How COVID-19 Could Usher In a New Age of Collective Drug Discovery
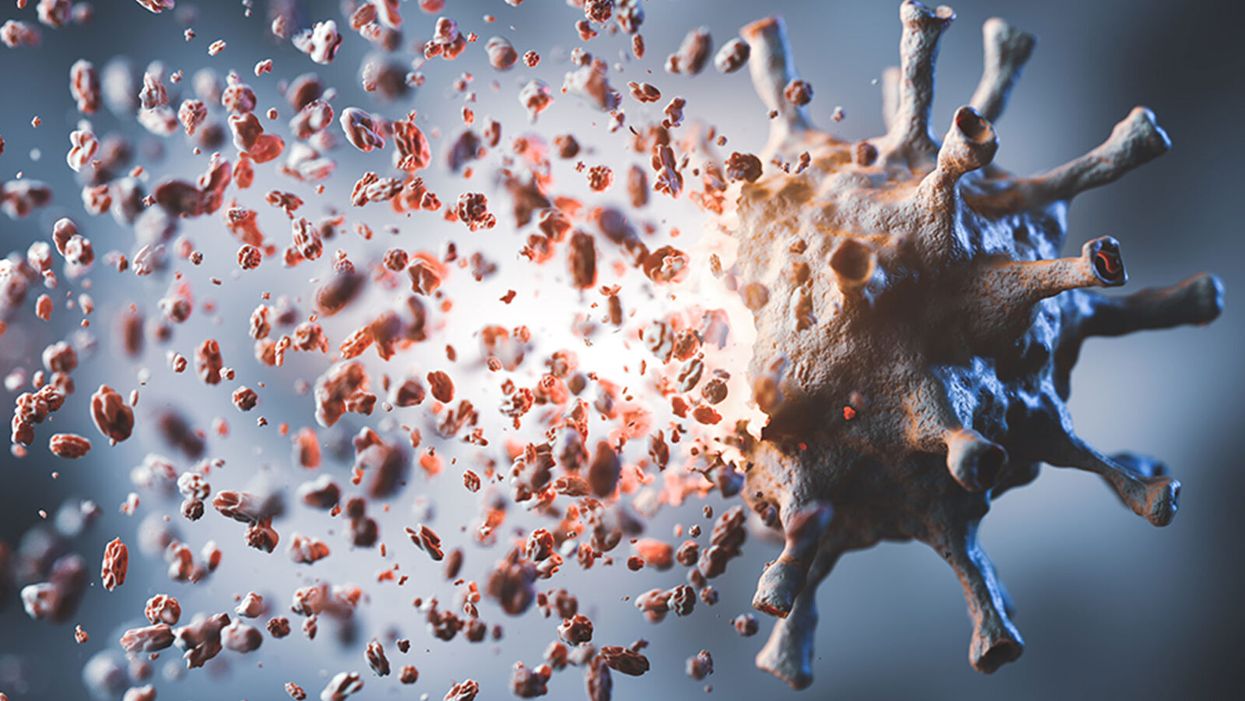
A COVID-19 moonshot program challenged chemists around the world to submit ideas for how best to design a drug to target the virus.
By mid-March, Alpha Lee was growing restless. A pioneer of AI-driven drug discovery, Lee leads a team of researchers at the University of Cambridge, but his lab had been closed amidst the government-initiated lockdowns spreading inexorably across Europe.
If the Moonshot proves successful, they hope it could serve as a future benchmark for finding new medicines for chronic diseases.
Having spoken to his collaborators across the globe – many of whom were seeing their own experiments and research projects postponed indefinitely due to the pandemic – he noticed a similar sense of frustration and helplessness in the face of COVID-19.
While there was talk of finding a novel treatment for the virus, Lee was well aware the process was likely to be long and laborious. Traditional methods of drug discovery risked suffering the same fate as the efforts to find a cure for SARS in the early 2000, which took years and were ultimately abandoned long before a drug ever reached the market.
To avoid such an outcome, Lee was convinced that global collaboration was required. Together with a collection of scientists in the UK, US and Israel, he launched the 'COVID Moonshot' – a project which encouraged chemists worldwide to share their ideas for potential drug designs. If the Moonshot proves successful, they hope it could serve as a future benchmark for finding new medicines for chronic diseases.
Solving a Complex Jigsaw
In February, ShanghaiTech University published the first detailed snapshots of the SARS-CoV-2 coronavirus's proteins using a technique called X-ray crystallography. In particular, they revealed a high-resolution profile of the virus's main protease – the part of its structure that enables it to replicate inside a host – and the main drug target. The images were tantalizing.
"We could see all the tiny pieces sitting in the structure like pieces of a jigsaw," said Lee. "All we needed was for someone to come up with the best idea of joining these pieces together with a drug. Then you'd be left with a strong molecule which sits in the protease, and stops it from working, killing the virus in the process."
Normally, ideas for how best to design such a drug would be kept as carefully guarded secrets within individual labs and companies due to their potential value. But as a result, the steady process of trial and error to reach an optimum design can take years to come to fruition.
However, given the scale of the global emergency, Lee felt that the scientific community would be open to collective brainstorming on a mass scale. "Big Pharma usually wouldn't necessarily do this, but time is of the essence here," he said. "It was a case of, 'Let's just rethink every drug discovery stage to see -- ok, how can we go as fast as we can?'"
On March 13, he launched the COVID moonshot, calling for chemists around the globe to come up with the most creative ideas they could think of, on their laptops at home. No design was too weird or wacky to be considered, and crucially nothing would be patented. The entire project would be done on a not-for-profit basis, meaning that any drug that makes it to market will have been created simply for the good of humanity.
It caught fire: Within just two weeks, more than 2,300 potential drug designs had been submitted. By the middle of July, over 10,000 had been received from scientists around the globe.
The Road Toward Clinical Trials
With so many designs to choose from, the team has been attempting to whittle them down to a shortlist of the most promising. Computational drug discovery experts at Diamond and the Weizmann Institute of Science in Rehovot, Israel, have enabled the Moonshot team to develop algorithms for predicting how quick and easy each design would be to make, and to predict how well each proposed drug might bind to the virus in real life.
The latter is an approach known as computational covalent docking and has previously been used in cancer research. "This was becoming more popular even before COVID-19, with several covalent drugs approved by the FDA in recent years," said Nir London, professor of organic chemistry at the Weizmann Institute, and one of the Moonshot team members. "However, all of these were for oncology. A covalent drug against SARS-CoV-2 will certainly highlight covalent drug-discovery as a viable option."
Through this approach, the team have selected 850 compounds to date, which they have manufactured and tested in various preclinical trials already. Fifty of these compounds - which appear to be especially promising when it comes to killing the virus in a test tube – are now being optimized further.
Lee is hoping that at least one of these potential drugs will be shown to be effective in curing animals of COVID-19 within the next six months, a step that would allow the Moonshot team to reach out to potential pharmaceutical partners to test their compounds in humans.
Future Implications
If the project does succeed, some believe it could open the door to scientific crowdsourcing as a future means of generating novel medicine ideas for other diseases. Frank von Delft, professor of protein science and structural biology at the University of Oxford's Nuffield Department of Medicine, described it as a new form of 'citizen science.'
"There's a vast resource of expertise and imagination that is simply dying to be tapped into," he said.
Others are slightly more skeptical, pointing out that the uniqueness of the current crisis has meant that many scientists were willing to contribute ideas without expecting any future compensation in return. This meant that it was easy to circumvent the traditional hurdles that prevent large-scale global collaborations from happening – namely how to decide who will profit from the final product and who will hold the intellectual property (IP) rights.
"I think it is too early to judge if this is a viable model for future drug discovery," says London. "I am not sure that without the existential threat we would have seen so many contributions, and so many people and institutions willing to waive compensation and future royalties. Many scientists found themselves at home, frustrated that they don't have a way to contribute to the fight against COVID-19, and this project gave them an opportunity. Plus many can get behind the fact that this project has no associated IP and no one will get rich off of this effort. This breaks down a lot of the typical barriers and red-tape for wider collaboration."
"If a drug would sprout from one of these crowdsourced ideas, it would serve as a very powerful argument to consider this mode of drug discovery further in the future."
However the Moonshot team believes that if they can succeed, it will at the very least send a strong statement to policy makers and the scientific community that greater efforts should be made to make such large-scale collaborations more feasible.
"All across the scientific world, we've seen unprecedented adoption of open-science, collaboration and collegiality during this crisis, perhaps recognizing that only a coordinated global effort could address this global challenge," says London. "If a drug would sprout from one of these crowdsourced ideas, it would serve as a very powerful argument to consider this mode of drug discovery further in the future."
[An earlier version of this article was published on June 8th, 2020 as part of a standalone magazine called GOOD10: The Pandemic Issue. Produced as a partnership among LeapsMag, The Aspen Institute, and GOOD, the magazine is available for free online.]
A new type of cancer therapy is shrinking deadly brain tumors with just one treatment
MRI scans after a new kind of immunotherapy for brain cancer show remarkable progress in one patient just days after the first treatment.
Few cancers are deadlier than glioblastomas—aggressive and lethal tumors that originate in the brain or spinal cord. Five years after diagnosis, less than five percent of glioblastoma patients are still alive—and more often, glioblastoma patients live just 14 months on average after receiving a diagnosis.
But an ongoing clinical trial at Mass General Cancer Center is giving new hope to glioblastoma patients and their families. The trial, called INCIPIENT, is meant to evaluate the effects of a special type of immune cell, called CAR-T cells, on patients with recurrent glioblastoma.
How CAR-T cell therapy works
CAR-T cell therapy is a type of cancer treatment called immunotherapy, where doctors modify a patient’s own immune system specifically to find and destroy cancer cells. In CAR-T cell therapy, doctors extract the patient’s T-cells, which are immune system cells that help fight off disease—particularly cancer. These T-cells are harvested from the patient and then genetically modified in a lab to produce proteins on their surface called chimeric antigen receptors (thus becoming CAR-T cells), which makes them able to bind to a specific protein on the patient’s cancer cells. Once modified, these CAR-T cells are grown in the lab for several weeks so that they can multiply into an army of millions. When enough cells have been grown, these super-charged T-cells are infused back into the patient where they can then seek out cancer cells, bind to them, and destroy them. CAR-T cell therapies have been approved by the US Food and Drug Administration (FDA) to treat certain types of lymphomas and leukemias, as well as multiple myeloma, but haven’t been approved to treat glioblastomas—yet.
CAR-T cell therapies don’t always work against solid tumors, such as glioblastomas. Because solid tumors contain different kinds of cancer cells, some cells can evade the immune system’s detection even after CAR-T cell therapy, according to a press release from Massachusetts General Hospital. For the INCIPIENT trial, researchers modified the CAR-T cells even further in hopes of making them more effective against solid tumors. These second-generation CAR-T cells (called CARv3-TEAM-E T cells) contain special antibodies that attack EFGR, a protein expressed in the majority of glioblastoma tumors. Unlike other CAR-T cell therapies, these particular CAR-T cells were designed to be directly injected into the patient’s brain.
The INCIPIENT trial results
The INCIPIENT trial involved three patients who were enrolled in the study between March and July 2023. All three patients—a 72-year-old man, a 74-year-old man, and a 57-year-old woman—were treated with chemo and radiation and enrolled in the trial with CAR-T cells after their glioblastoma tumors came back.
The results, which were published earlier this year in the New England Journal of Medicine (NEJM), were called “rapid” and “dramatic” by doctors involved in the trial. After just a single infusion of the CAR-T cells, each patient experienced a significant reduction in their tumor sizes. Just two days after receiving the infusion, the glioblastoma tumor of the 72-year-old man decreased by nearly twenty percent. Just two months later the tumor had shrunk by an astonishing 60 percent, and the change was maintained for more than six months. The most dramatic result was in the 57-year-old female patient, whose tumor shrank nearly completely after just one infusion of the CAR-T cells.
The results of the INCIPIENT trial were unexpected and astonishing—but unfortunately, they were also temporary. For all three patients, the tumors eventually began to grow back regardless of the CAR-T cell infusions. According to the press release from MGH, the medical team is now considering treating each patient with multiple infusions or prefacing each treatment with chemotherapy to prolong the response.
While there is still “more to do,” says co-author of the study neuro-oncologist Dr. Elizabeth Gerstner, the results are still promising. If nothing else, these second-generation CAR-T cell infusions may someday be able to give patients more time than traditional treatments would allow.
“These results are exciting but they are also just the beginning,” says Dr. Marcela Maus, a doctor and professor of medicine at Mass General who was involved in the clinical trial. “They tell us that we are on the right track in pursuing a therapy that has the potential to change the outlook for this intractable disease.”
A recent study in The Lancet Oncology showed that AI found 20 percent more cancers on mammogram screens than radiologists alone.
Since the early 2000s, AI systems have eliminated more than 1.7 million jobs, and that number will only increase as AI improves. Some research estimates that by 2025, AI will eliminate more than 85 million jobs.
But for all the talk about job security, AI is also proving to be a powerful tool in healthcare—specifically, cancer detection. One recently published study has shown that, remarkably, artificial intelligence was able to detect 20 percent more cancers in imaging scans than radiologists alone.
Published in The Lancet Oncology, the study analyzed the scans of 80,000 Swedish women with a moderate hereditary risk of breast cancer who had undergone a mammogram between April 2021 and July 2022. Half of these scans were read by AI and then a radiologist to double-check the findings. The second group of scans was read by two researchers without the help of AI. (Currently, the standard of care across Europe is to have two radiologists analyze a scan before diagnosing a patient with breast cancer.)
The study showed that the AI group detected cancer in 6 out of every 1,000 scans, while the radiologists detected cancer in 5 per 1,000 scans. In other words, AI found 20 percent more cancers than the highly-trained radiologists.
Scientists have been using MRI images (like the ones pictured here) to train artificial intelligence to detect cancers earlier and with more accuracy. Here, MIT's AI system, MIRAI, looks for patterns in a patient's mammograms to detect breast cancer earlier than ever before. news.mit.edu
But even though the AI was better able to pinpoint cancer on an image, it doesn’t mean radiologists will soon be out of a job. Dr. Laura Heacock, a breast radiologist at NYU, said in an interview with CNN that radiologists do much more than simply screening mammograms, and that even well-trained technology can make errors. “These tools work best when paired with highly-trained radiologists who make the final call on your mammogram. Think of it as a tool like a stethoscope for a cardiologist.”
AI is still an emerging technology, but more and more doctors are using them to detect different cancers. For example, researchers at MIT have developed a program called MIRAI, which looks at patterns in patient mammograms across a series of scans and uses an algorithm to model a patient's risk of developing breast cancer over time. The program was "trained" with more than 200,000 breast imaging scans from Massachusetts General Hospital and has been tested on over 100,000 women in different hospitals across the world. According to MIT, MIRAI "has been shown to be more accurate in predicting the risk for developing breast cancer in the short term (over a 3-year period) compared to traditional tools." It has also been able to detect breast cancer up to five years before a patient receives a diagnosis.
The challenges for cancer-detecting AI tools now is not just accuracy. AI tools are also being challenged to perform consistently well across different ages, races, and breast density profiles, particularly given the increased risks that different women face. For example, Black women are 42 percent more likely than white women to die from breast cancer, despite having nearly the same rates of breast cancer as white women. Recently, an FDA-approved AI device for screening breast cancer has come under fire for wrongly detecting cancer in Black patients significantly more often than white patients.
As AI technology improves, radiologists will be able to accurately scan a more diverse set of patients at a larger volume than ever before, potentially saving more lives than ever.