Scientists forecast new disease outbreaks
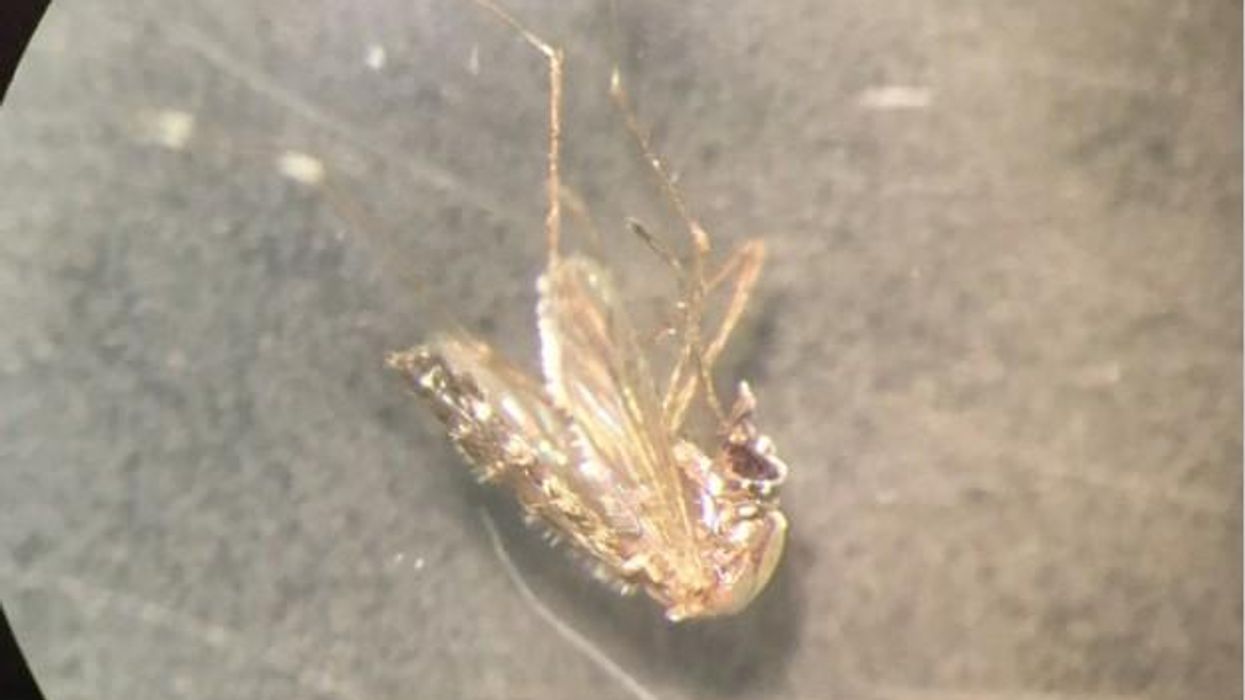
A mosquito under the microscope.
Two years, six million deaths and still counting, scientists are searching for answers to prevent another COVID-19-like tragedy from ever occurring again. And it’s a gargantuan task.
Our disturbed ecosystems are creating more favorable conditions for the spread of infectious disease. Global warming, deforestation, rising sea levels and flooding have contributed to a rise in mosquito-borne infections and longer tick seasons. Disease-carrying animals are in closer range to other species and humans as they migrate to escape the heat. Bats are thought to have carried the SARS-CoV-2 virus to Wuhan, either directly or through another host animal, but thousands of novel viruses are lurking within other wild creatures.
Understanding how climate change contributes to the spread of disease is critical in predicting and thwarting future calamities. But the problem is that predictive models aren’t yet where they need to be for forecasting with certainty beyond the next year, as we could for weather, for instance.
The association between climate and infectious disease is poorly understood, says Irina Tezaur, a computational scientist at Sandia National Laboratories. “Correlations have been observed but it’s not known if these correlations translate to causal relationships.”
To make accurate longer-term predictions, scientists need more empirical data, multiple datasets specific to locations and diseases, and the ability to calculate risks that depend on unpredictable nature and human behavior. Another obstacle is that climate scientists and epidemiologists are not collaborating effectively, so some researchers are calling for a multidisciplinary approach, a new field called Outbreak Science.
Climate scientists are far ahead of epidemiologists in gathering essential data.
Earth System Models—combining the interactions of atmosphere, ocean, land, ice and biosphere—have been in place for two decades to monitor the effects of global climate change. These models must be combined with epidemiological and human model research, areas that are easily skewed by unpredictable elements, from extreme weather events to public environmental policy shifts.
“There is never just one driver in tracking the impact of climate on infectious disease,” says Joacim Rocklöv, a professor at the Heidelberg Institute of Global Health & Heidelberg Interdisciplinary Centre for Scientific Computing in Germany. Rocklöv has studied how climate affects vector-borne diseases—those transmitted to humans by mosquitoes, ticks or fleas. “You need to disentangle the variables to find out how much difference climate makes to the outcome and how much is other factors.” Determinants from deforestation to population density to lack of healthcare access influence the spread of disease.
Even though climate change is not the primary driver of infectious disease today, it poses a major threat to public health in the future, says Rocklöv.
The promise of predictive modeling
“Models are simplifications of a system we’re trying to understand,” says Jeremy Hess, who directs the Center for Health and the Global Environment at University of Washington in Seattle. “They’re tools for learning that improve over time with new observations.”
Accurate predictions depend on high-quality, long-term observational data but models must start with assumptions. “It’s not possible to apply an evidence-based approach for the next 40 years,” says Rocklöv. “Using models to experiment and learn is the only way to figure out what climate means for infectious disease. We collect data and analyze what already happened. What we do today will not make a difference for several decades.”
To improve accuracy, scientists develop and draw on thousands of models to cover as many scenarios as possible. One model may capture the dynamics of disease transmission while another focuses on immunity data or ocean influences or seasonal components of a virus. Further, each model needs to be disease-specific and often location-specific to be useful.
“All models have biases so it’s important to use a suite of models,” Tezaur stresses.
The modeling scientist chooses the drivers of change and parameters based on the question explored. The drivers could be increased precipitation, poverty or mosquito prevalence, for instance. Later, the scientist may need to isolate the effect of one driver so that will require another model.
There have been some related successes, such as the latest models for mosquito-borne diseases like Dengue, Zika and malaria as well as those for flu and tick-borne diseases, says Hess.
Rocklöv was part of a research team that used test data from 2018 and 2019 to identify regions at risk for West Nile virus outbreaks. Using AI, scientists were able to forecast outbreaks of the virus for the entire transmission season in Europe. “In the end, we want data-driven models; that’s what AI can accomplish,” says Rocklöv. Other researchers are making an important headway in creating a framework to predict novel host–parasite interactions.
Modeling studies can run months, years or decades. “The scientist is working with layers of data. The challenge is how to transform and couple different models together on a planetary scale,” says Jeanne Fair, a scientist at Los Alamos National Laboratory, Biosecurity and Public Health, in New Mexico.
Disease forecasting will require a significant investment into the infrastructure needed to collect data about the environment, vectors, and hosts a tall spatial and temporal resolutions.
And it’s a constantly changing picture. A modeling study in an April 2022 issue of Nature predicted that thousands of animals will migrate to cooler locales as temperatures rise. This means that various species will come into closer contact with people and other mammals for the first time. This is likely to increase the risk of emerging infectious disease transmitted from animals to humans, especially in Africa and Asia.
Other things can happen too. Global warming could precipitate viral mutations or new infectious diseases that don’t respond to antimicrobial treatments. Insecticide-resistant mosquitoes could evolve. Weather-related food insecurity could increase malnutrition and weaken people’s immune systems. And the impact of an epidemic will be worse if it co-occurs during a heatwave, flood, or drought, says Hess.
The devil is in the climate variables
Solid predictions about the future of climate and disease are not possible with so many uncertainties. Difficult-to-measure drivers must be added to the empirical model mix, such as land and water use, ecosystem changes or the public’s willingness to accept a vaccine or practice social distancing. Nor is there any precedent for calculating the effect of climate changes that are accelerating at a faster speed than ever before.
The most critical climate variables thought to influence disease spread are temperature, precipitation, humidity, sunshine and wind, according to Tezaur’s research. And then there are variables within variables. Influenza scientists, for example, found that warm winters were predictors of the most severe flu seasons in the following year.
The human factor may be the most challenging determinant. To what degree will people curtail greenhouse gas emissions, if at all? The swift development of effective COVID-19 vaccines was a game-changer, but will scientists be able to repeat it during the next pandemic? Plus, no model could predict the amount of internet-fueled COVID-19 misinformation, Fair noted. To tackle this issue, infectious disease teams are looking to include more sociologists and political scientists in their modeling.
Addressing the gaps
Currently, researchers are focusing on the near future, predicting for next year, says Fair. “When it comes to long-term, that’s where we have the most work to do.” While scientists cannot foresee how political influences and misinformation spread will affect models, they are positioned to make headway in collecting and assessing new data streams that have never been merged.
Disease forecasting will require a significant investment into the infrastructure needed to collect data about the environment, vectors, and hosts at all spatial and temporal resolutions, Fair and her co-authors stated in their recent study. For example real-time data on mosquito prevalence and diversity in various settings and times is limited or non-existent. Fair also would like to see standards set in mosquito data collection in every country. “Standardizing across the US would be a huge accomplishment,” she says.
Understanding how climate change contributes to the spread of disease is critical for thwarting future calamities.
Jeanne Fair
Hess points to a dearth of data in local and regional datasets about how extreme weather events play out in different geographic locations. His research indicates that Africa and the Middle East experienced substantial climate shifts, for example, but are unrepresented in the evidentiary database, which limits conclusions. “A model for dengue may be good in Singapore but not necessarily in Port-au-Prince,” Hess explains. And, he adds, scientists need a way of evaluating models for how effective they are.
The hope, Rocklöv says, is that in the future we will have data-driven models rather than theoretical ones. In turn, sharper statistical analyses can inform resource allocation and intervention strategies to prevent outbreaks.
Most of all, experts emphasize that epidemiologists and climate scientists must stop working in silos. If scientists can successfully merge epidemiological data with climatic, biological, environmental, ecological and demographic data, they will make better predictions about complex disease patterns. Modeling “cross talk” and among disciplines and, in some cases, refusal to release data between countries is hindering discovery and advances.
It’s time for bold transdisciplinary action, says Hess. He points to initiatives that need funding in disease surveillance and control; developing and testing interventions; community education and social mobilization; decision-support analytics to predict when and where infections will emerge; advanced methodologies to improve modeling; training scientists in data management and integrated surveillance.
Establishing a new field of Outbreak Science to coordinate collaboration would accelerate progress. Investment in decision-support modeling tools for public health teams, policy makers, and other long-term planning stakeholders is imperative, too. We need to invest in programs that encourage people from climate modeling and epidemiology to work together in a cohesive fashion, says Tezaur. Joining forces is the only way to solve the formidable challenges ahead.
This article originally appeared in One Health/One Planet, a single-issue magazine that explores how climate change and other environmental shifts are increasing vulnerabilities to infectious diseases by land and by sea. The magazine probes how scientists are making progress with leaders in other fields toward solutions that embrace diverse perspectives and the interconnectedness of all lifeforms and the planet.
The association between climate and infectious disease is poorly understood, says Irina Tezaur, a computational scientist at Sandia National Laboratories. “Correlations have been observed but it’s not known if these correlations translate to causal relationships.”
To make accurate longer-term predictions, scientists need more empirical data, multiple datasets specific to locations and diseases, and the ability to calculate risks that depend on unpredictable nature and human behavior. Another obstacle is that climate scientists and epidemiologists are not collaborating effectively, so some researchers are calling for a multidisciplinary approach, a new field called Outbreak Science.
Climate scientists are far ahead of epidemiologists in gathering essential data.
Earth System Models—combining the interactions of atmosphere, ocean, land, ice and biosphere—have been in place for two decades to monitor the effects of global climate change. These models must be combined with epidemiological and human model research, areas that are easily skewed by unpredictable elements, from extreme weather events to public environmental policy shifts.
“There is never just one driver in tracking the impact of climate on infectious disease,” says Joacim Rocklöv, a professor at the Heidelberg Institute of Global Health & Heidelberg Interdisciplinary Centre for Scientific Computing in Germany. Rocklöv has studied how climate affects vector-borne diseases—those transmitted to humans by mosquitoes, ticks or fleas. “You need to disentangle the variables to find out how much difference climate makes to the outcome and how much is other factors.” Determinants from deforestation to population density to lack of healthcare access influence the spread of disease.
Even though climate change is not the primary driver of infectious disease today, it poses a major threat to public health in the future, says Rocklöv.
The promise of predictive modeling
“Models are simplifications of a system we’re trying to understand,” says Jeremy Hess, who directs the Center for Health and the Global Environment at University of Washington in Seattle. “They’re tools for learning that improve over time with new observations.”
Accurate predictions depend on high-quality, long-term observational data but models must start with assumptions. “It’s not possible to apply an evidence-based approach for the next 40 years,” says Rocklöv. “Using models to experiment and learn is the only way to figure out what climate means for infectious disease. We collect data and analyze what already happened. What we do today will not make a difference for several decades.”
To improve accuracy, scientists develop and draw on thousands of models to cover as many scenarios as possible. One model may capture the dynamics of disease transmission while another focuses on immunity data or ocean influences or seasonal components of a virus. Further, each model needs to be disease-specific and often location-specific to be useful.
“All models have biases so it’s important to use a suite of models,” Tezaur stresses.
The modeling scientist chooses the drivers of change and parameters based on the question explored. The drivers could be increased precipitation, poverty or mosquito prevalence, for instance. Later, the scientist may need to isolate the effect of one driver so that will require another model.
There have been some related successes, such as the latest models for mosquito-borne diseases like Dengue, Zika and malaria as well as those for flu and tick-borne diseases, says Hess.
Rocklöv was part of a research team that used test data from 2018 and 2019 to identify regions at risk for West Nile virus outbreaks. Using AI, scientists were able to forecast outbreaks of the virus for the entire transmission season in Europe. “In the end, we want data-driven models; that’s what AI can accomplish,” says Rocklöv. Other researchers are making an important headway in creating a framework to predict novel host–parasite interactions.
Modeling studies can run months, years or decades. “The scientist is working with layers of data. The challenge is how to transform and couple different models together on a planetary scale,” says Jeanne Fair, a scientist at Los Alamos National Laboratory, Biosecurity and Public Health, in New Mexico.
Disease forecasting will require a significant investment into the infrastructure needed to collect data about the environment, vectors, and hosts a tall spatial and temporal resolutions.
And it’s a constantly changing picture. A modeling study in an April 2022 issue of Nature predicted that thousands of animals will migrate to cooler locales as temperatures rise. This means that various species will come into closer contact with people and other mammals for the first time. This is likely to increase the risk of emerging infectious disease transmitted from animals to humans, especially in Africa and Asia.
Other things can happen too. Global warming could precipitate viral mutations or new infectious diseases that don’t respond to antimicrobial treatments. Insecticide-resistant mosquitoes could evolve. Weather-related food insecurity could increase malnutrition and weaken people’s immune systems. And the impact of an epidemic will be worse if it co-occurs during a heatwave, flood, or drought, says Hess.
The devil is in the climate variables
Solid predictions about the future of climate and disease are not possible with so many uncertainties. Difficult-to-measure drivers must be added to the empirical model mix, such as land and water use, ecosystem changes or the public’s willingness to accept a vaccine or practice social distancing. Nor is there any precedent for calculating the effect of climate changes that are accelerating at a faster speed than ever before.
The most critical climate variables thought to influence disease spread are temperature, precipitation, humidity, sunshine and wind, according to Tezaur’s research. And then there are variables within variables. Influenza scientists, for example, found that warm winters were predictors of the most severe flu seasons in the following year.
The human factor may be the most challenging determinant. To what degree will people curtail greenhouse gas emissions, if at all? The swift development of effective COVID-19 vaccines was a game-changer, but will scientists be able to repeat it during the next pandemic? Plus, no model could predict the amount of internet-fueled COVID-19 misinformation, Fair noted. To tackle this issue, infectious disease teams are looking to include more sociologists and political scientists in their modeling.
Addressing the gaps
Currently, researchers are focusing on the near future, predicting for next year, says Fair. “When it comes to long-term, that’s where we have the most work to do.” While scientists cannot foresee how political influences and misinformation spread will affect models, they are positioned to make headway in collecting and assessing new data streams that have never been merged.
Disease forecasting will require a significant investment into the infrastructure needed to collect data about the environment, vectors, and hosts at all spatial and temporal resolutions, Fair and her co-authors stated in their recent study. For example real-time data on mosquito prevalence and diversity in various settings and times is limited or non-existent. Fair also would like to see standards set in mosquito data collection in every country. “Standardizing across the US would be a huge accomplishment,” she says.
Understanding how climate change contributes to the spread of disease is critical for thwarting future calamities.
Jeanne Fair
Hess points to a dearth of data in local and regional datasets about how extreme weather events play out in different geographic locations. His research indicates that Africa and the Middle East experienced substantial climate shifts, for example, but are unrepresented in the evidentiary database, which limits conclusions. “A model for dengue may be good in Singapore but not necessarily in Port-au-Prince,” Hess explains. And, he adds, scientists need a way of evaluating models for how effective they are.
The hope, Rocklöv says, is that in the future we will have data-driven models rather than theoretical ones. In turn, sharper statistical analyses can inform resource allocation and intervention strategies to prevent outbreaks.
Most of all, experts emphasize that epidemiologists and climate scientists must stop working in silos. If scientists can successfully merge epidemiological data with climatic, biological, environmental, ecological and demographic data, they will make better predictions about complex disease patterns. Modeling “cross talk” and among disciplines and, in some cases, refusal to release data between countries is hindering discovery and advances.
It’s time for bold transdisciplinary action, says Hess. He points to initiatives that need funding in disease surveillance and control; developing and testing interventions; community education and social mobilization; decision-support analytics to predict when and where infections will emerge; advanced methodologies to improve modeling; training scientists in data management and integrated surveillance.
Establishing a new field of Outbreak Science to coordinate collaboration would accelerate progress. Investment in decision-support modeling tools for public health teams, policy makers, and other long-term planning stakeholders is imperative, too. We need to invest in programs that encourage people from climate modeling and epidemiology to work together in a cohesive fashion, says Tezaur. Joining forces is the only way to solve the formidable challenges ahead.
This article originally appeared in One Health/One Planet, a single-issue magazine that explores how climate change and other environmental shifts are increasing vulnerabilities to infectious diseases by land and by sea. The magazine probes how scientists are making progress with leaders in other fields toward solutions that embrace diverse perspectives and the interconnectedness of all lifeforms and the planet.
A new type of cancer therapy is shrinking deadly brain tumors with just one treatment
MRI scans after a new kind of immunotherapy for brain cancer show remarkable progress in one patient just days after the first treatment.
Few cancers are deadlier than glioblastomas—aggressive and lethal tumors that originate in the brain or spinal cord. Five years after diagnosis, less than five percent of glioblastoma patients are still alive—and more often, glioblastoma patients live just 14 months on average after receiving a diagnosis.
But an ongoing clinical trial at Mass General Cancer Center is giving new hope to glioblastoma patients and their families. The trial, called INCIPIENT, is meant to evaluate the effects of a special type of immune cell, called CAR-T cells, on patients with recurrent glioblastoma.
How CAR-T cell therapy works
CAR-T cell therapy is a type of cancer treatment called immunotherapy, where doctors modify a patient’s own immune system specifically to find and destroy cancer cells. In CAR-T cell therapy, doctors extract the patient’s T-cells, which are immune system cells that help fight off disease—particularly cancer. These T-cells are harvested from the patient and then genetically modified in a lab to produce proteins on their surface called chimeric antigen receptors (thus becoming CAR-T cells), which makes them able to bind to a specific protein on the patient’s cancer cells. Once modified, these CAR-T cells are grown in the lab for several weeks so that they can multiply into an army of millions. When enough cells have been grown, these super-charged T-cells are infused back into the patient where they can then seek out cancer cells, bind to them, and destroy them. CAR-T cell therapies have been approved by the US Food and Drug Administration (FDA) to treat certain types of lymphomas and leukemias, as well as multiple myeloma, but haven’t been approved to treat glioblastomas—yet.
CAR-T cell therapies don’t always work against solid tumors, such as glioblastomas. Because solid tumors contain different kinds of cancer cells, some cells can evade the immune system’s detection even after CAR-T cell therapy, according to a press release from Massachusetts General Hospital. For the INCIPIENT trial, researchers modified the CAR-T cells even further in hopes of making them more effective against solid tumors. These second-generation CAR-T cells (called CARv3-TEAM-E T cells) contain special antibodies that attack EFGR, a protein expressed in the majority of glioblastoma tumors. Unlike other CAR-T cell therapies, these particular CAR-T cells were designed to be directly injected into the patient’s brain.
The INCIPIENT trial results
The INCIPIENT trial involved three patients who were enrolled in the study between March and July 2023. All three patients—a 72-year-old man, a 74-year-old man, and a 57-year-old woman—were treated with chemo and radiation and enrolled in the trial with CAR-T cells after their glioblastoma tumors came back.
The results, which were published earlier this year in the New England Journal of Medicine (NEJM), were called “rapid” and “dramatic” by doctors involved in the trial. After just a single infusion of the CAR-T cells, each patient experienced a significant reduction in their tumor sizes. Just two days after receiving the infusion, the glioblastoma tumor of the 72-year-old man decreased by nearly twenty percent. Just two months later the tumor had shrunk by an astonishing 60 percent, and the change was maintained for more than six months. The most dramatic result was in the 57-year-old female patient, whose tumor shrank nearly completely after just one infusion of the CAR-T cells.
The results of the INCIPIENT trial were unexpected and astonishing—but unfortunately, they were also temporary. For all three patients, the tumors eventually began to grow back regardless of the CAR-T cell infusions. According to the press release from MGH, the medical team is now considering treating each patient with multiple infusions or prefacing each treatment with chemotherapy to prolong the response.
While there is still “more to do,” says co-author of the study neuro-oncologist Dr. Elizabeth Gerstner, the results are still promising. If nothing else, these second-generation CAR-T cell infusions may someday be able to give patients more time than traditional treatments would allow.
“These results are exciting but they are also just the beginning,” says Dr. Marcela Maus, a doctor and professor of medicine at Mass General who was involved in the clinical trial. “They tell us that we are on the right track in pursuing a therapy that has the potential to change the outlook for this intractable disease.”
A recent study in The Lancet Oncology showed that AI found 20 percent more cancers on mammogram screens than radiologists alone.
Since the early 2000s, AI systems have eliminated more than 1.7 million jobs, and that number will only increase as AI improves. Some research estimates that by 2025, AI will eliminate more than 85 million jobs.
But for all the talk about job security, AI is also proving to be a powerful tool in healthcare—specifically, cancer detection. One recently published study has shown that, remarkably, artificial intelligence was able to detect 20 percent more cancers in imaging scans than radiologists alone.
Published in The Lancet Oncology, the study analyzed the scans of 80,000 Swedish women with a moderate hereditary risk of breast cancer who had undergone a mammogram between April 2021 and July 2022. Half of these scans were read by AI and then a radiologist to double-check the findings. The second group of scans was read by two researchers without the help of AI. (Currently, the standard of care across Europe is to have two radiologists analyze a scan before diagnosing a patient with breast cancer.)
The study showed that the AI group detected cancer in 6 out of every 1,000 scans, while the radiologists detected cancer in 5 per 1,000 scans. In other words, AI found 20 percent more cancers than the highly-trained radiologists.
Scientists have been using MRI images (like the ones pictured here) to train artificial intelligence to detect cancers earlier and with more accuracy. Here, MIT's AI system, MIRAI, looks for patterns in a patient's mammograms to detect breast cancer earlier than ever before. news.mit.edu
But even though the AI was better able to pinpoint cancer on an image, it doesn’t mean radiologists will soon be out of a job. Dr. Laura Heacock, a breast radiologist at NYU, said in an interview with CNN that radiologists do much more than simply screening mammograms, and that even well-trained technology can make errors. “These tools work best when paired with highly-trained radiologists who make the final call on your mammogram. Think of it as a tool like a stethoscope for a cardiologist.”
AI is still an emerging technology, but more and more doctors are using them to detect different cancers. For example, researchers at MIT have developed a program called MIRAI, which looks at patterns in patient mammograms across a series of scans and uses an algorithm to model a patient's risk of developing breast cancer over time. The program was "trained" with more than 200,000 breast imaging scans from Massachusetts General Hospital and has been tested on over 100,000 women in different hospitals across the world. According to MIT, MIRAI "has been shown to be more accurate in predicting the risk for developing breast cancer in the short term (over a 3-year period) compared to traditional tools." It has also been able to detect breast cancer up to five years before a patient receives a diagnosis.
The challenges for cancer-detecting AI tools now is not just accuracy. AI tools are also being challenged to perform consistently well across different ages, races, and breast density profiles, particularly given the increased risks that different women face. For example, Black women are 42 percent more likely than white women to die from breast cancer, despite having nearly the same rates of breast cancer as white women. Recently, an FDA-approved AI device for screening breast cancer has come under fire for wrongly detecting cancer in Black patients significantly more often than white patients.
As AI technology improves, radiologists will be able to accurately scan a more diverse set of patients at a larger volume than ever before, potentially saving more lives than ever.