The Algorithm Will See You Now
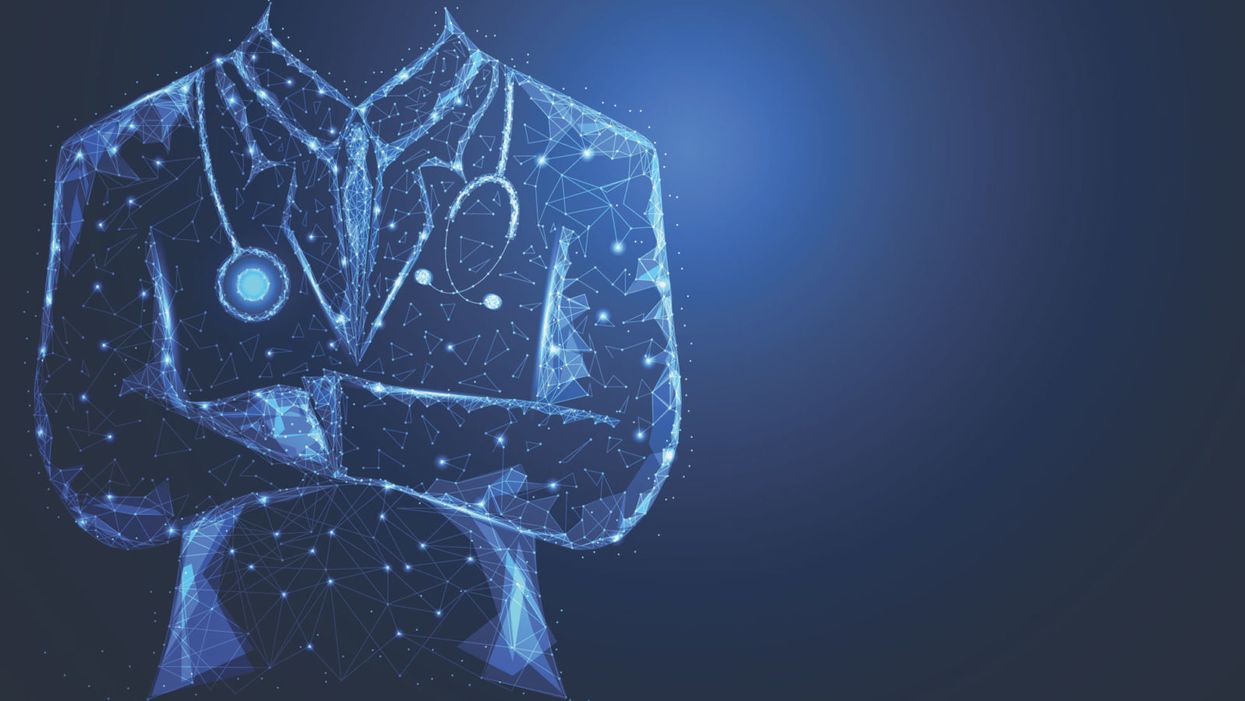
Artificial intelligence in medicine, still in an early phase, stands to transform how doctors and nurses spend their time.
There's a quiet revolution going on in medicine. It's driven by artificial intelligence, but paradoxically, new technology may put a more human face on healthcare.
AI's usefulness in healthcare ranges far and wide.
Artificial intelligence is software that can process massive amounts of information and learn over time, arriving at decisions with striking accuracy and efficiency. It offers greater accuracy in diagnosis, exponentially faster genome sequencing, the mining of medical literature and patient records at breathtaking speed, a dramatic reduction in administrative bureaucracy, personalized medicine, and even the democratization of healthcare.
The algorithms that bring these advantages won't replace doctors; rather, by offloading some of the most time-consuming tasks in healthcare, providers will be able to focus on personal interactions with patients—listening, empathizing, educating and generally putting the care back in healthcare. The relationship can focus on the alleviation of suffering, both the physical and emotional kind.
Challenges of Getting AI Up and Running
The AI revolution, still in its early phase in medicine, is already spurring some amazing advances, despite the fact that some experts say it has been overhyped. IBM's Watson Health program is a case in point. IBM capitalized on Watson's ability to process natural language by designing algorithms that devour data like medical articles and analyze images like MRIs and medical slides. The algorithms help diagnose diseases and recommend treatment strategies.
But Technology Review reported that a heavily hyped partnership with the MD Anderson Cancer Center in Houston fell apart in 2017 because of a lack of data in the proper format. The data existed, just not in a way that the voraciously data-hungry AI could use to train itself.
The hiccup certainly hasn't dampened the enthusiasm for medical AI among other tech giants, including Google and Apple, both of which have invested billions in their own healthcare projects. At this point, the main challenge is the need for algorithms to interpret a huge diversity of data mined from medical records. This can include everything from CT scans, MRIs, electrocardiograms, x-rays, and medical slides, to millions of pages of medical literature, physician's notes, and patient histories. It can even include data from implantables and wearables such as the Apple Watch and blood sugar monitors.
None of this information is in anything resembling a standard format across and even within hospitals, clinics, and diagnostic centers. Once the algorithms are trained, however, they can crunch massive amounts of data at blinding speed, with an accuracy that matches and sometimes even exceeds that of highly experienced doctors.
Genome sequencing, for example, took years to accomplish as recently as the early 2000s. The Human Genome Project, the first sequencing of the human genome, was an international effort that took 13 years to complete. In April of this year, Rady Children's Institute for Genomic Medicine in San Diego used an AI-powered genome sequencing algorithm to diagnose rare genetic diseases in infants in about 20 hours, according to ScienceDaily.
"Patient care will always begin and end with the doctor."
Dr. Stephen Kingsmore, the lead author of an article published in Science Translational Medicine, emphasized that even though the algorithm helped guide the treatment strategies of neonatal intensive care physicians, the doctor was still an indispensable link in the chain. "Some people call this artificial intelligence, we call it augmented intelligence," he says. "Patient care will always begin and end with the doctor."
One existing trend is helping to supply a great amount of valuable data to algorithms—the electronic health record. Initially blamed for exacerbating the already crushing workload of many physicians, the EHR is emerging as a boon for algorithms because it consolidates all of a patient's data in one record.
Examples of AI in Action Around the Globe
If you're a parent who has ever taken a child to the doctor with flulike symptoms, you know the anxiety of wondering if the symptoms signal something serious. Kang Zhang, M.D., Ph.D., the founding director of the Institute for Genomic Medicine at the University of California at San Diego, and colleagues developed an AI natural language processing model that used deep learning to analyze the EHRs of 1.3 million pediatric visits to a clinic in Guanzhou, China.
The AI identified common childhood diseases with about the same accuracy as human doctors, and it was even able to split the diagnoses into two categories—common conditions such as flu, and serious, life-threatening conditions like meningitis. Zhang has emphasized that the algorithm didn't replace the human doctor, but it did streamline the diagnostic process and could be used in a triage capacity when emergency room personnel need to prioritize the seriously ill over those suffering from common, less dangerous ailments.
AI's usefulness in healthcare ranges far and wide. In Uganda and several other African nations, AI is bringing modern diagnostics to remote villages that have no access to traditional technologies such as x-rays. The New York Times recently reported that there, doctors are using a pocket-sized, hand-held ultrasound machine that works in concert with a cell phone to image and diagnose everything from pneumonia (a common killer of children) to cancerous tumors.
The beauty of the highly portable, battery-powered device is that ultrasound images can be uploaded on computers so that physicians anywhere in the world can review them and weigh in with their advice. And the images are instantly incorporated into the patient's EHR.
Jonathan Rothberg, the founder of Butterfly Network, the Connecticut company that makes the device, told The New York Times that "Two thirds of the world's population gets no imaging at all. When you put something on a chip, the price goes down and you democratize it." The Butterfly ultrasound machine, which sells for $2,000, promises to be a game-changer in remote areas of Africa, South America, and Asia, as well as at the bedsides of patients in developed countries.
AI algorithms are rapidly emerging in healthcare across the U.S. and the world. China has become a major international player, set to surpass the U.S. this year in AI capital investment, the translation of AI research into marketable products, and even the number of often-cited research papers on AI. So far the U.S. is still the leader, but some experts describe the relationship between the U.S. and China as an AI cold war.
"The future of machine learning isn't sentient killer robots. It's longer human lives."
The U.S. Food and Drug Administration expanded its approval of medical algorithms from two in all of 2017 to about two per month throughout 2018. One of the first fields to be impacted is ophthalmology.
One algorithm, developed by the British AI company DeepMind (owned by Alphabet, the parent company of Google), instantly scans patients' retinas and is able to diagnose diabetic retinopathy without needing an ophthalmologist to interpret the scans. This means diabetics can get the test every year from their family physician without having to see a specialist. The Financial Times reported in March that the technology is now being used in clinics throughout Europe.
In Copenhagen, emergency service dispatchers are using a new voice-processing AI called Corti to analyze the conversations in emergency phone calls. The algorithm analyzes the verbal cues of callers, searches its huge database of medical information, and provides dispatchers with onscreen diagnostic information. Freddy Lippert, the CEO of EMS Copenhagen, notes that the algorithm has already saved lives by expediting accurate diagnoses in high-pressure situations where time is of the essence.
Researchers at the University of Nottingham in the UK have even developed a deep learning algorithm that predicts death more accurately than human clinicians. The algorithm incorporates data from a huge range of factors in a chronically ill population, including how many fruits and vegetables a patient eats on a daily basis. Dr. Stephen Weng, lead author of the study, published in PLOS ONE, said in a press release, "We found machine learning algorithms were significantly more accurate in predicting death than the standard prediction models developed by a human expert."
New digital technologies are allowing patients to participate in their healthcare as never before. A feature of the new Apple Watch is an app that detects cardiac arrhythmias and even produces an electrocardiogram if an abnormality is detected. The technology, approved by the FDA, is helping cardiologists monitor heart patients and design interventions for those who may be at higher risk of a cardiac event like a stroke.
If having an algorithm predict your death sends a shiver down your spine, consider that algorithms may keep you alive longer. In 2018, technology reporter Tristan Greene wrote for Medium that "…despite the unending deluge of panic-ridden articles declaring AI the path to apocalypse, we're now living in a world where algorithms save lives every day. The future of machine learning isn't sentient killer robots. It's longer human lives."
The Risks of AI Compiling Your Data
To be sure, the advent of AI-infused medical technology is not without its risks. One risk is that the use of AI wearables constantly monitoring our vital signs could turn us into a nation of hypochondriacs, racing to our doctors every time there's a blip in some vital sign. Such a development could stress an already overburdened system that suffers from, among other things, a shortage of doctors and nurses. Another risk has to do with the privacy protections on the massive repository of intimately personal information that AI will have on us.
In an article recently published in the Journal of the American Medical Association, Australian researcher Kit Huckvale and colleagues examined the handling of data by 36 smartphone apps that assisted people with either depression or smoking cessation, two areas that could lend themselves to stigmatization if they fell into the wrong hands.
Out of the 36 apps, 33 shared their data with third parties, despite the fact that just 25 of those apps had a privacy policy at all and out of those, only 23 stated that data would be shared with third parties. The recipients of all that data? It went almost exclusively to Facebook and Google, to be used for advertising and marketing purposes. But there's nothing to stop it from ending up in the hands of insurers, background databases, or any other entity.
Even when data isn't voluntarily shared, any digital information can be hacked. EHRs and even wearable devices share the same vulnerability as any other digital record or device. Still, the promise of AI to radically improve efficiency and accuracy in healthcare is hard to ignore.
AI Can Help Restore Humanity to Medicine
Eric Topol, director of the Scripps Research Translational Institute and author of the new book Deep Medicine, says that AI gives doctors and nurses the most precious gift of all: time.
Topol welcomes his patients' use of the Apple Watch cardiac feature and is optimistic about the ways that AI is revolutionizing medicine. He says that the watch helps doctors monitor how well medications are working and has already helped to prevent strokes. But in addition to that, AI will help bring the humanity back to a profession that has become as cold and hard as a stainless steel dissection table.
"When I graduated from medical school in the 1970s," he says, "you had a really intimate relationship with your doctor." Over the decades, he has seen that relationship steadily erode as medical organizations demanded that doctors see more and more patients within ever-shrinking time windows.
"Doctors have no time to think, to communicate. We need to restore the mission in medicine."
In addition to that, EHRs have meant that doctors and nurses are getting buried in paperwork and administrative tasks. This is no doubt one reason why a recent study by the World Health Organization showed that worldwide, about 50 percent of doctors suffer from burnout. People who are utterly exhausted make more mistakes, and medical clinicians are no different from the rest of us. Only medical mistakes have unacceptably high stakes. According to its website, Johns Hopkins University recently announced that in the U.S. alone, 250,000 people die from medical mistakes each year.
"Doctors have no time to think, to communicate," says Topol. "We need to restore the mission in medicine." AI is giving doctors more time to devote to the thing that attracted them to medicine in the first place—connecting deeply with patients.
There is a real danger at this juncture, though, that administrators aware of the time-saving aspects of AI will simply push doctors to see more patients, read more tests, and embrace an even more crushing workload.
"We can't leave it to the administrators to just make things worse," says Topol. "Now is the time for doctors to advocate for a restoration of the human touch. We need to stand up for patients and for the patient-doctor relationship."
AI could indeed be a game changer, he says, but rather than squander the huge benefits of more time, "We need a new equation going forward."
This Boy Struggled to Walk Before Gene Therapy. Now, Such Treatments Are Poised to Explode.
Conner Curran, now 10 years old, can walk more than two miles after gene therapy treatment for his Duchenne's muscular dystrophy.
Conner Curran was diagnosed with Duchenne's muscular dystrophy in 2015 when he was four years old. It's the most severe form of the genetic disease, with a nearly inevitable progression toward total paralysis. Many Duchenne's patients die in their teens; the average lifespan is 26.
But Conner, who is now 10, has experienced some astonishing improvements in recent years. He can now walk for more than two miles at a time – an impossible journey when he was younger.
In 2018, Conner became the very first patient to receive gene therapy specific to treating Duchenne's. In the initial clinical trial of nine children, nearly 80 percent reacted positively to the treatment). A larger-scale stage 3 clinical trial is currently underway, with initial results expected next year.
Gene therapy involves altering the genes in an individual's cells to stop or treat a disease. Such a procedure may be performed by adding new gene material to existing cells, or editing the defective genes to improve their functionality.
That the medical world is on the cusp of a successful treatment for a crippling and deadly disease is the culmination of more than 35 years of work by Dr. Jude Samulski, a professor of pharmacology at the University of North Carolina School of Medicine in Chapel Hill. More recently, he's become a leading gene therapy entrepreneur.
But Samulski likens this breakthrough to the frustrations of solving a Rubik's cube. "Just because one side is now all the color yellow does not mean that it is completely aligned," he says.
Although Conner's life and future have dramatically improved, he's not cured. The gene therapy tamed but did not extinguish his disorder: Conner is now suffering from the equivalent of Becker's muscular dystrophy, a milder form of the disease with symptoms that appear later in life and progress more slowly. Moreover, the loss of muscle cells Conner suffered prior to the treatment is permanent.
"It will take more time and more innovations," Samulski says of finding an even more effective gene therapy for muscular dystrophy.
Conner's family is still overjoyed with the results. "Jude's grit and determination gave Conner a chance at a new life, one that was not in his cards before gene therapy," says his mother Jessica Curran. She adds that "Conner is more confident than before and enjoys life, even though he has limitations, if compared to his brothers or peers."
Conner Curran holding a football post gene therapy treatment.
Courtesy of the Curran family
For now, the use of gene therapy as a treatment for diseases and disorders remains relatively isolated. On paper at least, progress appears glacially slow. In 2018, there were four FDA-approved gene therapies (excluding those reliant on bone marrow/stem cell transplants or implants). Today, there are 10. One therapy is solely for the cosmetic purpose of reducing facial lines and folds.
Nevertheless, experts in the space believe gene therapy is poised to expand dramatically.
"Certainly in the next three to five years you will see dozens of gene therapies and cell therapies be approved," says Dr. Pavan Cheruvu, who is CEO of Sio Gene Therapies in New York. The company is developing treatments for Parkinson's disease and Tay-Sachs, among other diseases.
Cheruvu's conclusion is supported by NEWDIGS, a think tank at the Massachusetts Institute of Technology that keeps tabs on gene therapy developments. NEWDIGS predicts there will be at least 60 gene therapies approved for use in the U.S. by the end of the decade. That number could be closer to 100 if Chinese researchers and biotech ventures decide the American market is a good fit for the therapies they develop.
"We are watching something of a conditional evolution, like a dot-com, or cellphones that were sizes of shoeboxes that have now matured to the size of wafers. Our space will follow along very similarly."
Dr. Carsten Brunn, a chemist by training and CEO of Selecta Biosciences outside of Boston, is developing ways to reduce the immune responses in patients who receive gene therapy. He observes that there are more than 300 therapies in development and thousands of clinical trials underway. "It's definitely an exciting time in the field," he says.
That's a far cry from the environment of little more than a decade ago. Research and investment in gene therapy had been brought low for years after the death of teenager Jesse Gelsinger in 1999 while he had been enrolled in a clinical trial to treat a liver disease. Gene therapy was a completely novel concept back then, and his death created existential questions about whether it was a proper pathway to pursue. Cheruvu, a cardiologist, calls the years after Gelsinger's death an "ice age" for gene therapy.
However, those dark years eventually yielded to a thaw. And while there have been some recent stumbles, they are considered part of the trial-and-error that has often accompanied medical research as opposed to an ominous "stop" sign.
The deaths of three patients last year receiving gene therapy for myotubular myopathy – a degenerative disease that causes severe muscle weakness – promptly ended the clinical trial in which they were enrolled. However, the incident caused few ripples beyond that. Researchers linked the deaths to dosage sizes that caused liver toxicity, as opposed to the gene therapy itself being an automatic death sentence; younger patients who received lower doses due to a less advanced disease state experienced improvements.
The gene sequencing and editing that helped create vaccines for COVID-19 in record time also bolstered the argument for more investment in research and development. Cheruvu notes that the field has usually been the domain of investors with significant expertise in the field; these days, more money is flowing in from generalists.
The Challenges Ahead
What will be the next step in gene therapy's evolution? Many of Samulski's earliest innovations came in the laboratory, for example. Then that led to him forming a company called AskBio in collaboration with the Muscular Dystrophy Association. AskBio sold its gene therapy to Pfizer five years ago to assure that enough could be manufactured for stage 3 clinical trials and eventually reach the market.
Cheruvu suggests that many future gene therapy innovations will be the result of what he calls "congruent innovation." That means publicly funded laboratories and privately funded companies might develop treatments separately or in collaboration. Or, university scientists may depend on private ventures to solve one of gene therapy's most vexing issues: producing enough finished material to test and treat on a large scale. "Manufacturing is a real bottleneck right now," Brunn says.
The alternative is referred to in the sector as the "valley of death": a lab has found a promising treatment, but is not far enough along in development to submit an investigational new drug application with the FDA. The promise withers away as a result. But the new abundance of venture capital for gene therapy has made this scenario less of an issue for private firms, some of which have received hundreds of millions of dollars in funding.
There are also numerous clinical challenges. Many gene therapies use what are known as adeno-associated virus vectors (AAVs) to deliver treatments. They are hollowed-out husks of viruses that can cause a variety of mostly mild maladies ranging from colds to pink eye. They are modified to deliver the genetic material used in the therapy. Most of these vectors trigger an antibody reaction that limits treatments to a single does or a handful of smaller ones. That can limit the potential progress for patients – an issue referred to as treatment "durability."
Although vectors from animals such as horses trigger far less of an antibody reaction in patients -- and there has been significant work done on using artificial vectors -- both are likely years away from being used on a large scale. "For the foreseeable future, AAV is the delivery system of choice," Brunn says.
Also, there will likely be demand for concurrent gene therapies that can lead to a complete cure – not only halting the progress of Duchenne's in kids like Conner Curran, but regenerating their lost muscle cells, perhaps through some form of stem cell therapy or another treatment that has yet to be devised.
Nevertheless, Samulski believes demand for imperfect treatments will be high – particularly with a disease such as muscular dystrophy, where many patients are mere months from spending the remainder of their lives in wheelchairs. But Samulski believes those therapies will also inevitably evolve into something far more effective.
"We are watching something of a conditional evolution, like a dot-com, or cellphones that were sizes of shoeboxes that have now matured to the size of wafers," he says. "Our space will follow along very similarly."
Jessica Curran will remain forever grateful for what her son has received: "Jude gave us new hope. He gave us something that is priceless – a chance to watch Conner grow up and live out his own dreams."
COVID Variants Are Like “a Thief Changing Clothes” – and Our Camera System Barely Exists
Being able to track variants of concern in real time is crucial to our ability to stay ahead of the virus.
Whether it's "natural selection" as Darwin called it, or it's "mutating" as the X-Men called it, living organisms change over time, developing thumbs or more efficient protein spikes, depending on the organism and the demands of its environment. The coronavirus that causes COVID-19, SARS-CoV-2, is not an exception, and now, after the virus has infected millions of people around the globe for more than a year, scientists are beginning to see those changes.
The notorious variants that have popped up include B.1.1.7, sometimes called the UK variant, as well as P.1 and B.1.351, which seem to have emerged in Brazil and South Africa respectively. As vaccinations are picking up pace, officials are warning that now
is not the time to become complacent or relax restrictions because the variants aren't well understood.
Some appear to be more transmissible, and deadlier, while others can evade the immune system's defenses better than earlier versions of the virus, potentially undermining the effectiveness of vaccines to some degree. Genomic surveillance, the process of sequencing the genetic code of the virus widely to observe changes and patterns, is a critical way that scientists can keep track of its evolution and work to understand how the variants might affect humans.
"It's like a thief changing clothes"
It's important to note that viruses mutate all the time. If there were funding and personnel to sequence the genome of every sample of the virus, scientists would see thousands of mutations. Not every variant deserves our attention. The vast majority of mutations are not important at all, but recognizing those that are is a crucial tool in getting and staying ahead of the virus. The work of sequencing, analyzing, observing patterns, and using public health tools as necessary is complicated and confusing to those without years of specialized training.
Jeremy Kamil, associate professor of microbiology and immunology at LSU Health Shreveport, in Louisiana, says that the variants developing are like a thief changing clothes. The thief goes in your house, steals your stuff, then leaves and puts on a different shirt and a wig, in the hopes you won't recognize them. Genomic surveillance catches the "thief" even in those different clothes.
One of the tricky things about variants is recognizing the point at which they move from interesting, to concerning at a local level, to dangerous in a larger context.
Understanding variants, both the uninteresting ones and the potentially concerning ones, gives public health officials and researchers at different levels a useful set of tools. Locally, knowing which variants are circulating in the community helps leaders know whether mask mandates and similar measures should be implemented or discontinued, or whether businesses and schools can open relatively safely.
There's more to it than observing new variants
Analysis is complex, particularly when it comes to understanding which variants are of concern. "So the question is always if a mutation becomes common, is that a random occurrence?" says Phoebe Lostroh, associate professor of molecular biology at Colorado College. "Or is the variant the result of some kind of selection because the mutation changes some property about the virus that makes it reproduce more quickly than variants of the virus that don't have that mutation? For a virus, [mutations can affect outcomes like] how much it replicates inside a person's body, how much somebody breathes it out, whether the particles that somebody might breathe in get smaller and can lead to greater transmission."
Along with all of those factors, accurate and useful genomic surveillance requires an understanding of where variants are occurring, how they are related, and an examination of why they might be prevalent.
For example, if a potentially worrisome variant appears in a community and begins to spread very quickly, it's not time to raise a public health alarm until several important questions have been answered, such as whether the variant is spreading due to specific events, or if it's happening because the mutation has allowed the virus to infect people more efficiently. Kamil offered a hypothetical scenario to explain: Imagine that a member of a community became infected and the virus mutated. That person went to church and three more people were infected, but one of them went to a karaoke bar and while singing infected 100 other people. Examining the conditions under which the virus has spread is, therefore, an essential part of untangling whether a mutation itself made the virus more transmissible or if an infected person's behaviors contributed to a local outbreak.
One of the tricky things about variants is recognizing the point at which they move from interesting, to concerning at a local level, to dangerous in a larger context. Genomic sequencing can help with that, but only when it's coordinated. When the same mutation occurs frequently, but is localized to one region, it's a concern, but when the same mutation happens in different places at the same time, it's much more likely that the "virus is learning that's a good mutation," explains Kamil.
The process is called convergent evolution, and it was a fascinating topic long before COVID. Just as your heritage can be traced through DNA, so can that of viruses, and when separate lineages develop similar traits it's almost like scientists can see evolution happening in real time. A mutation to SARS-CoV-2 that happens in more than one place at once is a mutation that makes it easier in some way for the virus to survive and that is when it may become alarming. The widespread, documented variants P.1 and B.1.351 are examples of convergence because they share some of the same virulent mutations despite having developed thousands of miles apart.
However, even variants that are emerging in different places at the same time don't present the kind of threat SARS-CoV-2 did in 2019. "This is nature," says Kamil. "It just means that this virus will not easily be driven to extinction or complete elimination by vaccines." Although a person who has already had COVID-19 can be reinfected with a variant, "it is almost always much milder disease" than the original infection, Kamil adds. Rather than causing full-fledged disease, variants have the potiental to "penetrate herd immunity, spreading relatively quietly among people who have developed natural immunity or been vaccinated, until the virus finds someone who has no immunity yet, and that person would be at risk of hospitalization-grade severe disease or death."
Surveillance and predictions
According to Lostroh, genomic surveillance can help scientists predict what's going to happen. "With the British strain, for instance, that's more transmissible, you can measure how fast it's doubling in the population and you can sort of tell whether we should take more measures against this mutation. Should we shut things down a little longer because that mutation is present in the population? That could be really useful if you did enough sampling in the population that you knew where it was," says Lostroh. If, for example, the more transmissible strain was present in 50 percent of cases, but in another county or state it was barely present, it would allow for rolling lockdowns instead of sweeping measures.
Variants are also extremely important when it comes to the development, manufacture, and distribution of vaccines. "You're also looking at medical countermeasures, such as whether your vaccine is still effective, or if your antiviral needs to be updated," says Lane Warmbrod, a senior analyst and research associate at Johns Hopkins Center for Health Security.
Properly funded and extensive genomic surveillance could eventually help control endemic diseases, too, like the seasonal flu, or other common respiratory infections. Kamil says he envisions a future in which genomic surveillance allows for prediction of sickness just as the weather is predicted today. "It's a 51 for infection today at the San Francisco Airport. There's been detection of some respiratory viruses," he says, offering an example. He says that if you're a vulnerable person, if you're immune-suppressed for some reason, you may want to wear a mask based on the sickness report.
The U.S. has the ability, but lacks standards
The benefits of widespread genomic surveillance are clear, and the United States certainly has the necessary technology, equipment, and personnel to carry it out. But, it's not happening at the speed and extent it needs to for the country to gain the benefits.
"The numbers are improving," said Kamil. "We're probably still at less than half a percent of all the samples that have been taken have been sequenced since the beginning of the pandemic."
Although there's no consensus on how many sequences is ideal for a robust surveillance program, modeling performed by the company Illumina suggests about 5 percent of positive tests should be sequenced. The reasons the U.S. has lagged in implementing a sequencing program are complex and varied, but solvable.
Perhaps the most important element that is currently missing is leadership. In order to conduct an effective genomic surveillance program, there need to be standards. The Johns Hopkins Center for Health Security recently published a paper with recommendations as to what kinds of elements need to be standardized in order to make the best use of sequencing technology and analysis.
"Along with which bioinformatic pipelines you're going to use to do the analyses, which sequencing strategy protocol are you going to use, what's your sampling strategy going to be, how is the data is going to be reported, what data gets reported," says Warmbrod. Currently, there's no guidance from the CDC on any of those things. So, while scientists can collect and report information, they may be collecting and reporting different information that isn't comparable, making it less useful for public health measures and vaccine updates.
Globally, one of the most important tools in making the information from genomic surveillance useful is GISAID, a platform designed for scientists to share -- and, importantly, to be credited for -- their data regarding genetic sequences of influenza. Originally, it was launched as a database of bird flu sequences, but has evolved to become an essential tool used by the WHO to make flu vaccine virus recommendations each year. Scientists who share their credentials have free access to the database, and anyone who uses information from the database must credit the scientist who uploaded that information.
Safety, logistics, and funding matter
Scientists at university labs and other small organizations have been uploading sequences to GISAID almost from the beginning of the pandemic, but their funding is generally limited, and there are no standards regarding information collection or reporting. Private, for-profit labs haven't had motivation to set up sequencing programs, although many of them have the logistical capabilities and funding to do so. Public health departments are understaffed, underfunded, and overwhelmed.
University labs may also be limited by safety concerns. The SARS-CoV-2 virus is dangerous, and there's a question of how samples should be transported to labs for sequencing.
Larger, for-profit organizations often have the tools and distribution capabilities to safely collect and sequence samples, but there hasn't been a profit motive. Genomic sequencing is less expensive now than ever before, but even at $100 per sample, the cost adds up -- not to mention the cost of employing a scientist with the proper credentials to analyze the sequence.
The path forward
The recently passed COVID-19 relief bill does have some funding to address genomic sequencing. Specifically, the American Rescue Plan Act includes $1.75 billion in funding for the Centers for Disease Control and Prevention's Advanced Molecular Detection (AMD) program. In an interview last month, CDC Director Rochelle Walensky said that the additional funding will be "a dial. And we're going to need to dial it up." AMD has already announced a collaboration called the Sequencing for Public Health Emergency Response, Epidemiology, and Surveillance (SPHERES) Initiative that will bring together scientists from public health, academic, clinical, and non-profit laboratories across the country with the goal of accelerating sequencing.
Such a collaboration is a step toward following the recommendations in the paper Warmbrod coauthored. Building capacity now, creating a network of labs, and standardizing procedures will mean improved health in the future. "I want to be optimistic," she says. "The good news is there are a lot of passionate, smart, capable people who are continuing to work with government and work with different stakeholders." She cautions, however, that without a national strategy we won't succeed.
"If we maximize the potential and create that framework now, we can also use it for endemic diseases," she says. "It's a very helpful system for more than COVID if we're smart in how we plan it."