells, some cells can evade the immune system’s detection even after CAR-T cell therapy, according to a press release from Massachusetts General Hospital. For the INCIPIENT trial, researchers modified the CAR-T cells even further in hopes of making them more effective against solid tumors. These second-generation CAR-T cells (called CARv3-TEAM-E T cells) contain special antibodies that attack EFGR, a protein expressed in the majority of glioblastoma tumors. Unlike other CAR-T cell therapies, these particular CAR-T cells were designed to be directly injected into the patient’s brain.
The INCIPIENT trial results
The INCIPIENT trial involved three patients who were enrolled in the study between March and July 2023. All three patients—a 72-year-old man, a 74-year-old man, and a 57-year-old woman—were treated with chemo and radiation and enrolled in the trial with CAR-T cells after their glioblastoma tumors came back.
The results, which were published earlier this year in the New England Journal of Medicine (NEJM), were called “rapid” and “dramatic” by doctors involved in the trial. After just a single infusion of the CAR-T cells, each patient experienced a significant reduction in their tumor sizes. Just two days after receiving the infusion, the glioblastoma tumor of the 72-year-old man decreased by nearly twenty percent. Just two months later the tumor had shrunk by an astonishing 60 percent, and the change was maintained for more than six months. The most dramatic result was in the 57-year-old female patient, whose tumor shrank nearly completely after just one infusion of the CAR-T cells.
The results of the INCIPIENT trial were unexpected and astonishing—but unfortunately, they were also temporary. For all three patients, the tumors eventually began to grow back regardless of the CAR-T cell infusions. According to the press release from MGH, the medical team is now considering treating each patient with multiple infusions or prefacing each treatment with chemotherapy to prolong the response.
While there is still “more to do,” says co-author of the study neuro-oncologist Dr. Elizabeth Gerstner, the results are still promising. If nothing else, these second-generation CAR-T cell infusions may someday be able to give patients more time than traditional treatments would allow.
“These results are exciting but they are also just the beginning,” says Dr. Marcela Maus, a doctor and professor of medicine at Mass General who was involved in the clinical trial. “They tell us that we are on the right track in pursuing a therapy that has the potential to change the outlook for this intractable disease.”
A recent study in The Lancet Oncology showed that AI found 20 percent more cancers on mammogram screens than radiologists alone.
Since the early 2000s, AI systems have eliminated more than 1.7 million jobs, and that number will only increase as AI improves. Some research estimates that by 2025, AI will eliminate more than 85 million jobs.
But for all the talk about job security, AI is also proving to be a powerful tool in healthcare—specifically, cancer detection. One recently published study has shown that, remarkably, artificial intelligence was able to detect 20 percent more cancers in imaging scans than radiologists alone.
Published in The Lancet Oncology, the study analyzed the scans of 80,000 Swedish women with a moderate hereditary risk of breast cancer who had undergone a mammogram between April 2021 and July 2022. Half of these scans were read by AI and then a radiologist to double-check the findings. The second group of scans was read by two researchers without the help of AI. (Currently, the standard of care across Europe is to have two radiologists analyze a scan before diagnosing a patient with breast cancer.)
The study showed that the AI group detected cancer in 6 out of every 1,000 scans, while the radiologists detected cancer in 5 per 1,000 scans. In other words, AI found 20 percent more cancers than the highly-trained radiologists.
Scientists have been using MRI images (like the ones pictured here) to train artificial intelligence to detect cancers earlier and with more accuracy. Here, MIT's AI system, MIRAI, looks for patterns in a patient's mammograms to detect breast cancer earlier than ever before. news.mit.edu
But even though the AI was better able to pinpoint cancer on an image, it doesn’t mean radiologists will soon be out of a job. Dr. Laura Heacock, a breast radiologist at NYU, said in an interview with CNN that radiologists do much more than simply screening mammograms, and that even well-trained technology can make errors. “These tools work best when paired with highly-trained radiologists who make the final call on your mammogram. Think of it as a tool like a stethoscope for a cardiologist.”
AI is still an emerging technology, but more and more doctors are using them to detect different cancers. For example, researchers at MIT have developed a program called MIRAI, which looks at patterns in patient mammograms across a series of scans and uses an algorithm to model a patient's risk of developing breast cancer over time. The program was "trained" with more than 200,000 breast imaging scans from Massachusetts General Hospital and has been tested on over 100,000 women in different hospitals across the world. According to MIT, MIRAI "has been shown to be more accurate in predicting the risk for developing breast cancer in the short term (over a 3-year period) compared to traditional tools." It has also been able to detect breast cancer up to five years before a patient receives a diagnosis.
The challenges for cancer-detecting AI tools now is not just accuracy. AI tools are also being challenged to perform consistently well across different ages, races, and breast density profiles, particularly given the increased risks that different women face. For example, Black women are 42 percent more likely than white women to die from breast cancer, despite having nearly the same rates of breast cancer as white women. Recently, an FDA-approved AI device for screening breast cancer has come under fire for wrongly detecting cancer in Black patients significantly more often than white patients.
As AI technology improves, radiologists will be able to accurately scan a more diverse set of patients at a larger volume than ever before, potentially saving more lives than ever.