The Algorithm Will See You Now
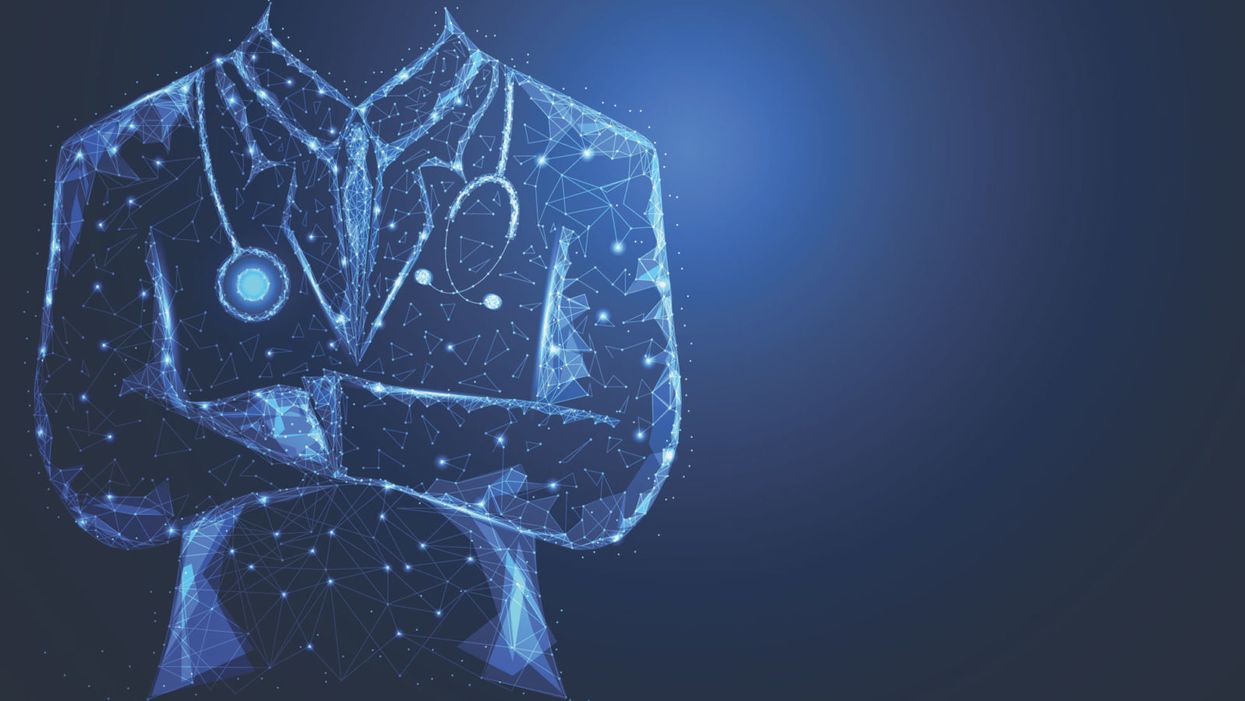
Artificial intelligence in medicine, still in an early phase, stands to transform how doctors and nurses spend their time.
There's a quiet revolution going on in medicine. It's driven by artificial intelligence, but paradoxically, new technology may put a more human face on healthcare.
AI's usefulness in healthcare ranges far and wide.
Artificial intelligence is software that can process massive amounts of information and learn over time, arriving at decisions with striking accuracy and efficiency. It offers greater accuracy in diagnosis, exponentially faster genome sequencing, the mining of medical literature and patient records at breathtaking speed, a dramatic reduction in administrative bureaucracy, personalized medicine, and even the democratization of healthcare.
The algorithms that bring these advantages won't replace doctors; rather, by offloading some of the most time-consuming tasks in healthcare, providers will be able to focus on personal interactions with patients—listening, empathizing, educating and generally putting the care back in healthcare. The relationship can focus on the alleviation of suffering, both the physical and emotional kind.
Challenges of Getting AI Up and Running
The AI revolution, still in its early phase in medicine, is already spurring some amazing advances, despite the fact that some experts say it has been overhyped. IBM's Watson Health program is a case in point. IBM capitalized on Watson's ability to process natural language by designing algorithms that devour data like medical articles and analyze images like MRIs and medical slides. The algorithms help diagnose diseases and recommend treatment strategies.
But Technology Review reported that a heavily hyped partnership with the MD Anderson Cancer Center in Houston fell apart in 2017 because of a lack of data in the proper format. The data existed, just not in a way that the voraciously data-hungry AI could use to train itself.
The hiccup certainly hasn't dampened the enthusiasm for medical AI among other tech giants, including Google and Apple, both of which have invested billions in their own healthcare projects. At this point, the main challenge is the need for algorithms to interpret a huge diversity of data mined from medical records. This can include everything from CT scans, MRIs, electrocardiograms, x-rays, and medical slides, to millions of pages of medical literature, physician's notes, and patient histories. It can even include data from implantables and wearables such as the Apple Watch and blood sugar monitors.
None of this information is in anything resembling a standard format across and even within hospitals, clinics, and diagnostic centers. Once the algorithms are trained, however, they can crunch massive amounts of data at blinding speed, with an accuracy that matches and sometimes even exceeds that of highly experienced doctors.
Genome sequencing, for example, took years to accomplish as recently as the early 2000s. The Human Genome Project, the first sequencing of the human genome, was an international effort that took 13 years to complete. In April of this year, Rady Children's Institute for Genomic Medicine in San Diego used an AI-powered genome sequencing algorithm to diagnose rare genetic diseases in infants in about 20 hours, according to ScienceDaily.
"Patient care will always begin and end with the doctor."
Dr. Stephen Kingsmore, the lead author of an article published in Science Translational Medicine, emphasized that even though the algorithm helped guide the treatment strategies of neonatal intensive care physicians, the doctor was still an indispensable link in the chain. "Some people call this artificial intelligence, we call it augmented intelligence," he says. "Patient care will always begin and end with the doctor."
One existing trend is helping to supply a great amount of valuable data to algorithms—the electronic health record. Initially blamed for exacerbating the already crushing workload of many physicians, the EHR is emerging as a boon for algorithms because it consolidates all of a patient's data in one record.
Examples of AI in Action Around the Globe
If you're a parent who has ever taken a child to the doctor with flulike symptoms, you know the anxiety of wondering if the symptoms signal something serious. Kang Zhang, M.D., Ph.D., the founding director of the Institute for Genomic Medicine at the University of California at San Diego, and colleagues developed an AI natural language processing model that used deep learning to analyze the EHRs of 1.3 million pediatric visits to a clinic in Guanzhou, China.
The AI identified common childhood diseases with about the same accuracy as human doctors, and it was even able to split the diagnoses into two categories—common conditions such as flu, and serious, life-threatening conditions like meningitis. Zhang has emphasized that the algorithm didn't replace the human doctor, but it did streamline the diagnostic process and could be used in a triage capacity when emergency room personnel need to prioritize the seriously ill over those suffering from common, less dangerous ailments.
AI's usefulness in healthcare ranges far and wide. In Uganda and several other African nations, AI is bringing modern diagnostics to remote villages that have no access to traditional technologies such as x-rays. The New York Times recently reported that there, doctors are using a pocket-sized, hand-held ultrasound machine that works in concert with a cell phone to image and diagnose everything from pneumonia (a common killer of children) to cancerous tumors.
The beauty of the highly portable, battery-powered device is that ultrasound images can be uploaded on computers so that physicians anywhere in the world can review them and weigh in with their advice. And the images are instantly incorporated into the patient's EHR.
Jonathan Rothberg, the founder of Butterfly Network, the Connecticut company that makes the device, told The New York Times that "Two thirds of the world's population gets no imaging at all. When you put something on a chip, the price goes down and you democratize it." The Butterfly ultrasound machine, which sells for $2,000, promises to be a game-changer in remote areas of Africa, South America, and Asia, as well as at the bedsides of patients in developed countries.
AI algorithms are rapidly emerging in healthcare across the U.S. and the world. China has become a major international player, set to surpass the U.S. this year in AI capital investment, the translation of AI research into marketable products, and even the number of often-cited research papers on AI. So far the U.S. is still the leader, but some experts describe the relationship between the U.S. and China as an AI cold war.
"The future of machine learning isn't sentient killer robots. It's longer human lives."
The U.S. Food and Drug Administration expanded its approval of medical algorithms from two in all of 2017 to about two per month throughout 2018. One of the first fields to be impacted is ophthalmology.
One algorithm, developed by the British AI company DeepMind (owned by Alphabet, the parent company of Google), instantly scans patients' retinas and is able to diagnose diabetic retinopathy without needing an ophthalmologist to interpret the scans. This means diabetics can get the test every year from their family physician without having to see a specialist. The Financial Times reported in March that the technology is now being used in clinics throughout Europe.
In Copenhagen, emergency service dispatchers are using a new voice-processing AI called Corti to analyze the conversations in emergency phone calls. The algorithm analyzes the verbal cues of callers, searches its huge database of medical information, and provides dispatchers with onscreen diagnostic information. Freddy Lippert, the CEO of EMS Copenhagen, notes that the algorithm has already saved lives by expediting accurate diagnoses in high-pressure situations where time is of the essence.
Researchers at the University of Nottingham in the UK have even developed a deep learning algorithm that predicts death more accurately than human clinicians. The algorithm incorporates data from a huge range of factors in a chronically ill population, including how many fruits and vegetables a patient eats on a daily basis. Dr. Stephen Weng, lead author of the study, published in PLOS ONE, said in a press release, "We found machine learning algorithms were significantly more accurate in predicting death than the standard prediction models developed by a human expert."
New digital technologies are allowing patients to participate in their healthcare as never before. A feature of the new Apple Watch is an app that detects cardiac arrhythmias and even produces an electrocardiogram if an abnormality is detected. The technology, approved by the FDA, is helping cardiologists monitor heart patients and design interventions for those who may be at higher risk of a cardiac event like a stroke.
If having an algorithm predict your death sends a shiver down your spine, consider that algorithms may keep you alive longer. In 2018, technology reporter Tristan Greene wrote for Medium that "…despite the unending deluge of panic-ridden articles declaring AI the path to apocalypse, we're now living in a world where algorithms save lives every day. The future of machine learning isn't sentient killer robots. It's longer human lives."
The Risks of AI Compiling Your Data
To be sure, the advent of AI-infused medical technology is not without its risks. One risk is that the use of AI wearables constantly monitoring our vital signs could turn us into a nation of hypochondriacs, racing to our doctors every time there's a blip in some vital sign. Such a development could stress an already overburdened system that suffers from, among other things, a shortage of doctors and nurses. Another risk has to do with the privacy protections on the massive repository of intimately personal information that AI will have on us.
In an article recently published in the Journal of the American Medical Association, Australian researcher Kit Huckvale and colleagues examined the handling of data by 36 smartphone apps that assisted people with either depression or smoking cessation, two areas that could lend themselves to stigmatization if they fell into the wrong hands.
Out of the 36 apps, 33 shared their data with third parties, despite the fact that just 25 of those apps had a privacy policy at all and out of those, only 23 stated that data would be shared with third parties. The recipients of all that data? It went almost exclusively to Facebook and Google, to be used for advertising and marketing purposes. But there's nothing to stop it from ending up in the hands of insurers, background databases, or any other entity.
Even when data isn't voluntarily shared, any digital information can be hacked. EHRs and even wearable devices share the same vulnerability as any other digital record or device. Still, the promise of AI to radically improve efficiency and accuracy in healthcare is hard to ignore.
AI Can Help Restore Humanity to Medicine
Eric Topol, director of the Scripps Research Translational Institute and author of the new book Deep Medicine, says that AI gives doctors and nurses the most precious gift of all: time.
Topol welcomes his patients' use of the Apple Watch cardiac feature and is optimistic about the ways that AI is revolutionizing medicine. He says that the watch helps doctors monitor how well medications are working and has already helped to prevent strokes. But in addition to that, AI will help bring the humanity back to a profession that has become as cold and hard as a stainless steel dissection table.
"When I graduated from medical school in the 1970s," he says, "you had a really intimate relationship with your doctor." Over the decades, he has seen that relationship steadily erode as medical organizations demanded that doctors see more and more patients within ever-shrinking time windows.
"Doctors have no time to think, to communicate. We need to restore the mission in medicine."
In addition to that, EHRs have meant that doctors and nurses are getting buried in paperwork and administrative tasks. This is no doubt one reason why a recent study by the World Health Organization showed that worldwide, about 50 percent of doctors suffer from burnout. People who are utterly exhausted make more mistakes, and medical clinicians are no different from the rest of us. Only medical mistakes have unacceptably high stakes. According to its website, Johns Hopkins University recently announced that in the U.S. alone, 250,000 people die from medical mistakes each year.
"Doctors have no time to think, to communicate," says Topol. "We need to restore the mission in medicine." AI is giving doctors more time to devote to the thing that attracted them to medicine in the first place—connecting deeply with patients.
There is a real danger at this juncture, though, that administrators aware of the time-saving aspects of AI will simply push doctors to see more patients, read more tests, and embrace an even more crushing workload.
"We can't leave it to the administrators to just make things worse," says Topol. "Now is the time for doctors to advocate for a restoration of the human touch. We need to stand up for patients and for the patient-doctor relationship."
AI could indeed be a game changer, he says, but rather than squander the huge benefits of more time, "We need a new equation going forward."
Recent leaps in technology represent an important step forward in unlocking artificial photosynthesis.
Since the beginning of life on Earth, plants have been naturally converting sunlight into energy. This photosynthesis process that's effortless for them has been anything but for scientists who have been trying to achieve artificial photosynthesis for the last half a century with the goal of creating a carbon-neutral fuel. Such a fuel could be a gamechanger — rather than putting CO2 back into the atmosphere like traditional fuels do, it would take CO2 out of the atmosphere and convert it into usable energy.
If given the option between a carbon-neutral fuel at the gas station and a fuel that produces carbon dioxide in spades -- and if costs and effectiveness were equal --who wouldn't choose the one best for the planet? That's the endgame scientists are after. A consumer switch to clean fuel could have a huge impact on our global CO2 emissions.
Up until this point, the methods used to make liquid fuel from atmospheric CO2 have been expensive, not efficient enough to really get off the ground, and often resulted in unwanted byproducts. But now, a new technology may be the key to unlocking the full potential of artificial photosynthesis. At the very least, it's a step forward and could help make a dent in atmospheric CO2 reduction.
"It's an important breakthrough in artificial photosynthesis," says Qian Wang, a researcher in the Department of Chemistry at Cambridge University and lead author on a recent study published in Nature about an innovation she calls "photosheets."
The latest version of the artificial leaf directly produces liquid fuel, which is easier to transport and use commercially.
These photosheets convert CO2, sunlight, and water into a carbon-neutral liquid fuel called formic acid without the aid of electricity. They're made of semiconductor powders that absorb sunlight. When in the presence of water and CO2, the electrons in the powders become excited and join with the CO2 and protons from the water molecules, reducing the CO2 in the process. The chemical reaction results in the production of formic acid, which can be used directly or converted to hydrogen, another clean energy fuel.
In the past, it's been difficult to reduce CO2 without creating a lot of unwanted byproducts. According to Wang, this new conversion process achieves the reduction and fuel creation with almost no byproducts.
The Cambridge team's new technology is a first and certainly momentous, but they're far from the only team to have produced fuel from CO2 using some form of artificial photosynthesis. More and more scientists are aiming to perfect the method in hopes of producing a truly sustainable, photosynthetic fuel capable of lowering carbon emissions.
Thanks to advancements in nanoscience, which has led to better control of materials, more successes are emerging. A team at the University of Illinois at Urbana-Champaign, for example, used gold nanoparticles as the photocatalysts in their process.
"My group demonstrated that you could actually use gold nanoparticles both as a light absorber and a catalyst in the process of converting carbon dioxide to hydrocarbons such as methane, ethane and propane fuels," says professor Prashant Jain, co-author of the study. Not only are gold nanoparticles great at absorbing light, they don't degrade as quickly as other metals, which makes them more sustainable.
That said, Jain's team, like every other research team working on artificial photosynthesis including the Cambridge team, is grappling with efficiency issues. Jain says that all parts of the process need to be optimized so the reaction can happen as quickly as possible.
"You can't just improve one [aspect], because that can lead to a decrease in performance in some other aspects," Jain explains.
The Cambridge team is currently experimenting with a range of catalysts to improve their device's stability and efficiency. Virgil Andrei, who is working on an artificial leaf design that was developed at Cambridge in 2019, was recently able to improve the performance and selectivity of the device. Now the leaf's solar-to-CO2 energy conversion efficiency is 0.2%, twice its previous efficiency.
The latest version also directly produces liquid fuel, which is easier to transport and use commercially.
In determining a method of fuel production's efficiency, one must consider how sustainable it is at every stage. That involves calculating whenever excess energy is needed to complete a step. According to Jain, in order to use CO2 for fuel production, you have to condense the CO2, which takes energy. And on the fuel production side, once the chemical reaction has created your byproducts, they need to be separated, which also takes energy.
To be truly sustainable, each part of the conversion system also needs to be durable. If parts need to be replaced often, or regularly maintained, that counts against it. Then you have to account for the system's reuse cycle. If you extract CO2 from the environment and convert it into fuel that's then put into a fuel cell, it's going to release CO2 at the other end. In order to create a fully green, carbon-neutral fuel source, that same amount of CO2 needs to be trapped and reintroduced back into the fuel conversion system.
"The cycle continues, and at each point, you will see a loss in efficiency, and depending on how much you [may also] see a loss in yield," says Jain. "And depending on what those efficiencies are at each one of those points will determine whether or not this process can be sustainable."
The science is at least a decade away from offering a competitive sustainable fuel option at scale. Streamlining a process to mimic what plants have perfected over billions of years is no small feat, but an ever-growing community of researchers using rapidly advancing technology is driving progress forward.
Genetic data sets skew too European, threatening to narrow who will benefit from future advances.
Genomics has begun its golden age. Just 20 years ago, sequencing a single genome cost nearly $3 billion and took over a decade. Today, the same feat can be achieved for a few hundred dollars and the better part of a day . Suddenly, the prospect of sequencing not just individuals, but whole populations, has become feasible.
The genetic differences between humans may seem meager, only around 0.1 percent of the genome on average, but this variation can have profound effects on an individual's risk of disease, responsiveness to medication, and even the dosage level that would work best.
Already, initiatives like the U.K.'s 100,000 Genomes Project - now expanding to 1 million genomes - and other similarly massive sequencing projects in Iceland and the U.S., have begun collecting population-scale data in order to capture and study this variation.
The resulting data sets are immensely valuable to researchers and drug developers working to design new 'precision' medicines and diagnostics, and to gain insights that may benefit patients. Yet, because the majority of this data comes from developed countries with well-established scientific and medical infrastructure, the data collected so far is heavily biased towards Western populations with largely European ancestry.
This presents a startling and fast-emerging problem: groups that are under-represented in these datasets are likely to benefit less from the new wave of therapeutics, diagnostics, and insights, simply because they were tailored for the genetic profiles of people with European ancestry.
We may indeed be approaching a golden age of genomics-enabled precision medicine. But if the data bias persists then there is a risk, as with most golden ages throughout history, that the benefits will not be equally accessible to all, and existing inequalities will only be exacerbated.
To remedy the situation, a number of initiatives have sprung up to sequence genomes of under-represented groups, adding them to the datasets and ensuring that they too will benefit from the rapidly unfolding genomic revolution.
Global Gene Corp
The idea behind Global Gene Corp was born eight years ago in Harvard when Sumit Jamuar, co-founder and CEO, met up with his two other co-founders, both experienced geneticists, for a coffee.
"They were discussing the limitless applications of understanding your genetic code," said Jamuar, a business executive from New Delhi.
"And so, being a technology enthusiast type, I was excited and I turned to them and said hey, this is incredible! Could you sequence me and give me some insights? And they actually just turned around and said no, because it's not going to be useful for you - there's not enough reference for what a good Sumit looks like."
What started as a curiosity-driven conversation on the power of genomics ended with a commitment to tackle one of the field's biggest roadblocks - its lack of global representation.
Jamuar set out to begin with India, which has about 20 percent of the world's population, including over 4000 different ethnicities, but contributes less than 2 percent of genomic data, he told Leaps.org.
Eight years later, Global Gene Corp's sequencing initiative is well underway, and is the largest in the history of the Indian subcontinent. The program is being carried out in collaboration with biotech giant Regeneron, with support from the Indian government, local communities, and the Indian healthcare ecosystem. In August 2020, Global Gene Corp's work was recognized through the $1 million 2020 Roddenberry award for organizations that advance the vision of 'Star Trek' creator Gene Roddenberry to better humanity.
This problem has already begun to manifest itself in, for example, much higher levels of genetic misdiagnosis among non-Europeans tested for their risk of certain diseases, such as hypertrophic cardiomyopathy - an inherited disease of the heart muscle.
Global Gene Corp also focuses on developing and implementing AI and machine learning tools to make sense of the deluge of genomic data. These tools are increasingly used by both industry and academia to guide future research by identifying particularly promising or clinically interesting genetic variants. But if the underlying data is skewed European, then the effectiveness of the computational analysis - along with the future advances and avenues of research that emerge from it - will be skewed towards Europeans too.
This problem has already begun to manifest itself in, for example, much higher levels of genetic misdiagnosis among non-Europeans tested for their risk of certain diseases, such as hypertrophic cardiomyopathy - an inherited disease of the heart muscle. Most of the genetic variants used in these tests were identified as being causal for the disease from studies of European genomes. However, many of these variants differ both in their distribution and clinical significance across populations, leading to many patients of non-European ancestry receiving false-positive test results - as their benign genetic variants were misclassified as pathogenic. Had even a small number of genomes from other ethnicities been included in the initial studies, these misdiagnoses could have been avoided.
"Unless we have a data set which is unbiased and representative, we're never going to achieve the success that we want," Jamuar says.
"When Siri was first launched, she could hardly recognize an accent which was not of a certain type, so if I was trying to speak to Siri, I would have to repeat myself multiple times and try to mimic an accent which wasn't my accent so that she could understand it.
"But over time the voice recognition technology improved tremendously because the training data was expanded to include people of very diverse backgrounds and their accents, so the algorithms were trained to be able to pick that up and it dramatically improved the technology. That's the way we have to think about it - without that good-quality diverse data, we will never be able to achieve the full potential of the computational tools."
While mapping India's rich genetic diversity has been the organization's primary focus so far, they plan, in time, to expand their work to other under-represented groups in Asia, the Middle East, Africa, and Latin America.
"As other like-minded people and partners join the mission, it just accelerates the achievement of what we have set out to do, which is to map out and organize the world's genomic diversity so that we can enable high-quality life and longevity benefits for everyone, everywhere," Jamuar says.
Empowering African Genomics
Africa is the birthplace of our species, and today still retains an inordinate amount of total human genetic diversity. Groups that left Africa and went on to populate the rest of the world, some 50 to 100,000 years ago, were likely small in number and only took a fraction of the total genetic diversity with them. This ancient bottleneck means that no other group in the world can match the level of genetic diversity seen in modern African populations.
Despite Africa's central importance in understanding the history and extent of human genetic diversity, the genomics of African populations remains wildly understudied. Addressing this disparity has become a central focus of the H3Africa Consortium, an initiative formally launched in 2012 with support from the African Academy of Sciences, the U.S. National Institutes of Health, and the UK's Wellcome Trust. Today, H3Africa supports over 50 projects across the continent, on an array of different research areas in genetics relevant to the health and heredity of Africans.
"Africa is the cradle of Humankind. So what that really means is that the populations that are currently living in Africa are among some of the oldest populations on the globe, and we know that the longer populations have had to go through evolutionary phases, the more variation there is in the genomes of people who live presently," says Zane Lombard, a principal investigator at H3Africa and Associate Professor of Human Genetics at the University of the Witwatersrand in Johannesburg, South Africa.
"So for that reason, African populations carry a huge amount of genetic variation and diversity, which is pretty much uncaptured. There's still a lot to learn as far as novel variation is concerned by looking at and studying African genomes."
A recent landmark H3Africa study, led by Lombard and published in Nature in October, sequenced the genomes of over 400 African individuals from 50 ethno-linguistic groups - many of which had never been sampled before.
Despite the relatively modest number of individuals sequenced in the study, over three million previously undescribed genetic variants were found, and complex patterns of ancestral migration were uncovered.
"In some of these ethno-linguistic groups they don't have a word for DNA, so we've had to really think about how to make sure that we communicate the purposes of different studies to participants so that you have true informed consent," says Lombard.
"The objective," she explained, "was to try and fill some of the gaps for many of these populations for which we didn't have any whole genome sequences or any genetic variation data...because if we're thinking about the future of precision medicine, if the patient is a member of a specific group where we don't know a lot about the genomic variation that exists in that group, it makes it really difficult to start thinking about clinical interpretation of their data."
From H3Africa's conception, the consortium's goal has not only been to better represent Africa's staggering genetic diversity in genomic data sets, but also to build Africa's domestic genomics capabilities and empower a new generation of African researchers. By doing so, the hope is that Africans will be able to set their own genomics agenda, and leapfrog to new and better ways of doing the work.
"The training that has happened on the continent and the number of new scientists, new students, and fellows that have come through the process and are now enabled to start their own research groups, to grow their own research in their countries, to be a spokesperson for genomics research in their countries, and to build that political will to do these larger types of sequencing initiatives - that is really a significant outcome from H3Africa as well. Over and above all the science that's coming out," Lombard says.
"What has been created through H3Africa is just this locus of researchers and scientists and bioethicists who have the same goal at heart - to work towards adjusting the data bias and making sure that all global populations are represented in genomics."