COVID Variants Are Like “a Thief Changing Clothes” – and Our Camera System Barely Exists
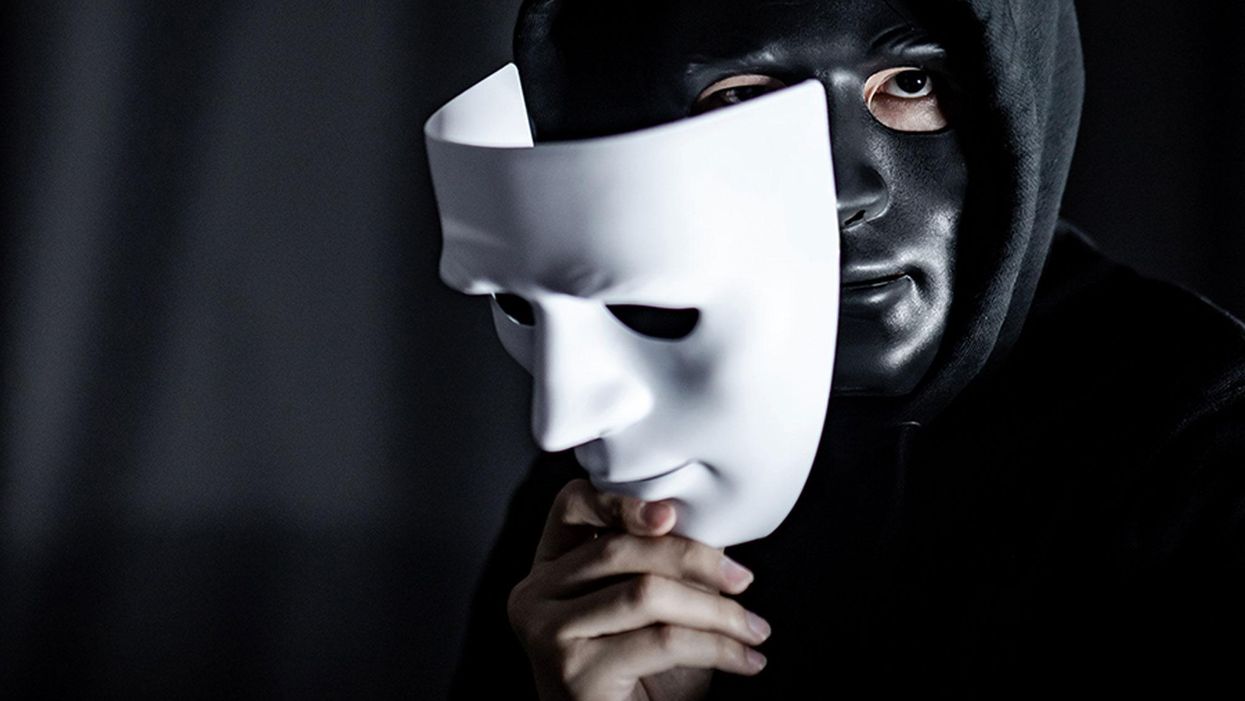
Being able to track variants of concern in real time is crucial to our ability to stay ahead of the virus.
Whether it's "natural selection" as Darwin called it, or it's "mutating" as the X-Men called it, living organisms change over time, developing thumbs or more efficient protein spikes, depending on the organism and the demands of its environment. The coronavirus that causes COVID-19, SARS-CoV-2, is not an exception, and now, after the virus has infected millions of people around the globe for more than a year, scientists are beginning to see those changes.
The notorious variants that have popped up include B.1.1.7, sometimes called the UK variant, as well as P.1 and B.1.351, which seem to have emerged in Brazil and South Africa respectively. As vaccinations are picking up pace, officials are warning that now
is not the time to become complacent or relax restrictions because the variants aren't well understood.
Some appear to be more transmissible, and deadlier, while others can evade the immune system's defenses better than earlier versions of the virus, potentially undermining the effectiveness of vaccines to some degree. Genomic surveillance, the process of sequencing the genetic code of the virus widely to observe changes and patterns, is a critical way that scientists can keep track of its evolution and work to understand how the variants might affect humans.
"It's like a thief changing clothes"
It's important to note that viruses mutate all the time. If there were funding and personnel to sequence the genome of every sample of the virus, scientists would see thousands of mutations. Not every variant deserves our attention. The vast majority of mutations are not important at all, but recognizing those that are is a crucial tool in getting and staying ahead of the virus. The work of sequencing, analyzing, observing patterns, and using public health tools as necessary is complicated and confusing to those without years of specialized training.
Jeremy Kamil, associate professor of microbiology and immunology at LSU Health Shreveport, in Louisiana, says that the variants developing are like a thief changing clothes. The thief goes in your house, steals your stuff, then leaves and puts on a different shirt and a wig, in the hopes you won't recognize them. Genomic surveillance catches the "thief" even in those different clothes.
One of the tricky things about variants is recognizing the point at which they move from interesting, to concerning at a local level, to dangerous in a larger context.
Understanding variants, both the uninteresting ones and the potentially concerning ones, gives public health officials and researchers at different levels a useful set of tools. Locally, knowing which variants are circulating in the community helps leaders know whether mask mandates and similar measures should be implemented or discontinued, or whether businesses and schools can open relatively safely.
There's more to it than observing new variants
Analysis is complex, particularly when it comes to understanding which variants are of concern. "So the question is always if a mutation becomes common, is that a random occurrence?" says Phoebe Lostroh, associate professor of molecular biology at Colorado College. "Or is the variant the result of some kind of selection because the mutation changes some property about the virus that makes it reproduce more quickly than variants of the virus that don't have that mutation? For a virus, [mutations can affect outcomes like] how much it replicates inside a person's body, how much somebody breathes it out, whether the particles that somebody might breathe in get smaller and can lead to greater transmission."
Along with all of those factors, accurate and useful genomic surveillance requires an understanding of where variants are occurring, how they are related, and an examination of why they might be prevalent.
For example, if a potentially worrisome variant appears in a community and begins to spread very quickly, it's not time to raise a public health alarm until several important questions have been answered, such as whether the variant is spreading due to specific events, or if it's happening because the mutation has allowed the virus to infect people more efficiently. Kamil offered a hypothetical scenario to explain: Imagine that a member of a community became infected and the virus mutated. That person went to church and three more people were infected, but one of them went to a karaoke bar and while singing infected 100 other people. Examining the conditions under which the virus has spread is, therefore, an essential part of untangling whether a mutation itself made the virus more transmissible or if an infected person's behaviors contributed to a local outbreak.
One of the tricky things about variants is recognizing the point at which they move from interesting, to concerning at a local level, to dangerous in a larger context. Genomic sequencing can help with that, but only when it's coordinated. When the same mutation occurs frequently, but is localized to one region, it's a concern, but when the same mutation happens in different places at the same time, it's much more likely that the "virus is learning that's a good mutation," explains Kamil.
The process is called convergent evolution, and it was a fascinating topic long before COVID. Just as your heritage can be traced through DNA, so can that of viruses, and when separate lineages develop similar traits it's almost like scientists can see evolution happening in real time. A mutation to SARS-CoV-2 that happens in more than one place at once is a mutation that makes it easier in some way for the virus to survive and that is when it may become alarming. The widespread, documented variants P.1 and B.1.351 are examples of convergence because they share some of the same virulent mutations despite having developed thousands of miles apart.
However, even variants that are emerging in different places at the same time don't present the kind of threat SARS-CoV-2 did in 2019. "This is nature," says Kamil. "It just means that this virus will not easily be driven to extinction or complete elimination by vaccines." Although a person who has already had COVID-19 can be reinfected with a variant, "it is almost always much milder disease" than the original infection, Kamil adds. Rather than causing full-fledged disease, variants have the potiental to "penetrate herd immunity, spreading relatively quietly among people who have developed natural immunity or been vaccinated, until the virus finds someone who has no immunity yet, and that person would be at risk of hospitalization-grade severe disease or death."
Surveillance and predictions
According to Lostroh, genomic surveillance can help scientists predict what's going to happen. "With the British strain, for instance, that's more transmissible, you can measure how fast it's doubling in the population and you can sort of tell whether we should take more measures against this mutation. Should we shut things down a little longer because that mutation is present in the population? That could be really useful if you did enough sampling in the population that you knew where it was," says Lostroh. If, for example, the more transmissible strain was present in 50 percent of cases, but in another county or state it was barely present, it would allow for rolling lockdowns instead of sweeping measures.
Variants are also extremely important when it comes to the development, manufacture, and distribution of vaccines. "You're also looking at medical countermeasures, such as whether your vaccine is still effective, or if your antiviral needs to be updated," says Lane Warmbrod, a senior analyst and research associate at Johns Hopkins Center for Health Security.
Properly funded and extensive genomic surveillance could eventually help control endemic diseases, too, like the seasonal flu, or other common respiratory infections. Kamil says he envisions a future in which genomic surveillance allows for prediction of sickness just as the weather is predicted today. "It's a 51 for infection today at the San Francisco Airport. There's been detection of some respiratory viruses," he says, offering an example. He says that if you're a vulnerable person, if you're immune-suppressed for some reason, you may want to wear a mask based on the sickness report.
The U.S. has the ability, but lacks standards
The benefits of widespread genomic surveillance are clear, and the United States certainly has the necessary technology, equipment, and personnel to carry it out. But, it's not happening at the speed and extent it needs to for the country to gain the benefits.
"The numbers are improving," said Kamil. "We're probably still at less than half a percent of all the samples that have been taken have been sequenced since the beginning of the pandemic."
Although there's no consensus on how many sequences is ideal for a robust surveillance program, modeling performed by the company Illumina suggests about 5 percent of positive tests should be sequenced. The reasons the U.S. has lagged in implementing a sequencing program are complex and varied, but solvable.
Perhaps the most important element that is currently missing is leadership. In order to conduct an effective genomic surveillance program, there need to be standards. The Johns Hopkins Center for Health Security recently published a paper with recommendations as to what kinds of elements need to be standardized in order to make the best use of sequencing technology and analysis.
"Along with which bioinformatic pipelines you're going to use to do the analyses, which sequencing strategy protocol are you going to use, what's your sampling strategy going to be, how is the data is going to be reported, what data gets reported," says Warmbrod. Currently, there's no guidance from the CDC on any of those things. So, while scientists can collect and report information, they may be collecting and reporting different information that isn't comparable, making it less useful for public health measures and vaccine updates.
Globally, one of the most important tools in making the information from genomic surveillance useful is GISAID, a platform designed for scientists to share -- and, importantly, to be credited for -- their data regarding genetic sequences of influenza. Originally, it was launched as a database of bird flu sequences, but has evolved to become an essential tool used by the WHO to make flu vaccine virus recommendations each year. Scientists who share their credentials have free access to the database, and anyone who uses information from the database must credit the scientist who uploaded that information.
Safety, logistics, and funding matter
Scientists at university labs and other small organizations have been uploading sequences to GISAID almost from the beginning of the pandemic, but their funding is generally limited, and there are no standards regarding information collection or reporting. Private, for-profit labs haven't had motivation to set up sequencing programs, although many of them have the logistical capabilities and funding to do so. Public health departments are understaffed, underfunded, and overwhelmed.
University labs may also be limited by safety concerns. The SARS-CoV-2 virus is dangerous, and there's a question of how samples should be transported to labs for sequencing.
Larger, for-profit organizations often have the tools and distribution capabilities to safely collect and sequence samples, but there hasn't been a profit motive. Genomic sequencing is less expensive now than ever before, but even at $100 per sample, the cost adds up -- not to mention the cost of employing a scientist with the proper credentials to analyze the sequence.
The path forward
The recently passed COVID-19 relief bill does have some funding to address genomic sequencing. Specifically, the American Rescue Plan Act includes $1.75 billion in funding for the Centers for Disease Control and Prevention's Advanced Molecular Detection (AMD) program. In an interview last month, CDC Director Rochelle Walensky said that the additional funding will be "a dial. And we're going to need to dial it up." AMD has already announced a collaboration called the Sequencing for Public Health Emergency Response, Epidemiology, and Surveillance (SPHERES) Initiative that will bring together scientists from public health, academic, clinical, and non-profit laboratories across the country with the goal of accelerating sequencing.
Such a collaboration is a step toward following the recommendations in the paper Warmbrod coauthored. Building capacity now, creating a network of labs, and standardizing procedures will mean improved health in the future. "I want to be optimistic," she says. "The good news is there are a lot of passionate, smart, capable people who are continuing to work with government and work with different stakeholders." She cautions, however, that without a national strategy we won't succeed.
"If we maximize the potential and create that framework now, we can also use it for endemic diseases," she says. "It's a very helpful system for more than COVID if we're smart in how we plan it."
Giving robots self-awareness as they move through space - and maybe even providing them with gene-like methods for storing rules of behavior - could be important steps toward creating more intelligent machines.
One day in recent past, scientists at Columbia University’s Creative Machines Lab set up a robotic arm inside a circle of five streaming video cameras and let the robot watch itself move, turn and twist. For about three hours the robot did exactly that—it looked at itself this way and that, like toddlers exploring themselves in a room full of mirrors. By the time the robot stopped, its internal neural network finished learning the relationship between the robot’s motor actions and the volume it occupied in its environment. In other words, the robot built a spatial self-awareness, just like humans do. “We trained its deep neural network to understand how it moved in space,” says Boyuan Chen, one of the scientists who worked on it.
For decades robots have been doing helpful tasks that are too hard, too dangerous, or physically impossible for humans to carry out themselves. Robots are ultimately superior to humans in complex calculations, following rules to a tee and repeating the same steps perfectly. But even the biggest successes for human-robot collaborations—those in manufacturing and automotive industries—still require separating the two for safety reasons. Hardwired for a limited set of tasks, industrial robots don't have the intelligence to know where their robo-parts are in space, how fast they’re moving and when they can endanger a human.
Over the past decade or so, humans have begun to expect more from robots. Engineers have been building smarter versions that can avoid obstacles, follow voice commands, respond to human speech and make simple decisions. Some of them proved invaluable in many natural and man-made disasters like earthquakes, forest fires, nuclear accidents and chemical spills. These disaster recovery robots helped clean up dangerous chemicals, looked for survivors in crumbled buildings, and ventured into radioactive areas to assess damage.
Now roboticists are going a step further, training their creations to do even better: understand their own image in space and interact with humans like humans do. Today, there are already robot-teachers like KeeKo, robot-pets like Moffin, robot-babysitters like iPal, and robotic companions for the elderly like Pepper.
But even these reasonably intelligent creations still have huge limitations, some scientists think. “There are niche applications for the current generations of robots,” says professor Anthony Zador at Cold Spring Harbor Laboratory—but they are not “generalists” who can do varied tasks all on their own, as they mostly lack the abilities to improvise, make decisions based on a multitude of facts or emotions, and adjust to rapidly changing circumstances. “We don’t have general purpose robots that can interact with the world. We’re ages away from that.”
Robotic spatial self-awareness – the achievement by the team at Columbia – is an important step toward creating more intelligent machines. Hod Lipson, professor of mechanical engineering who runs the Columbia lab, says that future robots will need this ability to assist humans better. Knowing how you look and where in space your parts are, decreases the need for human oversight. It also helps the robot to detect and compensate for damage and keep up with its own wear-and-tear. And it allows robots to realize when something is wrong with them or their parts. “We want our robots to learn and continue to grow their minds and bodies on their own,” Chen says. That’s what Zador wants too—and on a much grander level. “I want a robot who can drive my car, take my dog for a walk and have a conversation with me.”
Columbia scientists have trained a robot to become aware of its own "body," so it can map the right path to touch a ball without running into an obstacle, in this case a square.
Jane Nisselson and Yinuo Qin/ Columbia Engineering
Today’s technological advances are making some of these leaps of progress possible. One of them is the so-called Deep Learning—a method that trains artificial intelligence systems to learn and use information similar to how humans do it. Described as a machine learning method based on neural network architectures with multiple layers of processing units, Deep Learning has been used to successfully teach machines to recognize images, understand speech and even write text.
Trained by Google, one of these language machine learning geniuses, BERT, can finish sentences. Another one called GPT3, designed by San Francisco-based company OpenAI, can write little stories. Yet, both of them still make funny mistakes in their linguistic exercises that even a child wouldn’t. According to a paper published by Stanford’s Center for Research on Foundational Models, BERT seems to not understand the word “not.” When asked to fill in the word after “A robin is a __” it correctly answers “bird.” But try inserting the word “not” into that sentence (“A robin is not a __”) and BERT still completes it the same way. Similarly, in one of its stories, GPT3 wrote that if you mix a spoonful of grape juice into your cranberry juice and drink the concoction, you die. It seems that robots, and artificial intelligence systems in general, are still missing some rudimentary facts of life that humans and animals grasp naturally and effortlessly.
How does one give robots a genome? Zador has an idea. We can’t really equip machines with real biological nucleotide-based genes, but we can mimic the neuronal blueprint those genes create.
It's not exactly the robots’ fault. Compared to humans, and all other organisms that have been around for thousands or millions of years, robots are very new. They are missing out on eons of evolutionary data-building. Animals and humans are born with the ability to do certain things because they are pre-wired in them. Flies know how to fly, fish knows how to swim, cats know how to meow, and babies know how to cry. Yet, flies don’t really learn to fly, fish doesn’t learn to swim, cats don’t learn to meow, and babies don’t learn to cry—they are born able to execute such behaviors because they’re preprogrammed to do so. All that happens thanks to the millions of years of evolutions wired into their respective genomes, which give rise to the brain’s neural networks responsible for these behaviors. Robots are the newbies, missing out on that trove of information, Zador argues.
A neuroscience professor who studies how brain circuitry generates various behaviors, Zador has a different approach to developing the robotic mind. Until their creators figure out a way to imbue the bots with that information, robots will remain quite limited in their abilities. Each model will only be able to do certain things it was programmed to do, but it will never go above and beyond its original code. So Zador argues that we have to start giving robots a genome.
How does one do that? Zador has an idea. We can’t really equip machines with real biological nucleotide-based genes, but we can mimic the neuronal blueprint those genes create. Genomes lay out rules for brain development. Specifically, the genome encodes blueprints for wiring up our nervous system—the details of which neurons are connected, the strength of those connections and other specs that will later hold the information learned throughout life. “Our genomes serve as blueprints for building our nervous system and these blueprints give rise to a human brain, which contains about 100 billion neurons,” Zador says.
If you think what a genome is, he explains, it is essentially a very compact and compressed form of information storage. Conceptually, genomes are similar to CliffsNotes and other study guides. When students read these short summaries, they know about what happened in a book, without actually reading that book. And that’s how we should be designing the next generation of robots if we ever want them to act like humans, Zador says. “We should give them a set of behavioral CliffsNotes, which they can then unwrap into brain-like structures.” Robots that have such brain-like structures will acquire a set of basic rules to generate basic behaviors and use them to learn more complex ones.
Currently Zador is in the process of developing algorithms that function like simple rules that generate such behaviors. “My algorithms would write these CliffsNotes, outlining how to solve a particular problem,” he explains. “And then, the neural networks will use these CliffsNotes to figure out which ones are useful and use them in their behaviors.” That’s how all living beings operate. They use the pre-programmed info from their genetics to adapt to their changing environments and learn what’s necessary to survive and thrive in these settings.
For example, a robot’s neural network could draw from CliffsNotes with “genetic” instructions for how to be aware of its own body or learn to adjust its movements. And other, different sets of CliffsNotes may imbue it with the basics of physical safety or the fundamentals of speech.
At the moment, Zador is working on algorithms that are trying to mimic neuronal blueprints for very simple organisms—such as earthworms, which have only 302 neurons and about 7000 synapses compared to the millions we have. That’s how evolution worked, too—expanding the brains from simple creatures to more complex to the Homo Sapiens. But if it took millions of years to arrive at modern humans, how long would it take scientists to forge a robot with human intelligence? That’s a billion-dollar question. Yet, Zador is optimistic. “My hypotheses is that if you can build simple organisms that can interact with the world, then the higher level functions will not be nearly as challenging as they currently are.”
Lina Zeldovich has written about science, medicine and technology for Popular Science, Smithsonian, National Geographic, Scientific American, Reader’s Digest, the New York Times and other major national and international publications. A Columbia J-School alumna, she has won several awards for her stories, including the ASJA Crisis Coverage Award for Covid reporting, and has been a contributing editor at Nautilus Magazine. In 2021, Zeldovich released her first book, The Other Dark Matter, published by the University of Chicago Press, about the science and business of turning waste into wealth and health. You can find her on http://linazeldovich.com/ and @linazeldovich.
Podcast: Wellness chatbots and meditation pods with Deepak Chopra
Leaps.org talked with Deepak Chopra about new technologies he's developing for mental health with Jonathan Marcoschamer, CEO of OpenSeed, and others.
Over the last few decades, perhaps no one has impacted healthy lifestyles more than Deepak Chopra. While several of his theories and recommendations have been criticized by prominent members of the scientific community, he has helped bring meditation, yoga and other practices for well-being into the mainstream in ways that benefit the health of vast numbers of people every day. His work has led many to accept new ways of thinking about alternative medicine, the power of mind over body, and the malleability of the aging process.
His impact is such that it's been observed our culture no longer recognizes him as a human being but as a pervasive symbol of new-agey personal health and spiritual growth. Last week, I had a chance to confirm that Chopra is, in fact, a human being – and deserving of his icon status – when I talked with him for the Leaps.org podcast. He relayed ideas that were wise and ancient, yet highly relevant to our world today, with the fluidity and ease of someone discussing the weather. Showing no signs of slowing down at age 76, he described his prolific work, including the publication of two books in the past year and a range of technologies he’s developing, including a meditation app, meditation pods for the workplace, and a chatbot for mental health called Piwi.
Take a listen and get inspired to do some meditation and deep thinking on the future of health. As Chopra told me, “If you don’t have time to meditate once per day, you probably need to meditate twice per day.”
Highlights:
2:10: Chopra talks about meditation broadly and meditation pods, including the ones made by OpenSeed for meditation in the workplace.
6:10: The drawbacks of quick fixes like drugs for mental health.
10:30: The benefits of group meditation versus individual meditation.
14:35: What is a "metahuman" and how to become one.
19:40: The difference between the conditioned mind and the mind that's infinitely creative.
22:48: How Chopra's views of free will differ from the views of many neuroscientists.
28:04: Thinking Fast and Slow, and the role of intuition.
31:20: Athletic and creative geniuses.
32:43: The nature of fundamental truth.
34:00: Meditation for kids.
37:12: Never alone.Love and how AI chatbots can support mental health.
42:30: Extending lifespan, gene editing and lifestyle.
46:05: Chopra's mentor in living a long good life (and my mentor).
47:45: The power of yoga.
Links:
- OpenSeed meditation pods for people to meditate at work (Chopra is an advisor to OpenSeed).
- Chopra's book from 2021, Metahuman: Unleash Your Infinite Potential
- Chopra's book from 2022, Abundance: The Inner Path to Wealth
- NeverAlone.Love, Chopra's collaboration of businesses, policy makers, mental health professionals and others to raise awareness about mental health, advance scientific research and "create a global technology platform to democratize access to resources."
- The Piwi chatbot for mental health
- The Chopra Meditation & Well-Being App for people of all ages
- Only 1.6 percent of U.S. children meditate, according to the National Center for Complementary and Integrative Health