This Revolutionary Medical Breakthrough Is Not a Treatment or a Cure
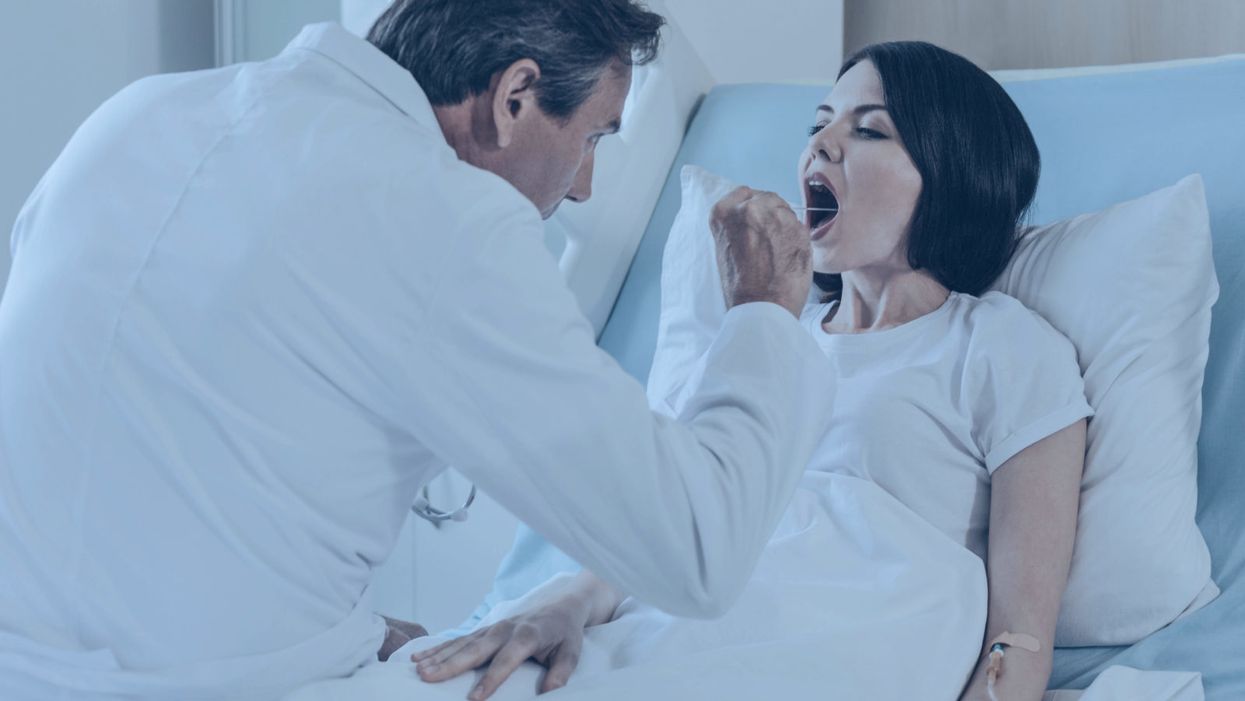
A doctor examines a patient to determine the cause of her illness.
What is a disease? This seemingly abstract and theoretical question is actually among the most practical questions in all of biomedicine. How patients are diagnosed, treated, managed and excused from various social and moral obligations hinges on the answer that is given. So do issues of how research is done and health care paid for. The question is also becoming one of the most problematic issues that those in health care will face in the next decade.
"The revolution in our understanding of the human genome, molecular biology, and genetics is creating a huge--if little acknowledged--shift in the understanding of what a disease is."
That is because the current conception of disease is undergoing a revolutionary change, fueled by progress in genetics and molecular biology. The consequences of this shift in the definition of disease promise to be as impactful as any other advance in biomedicine has ever been, which is admittedly saying a lot for what is in essence a conceptual change rather than one based on an empirical scientific advance.
For a long time, disease was defined by patient reports of feeling sick. It was not until the twentieth century that a shift occurred away from subjective reports of clusters of symptoms to defining diseases in terms of physiological states. Doctors began to realize that not all symptoms of fever represented the presence of the same disease. Flu got distinguished from malaria. Diseases such as hypertension, osteoporosis, cancer, lipidemia, silent myocardial infarction, retinopathy, blood clots and many others were recognized as not producing any or slight symptoms until suddenly the patient had a stroke or died.
The ability to assess both biology and biochemistry and to predict the consequences of subclinical pathological processes caused a distinction to be made between illness—what a person experiences—and disease—an underlying pathological process with a predictable course. Some conditions, such as Gulf War Syndrome, PTSD, many mental illnesses and fibromyalgia, remain controversial because no underlying pathological process has been found that correlates with them—a landmark criterion for diagnosing disease throughout most of the last century.
"Diseases for which no relationship had ever been posited are being lumped together due to common biochemical causal pathways...that are amenable to the same curative intervention."
The revolution in our understanding of the human genome, molecular biology, and genetics is creating a huge--if little acknowledged--shift in the understanding of what a disease is. A better understanding of the genetic and molecular roots of pathophysiology is leading to the reclassification of many familiar diseases. The test of disease is now not the pathophysiology but the presence of a gene, set of genes or molecular pathway that causes pathophysiology. Just as fever was differentiated into a multitude of diseases in the last century, cancer, cognitive impairment, addiction and many other diseases are being broken or split into many subkinds. And other diseases for which no relationship had ever been posited are being lumped together due to common biochemical causal pathways or the presence of similar dangerous biochemical products that are amenable to the same curative intervention, no matter how disparate the patients' symptoms or organic pathologies might appear.
We used to differentiate ovarian and breast cancers. Now we are thinking of them as outcomes of the same mutations in certain genes in the BRCA regions. They may eventually lump together as BRCA disease.
Other diseases such as familial amyloid polyneuropathy (FAP) which causes polyneuropathy and autonomic dysfunction are being split apart into new types or kinds. The disease is the product of mutations in the transthyretin gene. It was thought to be an autosomal dominant disease with symptomatic onset between 20-40 years of age. However, as genetic testing has improved, it has become clear that FAP's traditional clinical presentation represents a relatively small portion of those with FAP. Many patients with mutations in transthyretin — even mutations commonly seen in traditional FAP patients — do not fit the common clinical presentation. As the mutations begin to be understood, some people that were previously thought to have other polyneuropathies, such as chronic inflammatory demyelinating neuropathy, are now being rediagnosed with newly discovered variants of FAP.
"We are at the start of a major conceptual shift in how we organize the world of disease, and for that matter, health promotion."
Genome-wide association studies are beginning to find many links between diseases not thought to have any connection or association. For example some forms of diabetes, rheumatoid arthritis and thyroid disease may be the products of a small family of genetic mutations.
So why is this shift toward a genetic and molecular diagnostics likely to shake up medicine? One obvious way is that research projects may propose to recruit subjects not according to current standards of disease but on the basis of common genetic mutations or similar errors in biochemical pathways. It won't matter in a future study if subjects in a trial have what today might be termed nicotine addiction or Parkinsonism. If the molecular pathways producing the pathology are the same, then both groups might well wind up in the same trial of a drug.
In addition, what today look like common maladies—pancreatic cancer, severe depression, or acne, for example, could wind up being subdivided into so many highly differentiated versions of these conditions that each must be treated as what we now classify as a rare or ultra-rare disease. Unique biochemical markers or genetic messages may see many diseases broken into a huge number of distinct individual disease entities.
Patients may find that common genetic pathways or multiple effects from a single gene may create new alliances for fund-raising and advocacy. Groups fighting to cure mental and physical illnesses may wind up forgetting about their outward differences in the effort to alter genes or attack common protein markers.
Disease classification appears stable to us—until it isn't. And we are at the start of a major conceptual shift in how we organize the world of disease, and for that matter, health promotion. Classic reductionism, the view that all observable biological phenomena can be explained in terms of underlying chemical and physical principles, may turn out not to be true. But the molecular and genetic revolutions churning through medicine are illustrating that reductionism is going to have an enormous influence on disease classification. That is not a bad thing, but it is something that is going to take a lot to get used to.
A new type of cancer therapy is shrinking deadly brain tumors with just one treatment
MRI scans after a new kind of immunotherapy for brain cancer show remarkable progress in one patient just days after the first treatment.
Few cancers are deadlier than glioblastomas—aggressive and lethal tumors that originate in the brain or spinal cord. Five years after diagnosis, less than five percent of glioblastoma patients are still alive—and more often, glioblastoma patients live just 14 months on average after receiving a diagnosis.
But an ongoing clinical trial at Mass General Cancer Center is giving new hope to glioblastoma patients and their families. The trial, called INCIPIENT, is meant to evaluate the effects of a special type of immune cell, called CAR-T cells, on patients with recurrent glioblastoma.
How CAR-T cell therapy works
CAR-T cell therapy is a type of cancer treatment called immunotherapy, where doctors modify a patient’s own immune system specifically to find and destroy cancer cells. In CAR-T cell therapy, doctors extract the patient’s T-cells, which are immune system cells that help fight off disease—particularly cancer. These T-cells are harvested from the patient and then genetically modified in a lab to produce proteins on their surface called chimeric antigen receptors (thus becoming CAR-T cells), which makes them able to bind to a specific protein on the patient’s cancer cells. Once modified, these CAR-T cells are grown in the lab for several weeks so that they can multiply into an army of millions. When enough cells have been grown, these super-charged T-cells are infused back into the patient where they can then seek out cancer cells, bind to them, and destroy them. CAR-T cell therapies have been approved by the US Food and Drug Administration (FDA) to treat certain types of lymphomas and leukemias, as well as multiple myeloma, but haven’t been approved to treat glioblastomas—yet.
CAR-T cell therapies don’t always work against solid tumors, such as glioblastomas. Because solid tumors contain different kinds of cancer cells, some cells can evade the immune system’s detection even after CAR-T cell therapy, according to a press release from Massachusetts General Hospital. For the INCIPIENT trial, researchers modified the CAR-T cells even further in hopes of making them more effective against solid tumors. These second-generation CAR-T cells (called CARv3-TEAM-E T cells) contain special antibodies that attack EFGR, a protein expressed in the majority of glioblastoma tumors. Unlike other CAR-T cell therapies, these particular CAR-T cells were designed to be directly injected into the patient’s brain.
The INCIPIENT trial results
The INCIPIENT trial involved three patients who were enrolled in the study between March and July 2023. All three patients—a 72-year-old man, a 74-year-old man, and a 57-year-old woman—were treated with chemo and radiation and enrolled in the trial with CAR-T cells after their glioblastoma tumors came back.
The results, which were published earlier this year in the New England Journal of Medicine (NEJM), were called “rapid” and “dramatic” by doctors involved in the trial. After just a single infusion of the CAR-T cells, each patient experienced a significant reduction in their tumor sizes. Just two days after receiving the infusion, the glioblastoma tumor of the 72-year-old man decreased by nearly twenty percent. Just two months later the tumor had shrunk by an astonishing 60 percent, and the change was maintained for more than six months. The most dramatic result was in the 57-year-old female patient, whose tumor shrank nearly completely after just one infusion of the CAR-T cells.
The results of the INCIPIENT trial were unexpected and astonishing—but unfortunately, they were also temporary. For all three patients, the tumors eventually began to grow back regardless of the CAR-T cell infusions. According to the press release from MGH, the medical team is now considering treating each patient with multiple infusions or prefacing each treatment with chemotherapy to prolong the response.
While there is still “more to do,” says co-author of the study neuro-oncologist Dr. Elizabeth Gerstner, the results are still promising. If nothing else, these second-generation CAR-T cell infusions may someday be able to give patients more time than traditional treatments would allow.
“These results are exciting but they are also just the beginning,” says Dr. Marcela Maus, a doctor and professor of medicine at Mass General who was involved in the clinical trial. “They tell us that we are on the right track in pursuing a therapy that has the potential to change the outlook for this intractable disease.”
A recent study in The Lancet Oncology showed that AI found 20 percent more cancers on mammogram screens than radiologists alone.
Since the early 2000s, AI systems have eliminated more than 1.7 million jobs, and that number will only increase as AI improves. Some research estimates that by 2025, AI will eliminate more than 85 million jobs.
But for all the talk about job security, AI is also proving to be a powerful tool in healthcare—specifically, cancer detection. One recently published study has shown that, remarkably, artificial intelligence was able to detect 20 percent more cancers in imaging scans than radiologists alone.
Published in The Lancet Oncology, the study analyzed the scans of 80,000 Swedish women with a moderate hereditary risk of breast cancer who had undergone a mammogram between April 2021 and July 2022. Half of these scans were read by AI and then a radiologist to double-check the findings. The second group of scans was read by two researchers without the help of AI. (Currently, the standard of care across Europe is to have two radiologists analyze a scan before diagnosing a patient with breast cancer.)
The study showed that the AI group detected cancer in 6 out of every 1,000 scans, while the radiologists detected cancer in 5 per 1,000 scans. In other words, AI found 20 percent more cancers than the highly-trained radiologists.
Scientists have been using MRI images (like the ones pictured here) to train artificial intelligence to detect cancers earlier and with more accuracy. Here, MIT's AI system, MIRAI, looks for patterns in a patient's mammograms to detect breast cancer earlier than ever before. news.mit.edu
But even though the AI was better able to pinpoint cancer on an image, it doesn’t mean radiologists will soon be out of a job. Dr. Laura Heacock, a breast radiologist at NYU, said in an interview with CNN that radiologists do much more than simply screening mammograms, and that even well-trained technology can make errors. “These tools work best when paired with highly-trained radiologists who make the final call on your mammogram. Think of it as a tool like a stethoscope for a cardiologist.”
AI is still an emerging technology, but more and more doctors are using them to detect different cancers. For example, researchers at MIT have developed a program called MIRAI, which looks at patterns in patient mammograms across a series of scans and uses an algorithm to model a patient's risk of developing breast cancer over time. The program was "trained" with more than 200,000 breast imaging scans from Massachusetts General Hospital and has been tested on over 100,000 women in different hospitals across the world. According to MIT, MIRAI "has been shown to be more accurate in predicting the risk for developing breast cancer in the short term (over a 3-year period) compared to traditional tools." It has also been able to detect breast cancer up to five years before a patient receives a diagnosis.
The challenges for cancer-detecting AI tools now is not just accuracy. AI tools are also being challenged to perform consistently well across different ages, races, and breast density profiles, particularly given the increased risks that different women face. For example, Black women are 42 percent more likely than white women to die from breast cancer, despite having nearly the same rates of breast cancer as white women. Recently, an FDA-approved AI device for screening breast cancer has come under fire for wrongly detecting cancer in Black patients significantly more often than white patients.
As AI technology improves, radiologists will be able to accurately scan a more diverse set of patients at a larger volume than ever before, potentially saving more lives than ever.