This App Helps Diagnose Rare Genetic Disorders from a Picture
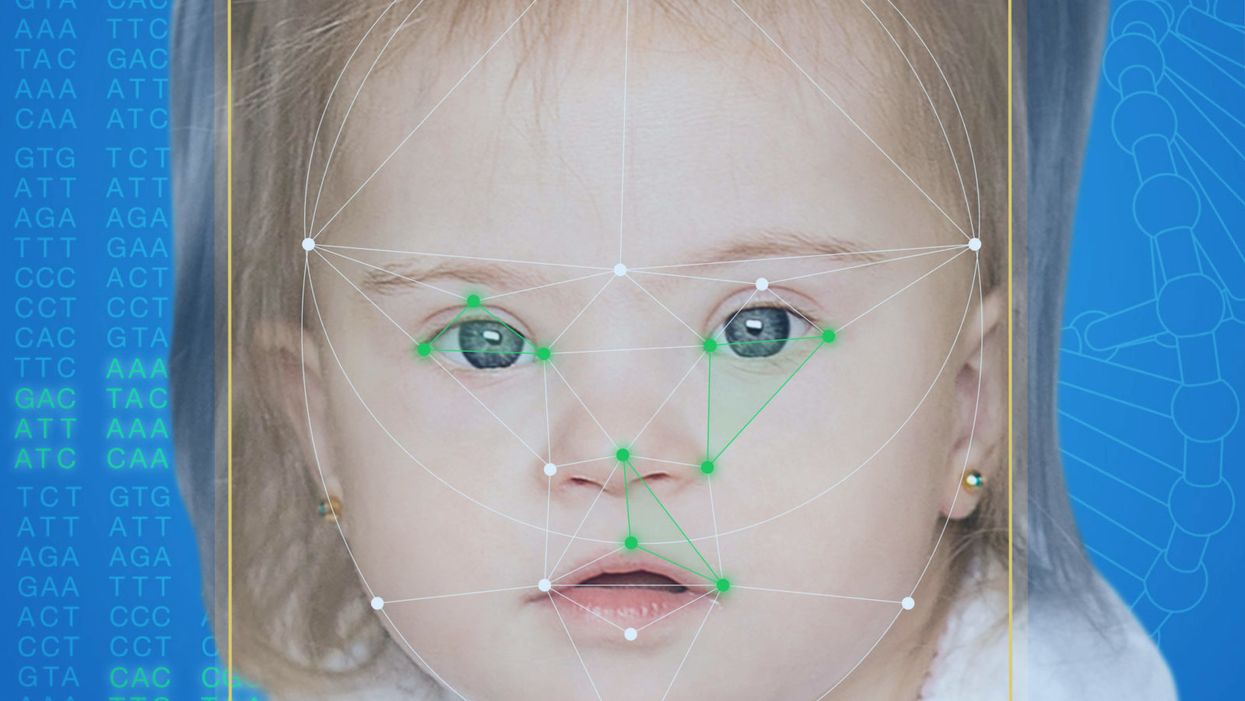
FDNA's Face2Gene technology analyzes patient biometric data using artificial intelligence, identifying correlations with disease-causing genetic variations.
Medical geneticist Omar Abdul-Rahman had a hunch. He thought that the three-year-old boy with deep-set eyes, a rounded nose, and uplifted earlobes might have Mowat-Wilson syndrome, but he'd never seen a patient with the rare disorder before.
"If it weren't for the app I'm not sure I would have had the confidence to say 'yes you should spend $1000 on this test."
Rahman had already ordered genetic tests for three different conditions without any luck, and he didn't want to cost the family any more money—or hope—if he wasn't sure of the diagnosis. So he took a picture of the boy and uploaded the photo to Face2Gene, a diagnostic aid for rare genetic disorders. Sure enough, Mowat-Wilson came up as a potential match. The family agreed to one final genetic test, which was positive for the syndrome.
"If it weren't for the app I'm not sure I would have had the confidence to say 'yes you should spend $1000 on this test,'" says Rahman, who is now the director of Genetic Medicine at the University of Nebraska Medical Center, but saw the boy when he was in the Department of Pediatrics at the University of Mississippi Medical Center in 2012.
"Families who are dealing with undiagnosed diseases never know what's going to come around the corner, what other organ system might be a problem next week," Rahman says. With a diagnosis, "You don't have to wait for the other shoe to drop because now you know the extent of the condition."
A diagnosis is the first and most important step for patients to attain medical care. Disease prognosis, treatment plans, and emotional coping all stem from this critical phase. But diagnosis can also be the trickiest part of the process, particularly for rare disorders. According to one European survey, 40 percent of rare diseases are initially misdiagnosed.
Healthcare professionals and medical technology companies hope that facial recognition software will help prevent families from facing difficult disruptions due to misdiagnoses.
"Patients with rare diseases or genetic disorders go through a long period of diagnostic odyssey, and just putting a name to a syndrome or finding a diagnosis can be very helpful and relieve a lot of tension for the family," says Dekel Gelbman, CEO of FDNA.
Consequently, a misdiagnosis can be devastating for families. Money and time may have been wasted on fruitless treatments, while opportunities for potentially helpful therapies or clinical trials were missed. Parents led down the wrong path must change their expectations of their child's long-term prognosis and care. In addition, they may be misinformed regarding future decisions about family planning.
Healthcare professionals and medical technology companies hope that facial recognition software will help prevent families from facing these difficult disruptions by improving the accuracy and ease of diagnosing genetic disorders. Traditionally, doctors diagnose these types of conditions by identifying unique patterns of facial features, a practice called dysmorphology. Trained physicians can read a child's face like a map and detect any abnormal ridges or plateaus—wide-set eyes, broad forehead, flat nose, rotated ears—that, combined with other symptoms such as intellectual disability or abnormal height and weight, signify a specific genetic disorder.
These morphological changes can be subtle, though, and often only specialized medical geneticists are able to detect and interpret these facial clues. What's more, some genetic disorders are so rare that even a specialist may not have encountered it before, much less a general practitioner. Diagnosing rare conditions has improved thanks to genomic testing that can confirm (or refute) a doctor's suspicion. Yet with thousands of variants in each person's genome, identifying the culprit mutation or deletion can be extremely difficult if you don't know what you're looking for.
Facial recognition technology is trying to take some of the guesswork out of this process. Software such as the Face2Gene app use machine learning to compare a picture of a patient against images of thousands of disorders and come back with suggestions of possible diagnoses.
"This is a classic field for artificial intelligence because no human being can really have enough knowledge and enough experience to be able to do this for thousands of different disorders."
"When we met a geneticist for the first time we were pretty blown away with the fact that they actually use their own human pattern recognition" to diagnose patients, says Gelbman. "This is a classic field for AI [artificial intelligence], for machine learning because no human being can really have enough knowledge and enough experience to be able to do this for thousands of different disorders."
When a physician uploads a photo to the app, they are given a list of different diagnostic suggestions, each with a heat map to indicate how similar the facial features are to a classic representation of the syndrome. The physician can hone the suggestions by adding in other symptoms or family history. Gelbman emphasized that the app is a "search and reference tool" and should not "be used to diagnose or treat medical conditions." It is not approved by the FDA as a diagnostic.
"As a tool, we've all been waiting for this, something that can help everyone," says Julian Martinez-Agosto, an associate professor in human genetics and pediatrics at UCLA. He sees the greatest benefit of facial recognition technology in its ability to empower non-specialists to make a diagnosis. Many areas, including rural communities or resource-poor countries, do not have access to either medical geneticists trained in these types of diagnostics or genomic screens. Apps like Face2Gene can help guide a general practitioner or flag diseases they might not be familiar with.
One concern is that most textbook images of genetic disorders come from the West, so the "classic" face of a condition is often a child of European descent.
Maximilian Muenke, a senior investigator at the National Human Genome Research Institute (NHGRI), agrees that in many countries, facial recognition programs could be the only way for a doctor to make a diagnosis.
"There are only geneticists in countries like the U.S., Canada, Europe, Japan. In most countries, geneticists don't exist at all," Muenke says. "In Nigeria, the most populous country in all of Africa with 160 million people, there's not a single clinical geneticist. So in a country like that, facial recognition programs will be sought after and will be extremely useful to help make a diagnosis to the non-geneticists."
One concern about providing this type of technology to a global population is that most textbook images of genetic disorders come from the West, so the "classic" face of a condition is often a child of European descent. However, the defining facial features of some of these disorders manifest differently across ethnicities, leaving clinicians from other geographic regions at a disadvantage.
"Every syndrome is either more easy or more difficult to detect in people from different geographic backgrounds," explains Muenke. For example, "in some countries of Southeast Asia, the eyes are slanted upward, and that happens to be one of the findings that occurs mostly with children with Down Syndrome. So then it might be more difficult for some individuals to recognize Down Syndrome in children from Southeast Asia."
There is a risk that providing this type of diagnostic information online will lead to parents trying to classify their own children.
To combat this issue, Muenke helped develop the Atlas of Human Malformation Syndromes, a database that incorporates descriptions and pictures of patients from every continent. By providing examples of rare genetic disorders in children from outside of the United States and Europe, Muenke hopes to provide clinicians with a better understanding of what to look for in each condition, regardless of where they practice.
There is a risk that providing this type of diagnostic information online will lead to parents trying to classify their own children. Face2Gene is free to download in the app store, although users must be authenticated by the company as a healthcare professional before they can access the database. The NHGRI Atlas can be accessed by anyone through their website. However, Martinez and Muenke say parents already use Google and WebMD to look up their child's symptoms; facial recognition programs and databases are just an extension of that trend. In fact, Martinez says, "Empowering families is another way to facilitate access to care. Some families live in rural areas and have no access to geneticists. If they can use software to get a diagnosis and then contact someone at a large hospital, it can help facilitate the process."
Martinez also says the app could go further by providing greater transparency about how the program makes its assessments. Giving clinicians feedback about why a diagnosis fits certain facial features would offer a valuable teaching opportunity in addition to a diagnostic aid.
Both Martinez and Muenke think the technology is an innovation that could vastly benefit patients. "In the beginning, I was quite skeptical and I could not believe that a machine could replace a human," says Muenke. "However, I am a convert that it actually can help tremendously in making a diagnosis. I think there is a place for facial recognition programs, and I am a firm believer that this will spread over the next five years."
A new type of cancer therapy is shrinking deadly brain tumors with just one treatment
MRI scans after a new kind of immunotherapy for brain cancer show remarkable progress in one patient just days after the first treatment.
Few cancers are deadlier than glioblastomas—aggressive and lethal tumors that originate in the brain or spinal cord. Five years after diagnosis, less than five percent of glioblastoma patients are still alive—and more often, glioblastoma patients live just 14 months on average after receiving a diagnosis.
But an ongoing clinical trial at Mass General Cancer Center is giving new hope to glioblastoma patients and their families. The trial, called INCIPIENT, is meant to evaluate the effects of a special type of immune cell, called CAR-T cells, on patients with recurrent glioblastoma.
How CAR-T cell therapy works
CAR-T cell therapy is a type of cancer treatment called immunotherapy, where doctors modify a patient’s own immune system specifically to find and destroy cancer cells. In CAR-T cell therapy, doctors extract the patient’s T-cells, which are immune system cells that help fight off disease—particularly cancer. These T-cells are harvested from the patient and then genetically modified in a lab to produce proteins on their surface called chimeric antigen receptors (thus becoming CAR-T cells), which makes them able to bind to a specific protein on the patient’s cancer cells. Once modified, these CAR-T cells are grown in the lab for several weeks so that they can multiply into an army of millions. When enough cells have been grown, these super-charged T-cells are infused back into the patient where they can then seek out cancer cells, bind to them, and destroy them. CAR-T cell therapies have been approved by the US Food and Drug Administration (FDA) to treat certain types of lymphomas and leukemias, as well as multiple myeloma, but haven’t been approved to treat glioblastomas—yet.
CAR-T cell therapies don’t always work against solid tumors, such as glioblastomas. Because solid tumors contain different kinds of cancer cells, some cells can evade the immune system’s detection even after CAR-T cell therapy, according to a press release from Massachusetts General Hospital. For the INCIPIENT trial, researchers modified the CAR-T cells even further in hopes of making them more effective against solid tumors. These second-generation CAR-T cells (called CARv3-TEAM-E T cells) contain special antibodies that attack EFGR, a protein expressed in the majority of glioblastoma tumors. Unlike other CAR-T cell therapies, these particular CAR-T cells were designed to be directly injected into the patient’s brain.
The INCIPIENT trial results
The INCIPIENT trial involved three patients who were enrolled in the study between March and July 2023. All three patients—a 72-year-old man, a 74-year-old man, and a 57-year-old woman—were treated with chemo and radiation and enrolled in the trial with CAR-T cells after their glioblastoma tumors came back.
The results, which were published earlier this year in the New England Journal of Medicine (NEJM), were called “rapid” and “dramatic” by doctors involved in the trial. After just a single infusion of the CAR-T cells, each patient experienced a significant reduction in their tumor sizes. Just two days after receiving the infusion, the glioblastoma tumor of the 72-year-old man decreased by nearly twenty percent. Just two months later the tumor had shrunk by an astonishing 60 percent, and the change was maintained for more than six months. The most dramatic result was in the 57-year-old female patient, whose tumor shrank nearly completely after just one infusion of the CAR-T cells.
The results of the INCIPIENT trial were unexpected and astonishing—but unfortunately, they were also temporary. For all three patients, the tumors eventually began to grow back regardless of the CAR-T cell infusions. According to the press release from MGH, the medical team is now considering treating each patient with multiple infusions or prefacing each treatment with chemotherapy to prolong the response.
While there is still “more to do,” says co-author of the study neuro-oncologist Dr. Elizabeth Gerstner, the results are still promising. If nothing else, these second-generation CAR-T cell infusions may someday be able to give patients more time than traditional treatments would allow.
“These results are exciting but they are also just the beginning,” says Dr. Marcela Maus, a doctor and professor of medicine at Mass General who was involved in the clinical trial. “They tell us that we are on the right track in pursuing a therapy that has the potential to change the outlook for this intractable disease.”
A recent study in The Lancet Oncology showed that AI found 20 percent more cancers on mammogram screens than radiologists alone.
Since the early 2000s, AI systems have eliminated more than 1.7 million jobs, and that number will only increase as AI improves. Some research estimates that by 2025, AI will eliminate more than 85 million jobs.
But for all the talk about job security, AI is also proving to be a powerful tool in healthcare—specifically, cancer detection. One recently published study has shown that, remarkably, artificial intelligence was able to detect 20 percent more cancers in imaging scans than radiologists alone.
Published in The Lancet Oncology, the study analyzed the scans of 80,000 Swedish women with a moderate hereditary risk of breast cancer who had undergone a mammogram between April 2021 and July 2022. Half of these scans were read by AI and then a radiologist to double-check the findings. The second group of scans was read by two researchers without the help of AI. (Currently, the standard of care across Europe is to have two radiologists analyze a scan before diagnosing a patient with breast cancer.)
The study showed that the AI group detected cancer in 6 out of every 1,000 scans, while the radiologists detected cancer in 5 per 1,000 scans. In other words, AI found 20 percent more cancers than the highly-trained radiologists.
Scientists have been using MRI images (like the ones pictured here) to train artificial intelligence to detect cancers earlier and with more accuracy. Here, MIT's AI system, MIRAI, looks for patterns in a patient's mammograms to detect breast cancer earlier than ever before. news.mit.edu
But even though the AI was better able to pinpoint cancer on an image, it doesn’t mean radiologists will soon be out of a job. Dr. Laura Heacock, a breast radiologist at NYU, said in an interview with CNN that radiologists do much more than simply screening mammograms, and that even well-trained technology can make errors. “These tools work best when paired with highly-trained radiologists who make the final call on your mammogram. Think of it as a tool like a stethoscope for a cardiologist.”
AI is still an emerging technology, but more and more doctors are using them to detect different cancers. For example, researchers at MIT have developed a program called MIRAI, which looks at patterns in patient mammograms across a series of scans and uses an algorithm to model a patient's risk of developing breast cancer over time. The program was "trained" with more than 200,000 breast imaging scans from Massachusetts General Hospital and has been tested on over 100,000 women in different hospitals across the world. According to MIT, MIRAI "has been shown to be more accurate in predicting the risk for developing breast cancer in the short term (over a 3-year period) compared to traditional tools." It has also been able to detect breast cancer up to five years before a patient receives a diagnosis.
The challenges for cancer-detecting AI tools now is not just accuracy. AI tools are also being challenged to perform consistently well across different ages, races, and breast density profiles, particularly given the increased risks that different women face. For example, Black women are 42 percent more likely than white women to die from breast cancer, despite having nearly the same rates of breast cancer as white women. Recently, an FDA-approved AI device for screening breast cancer has come under fire for wrongly detecting cancer in Black patients significantly more often than white patients.
As AI technology improves, radiologists will be able to accurately scan a more diverse set of patients at a larger volume than ever before, potentially saving more lives than ever.