The Algorithm Will See You Now
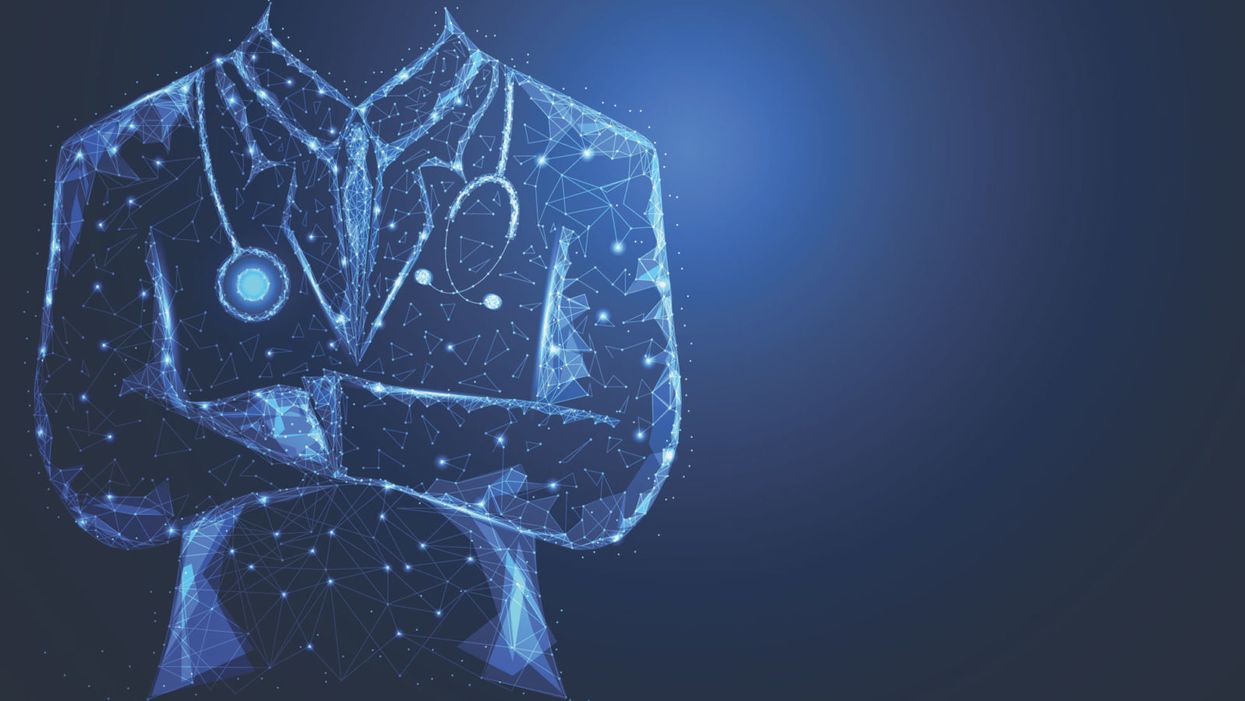
Artificial intelligence in medicine, still in an early phase, stands to transform how doctors and nurses spend their time.
There's a quiet revolution going on in medicine. It's driven by artificial intelligence, but paradoxically, new technology may put a more human face on healthcare.
AI's usefulness in healthcare ranges far and wide.
Artificial intelligence is software that can process massive amounts of information and learn over time, arriving at decisions with striking accuracy and efficiency. It offers greater accuracy in diagnosis, exponentially faster genome sequencing, the mining of medical literature and patient records at breathtaking speed, a dramatic reduction in administrative bureaucracy, personalized medicine, and even the democratization of healthcare.
The algorithms that bring these advantages won't replace doctors; rather, by offloading some of the most time-consuming tasks in healthcare, providers will be able to focus on personal interactions with patients—listening, empathizing, educating and generally putting the care back in healthcare. The relationship can focus on the alleviation of suffering, both the physical and emotional kind.
Challenges of Getting AI Up and Running
The AI revolution, still in its early phase in medicine, is already spurring some amazing advances, despite the fact that some experts say it has been overhyped. IBM's Watson Health program is a case in point. IBM capitalized on Watson's ability to process natural language by designing algorithms that devour data like medical articles and analyze images like MRIs and medical slides. The algorithms help diagnose diseases and recommend treatment strategies.
But Technology Review reported that a heavily hyped partnership with the MD Anderson Cancer Center in Houston fell apart in 2017 because of a lack of data in the proper format. The data existed, just not in a way that the voraciously data-hungry AI could use to train itself.
The hiccup certainly hasn't dampened the enthusiasm for medical AI among other tech giants, including Google and Apple, both of which have invested billions in their own healthcare projects. At this point, the main challenge is the need for algorithms to interpret a huge diversity of data mined from medical records. This can include everything from CT scans, MRIs, electrocardiograms, x-rays, and medical slides, to millions of pages of medical literature, physician's notes, and patient histories. It can even include data from implantables and wearables such as the Apple Watch and blood sugar monitors.
None of this information is in anything resembling a standard format across and even within hospitals, clinics, and diagnostic centers. Once the algorithms are trained, however, they can crunch massive amounts of data at blinding speed, with an accuracy that matches and sometimes even exceeds that of highly experienced doctors.
Genome sequencing, for example, took years to accomplish as recently as the early 2000s. The Human Genome Project, the first sequencing of the human genome, was an international effort that took 13 years to complete. In April of this year, Rady Children's Institute for Genomic Medicine in San Diego used an AI-powered genome sequencing algorithm to diagnose rare genetic diseases in infants in about 20 hours, according to ScienceDaily.
"Patient care will always begin and end with the doctor."
Dr. Stephen Kingsmore, the lead author of an article published in Science Translational Medicine, emphasized that even though the algorithm helped guide the treatment strategies of neonatal intensive care physicians, the doctor was still an indispensable link in the chain. "Some people call this artificial intelligence, we call it augmented intelligence," he says. "Patient care will always begin and end with the doctor."
One existing trend is helping to supply a great amount of valuable data to algorithms—the electronic health record. Initially blamed for exacerbating the already crushing workload of many physicians, the EHR is emerging as a boon for algorithms because it consolidates all of a patient's data in one record.
Examples of AI in Action Around the Globe
If you're a parent who has ever taken a child to the doctor with flulike symptoms, you know the anxiety of wondering if the symptoms signal something serious. Kang Zhang, M.D., Ph.D., the founding director of the Institute for Genomic Medicine at the University of California at San Diego, and colleagues developed an AI natural language processing model that used deep learning to analyze the EHRs of 1.3 million pediatric visits to a clinic in Guanzhou, China.
The AI identified common childhood diseases with about the same accuracy as human doctors, and it was even able to split the diagnoses into two categories—common conditions such as flu, and serious, life-threatening conditions like meningitis. Zhang has emphasized that the algorithm didn't replace the human doctor, but it did streamline the diagnostic process and could be used in a triage capacity when emergency room personnel need to prioritize the seriously ill over those suffering from common, less dangerous ailments.
AI's usefulness in healthcare ranges far and wide. In Uganda and several other African nations, AI is bringing modern diagnostics to remote villages that have no access to traditional technologies such as x-rays. The New York Times recently reported that there, doctors are using a pocket-sized, hand-held ultrasound machine that works in concert with a cell phone to image and diagnose everything from pneumonia (a common killer of children) to cancerous tumors.
The beauty of the highly portable, battery-powered device is that ultrasound images can be uploaded on computers so that physicians anywhere in the world can review them and weigh in with their advice. And the images are instantly incorporated into the patient's EHR.
Jonathan Rothberg, the founder of Butterfly Network, the Connecticut company that makes the device, told The New York Times that "Two thirds of the world's population gets no imaging at all. When you put something on a chip, the price goes down and you democratize it." The Butterfly ultrasound machine, which sells for $2,000, promises to be a game-changer in remote areas of Africa, South America, and Asia, as well as at the bedsides of patients in developed countries.
AI algorithms are rapidly emerging in healthcare across the U.S. and the world. China has become a major international player, set to surpass the U.S. this year in AI capital investment, the translation of AI research into marketable products, and even the number of often-cited research papers on AI. So far the U.S. is still the leader, but some experts describe the relationship between the U.S. and China as an AI cold war.
"The future of machine learning isn't sentient killer robots. It's longer human lives."
The U.S. Food and Drug Administration expanded its approval of medical algorithms from two in all of 2017 to about two per month throughout 2018. One of the first fields to be impacted is ophthalmology.
One algorithm, developed by the British AI company DeepMind (owned by Alphabet, the parent company of Google), instantly scans patients' retinas and is able to diagnose diabetic retinopathy without needing an ophthalmologist to interpret the scans. This means diabetics can get the test every year from their family physician without having to see a specialist. The Financial Times reported in March that the technology is now being used in clinics throughout Europe.
In Copenhagen, emergency service dispatchers are using a new voice-processing AI called Corti to analyze the conversations in emergency phone calls. The algorithm analyzes the verbal cues of callers, searches its huge database of medical information, and provides dispatchers with onscreen diagnostic information. Freddy Lippert, the CEO of EMS Copenhagen, notes that the algorithm has already saved lives by expediting accurate diagnoses in high-pressure situations where time is of the essence.
Researchers at the University of Nottingham in the UK have even developed a deep learning algorithm that predicts death more accurately than human clinicians. The algorithm incorporates data from a huge range of factors in a chronically ill population, including how many fruits and vegetables a patient eats on a daily basis. Dr. Stephen Weng, lead author of the study, published in PLOS ONE, said in a press release, "We found machine learning algorithms were significantly more accurate in predicting death than the standard prediction models developed by a human expert."
New digital technologies are allowing patients to participate in their healthcare as never before. A feature of the new Apple Watch is an app that detects cardiac arrhythmias and even produces an electrocardiogram if an abnormality is detected. The technology, approved by the FDA, is helping cardiologists monitor heart patients and design interventions for those who may be at higher risk of a cardiac event like a stroke.
If having an algorithm predict your death sends a shiver down your spine, consider that algorithms may keep you alive longer. In 2018, technology reporter Tristan Greene wrote for Medium that "…despite the unending deluge of panic-ridden articles declaring AI the path to apocalypse, we're now living in a world where algorithms save lives every day. The future of machine learning isn't sentient killer robots. It's longer human lives."
The Risks of AI Compiling Your Data
To be sure, the advent of AI-infused medical technology is not without its risks. One risk is that the use of AI wearables constantly monitoring our vital signs could turn us into a nation of hypochondriacs, racing to our doctors every time there's a blip in some vital sign. Such a development could stress an already overburdened system that suffers from, among other things, a shortage of doctors and nurses. Another risk has to do with the privacy protections on the massive repository of intimately personal information that AI will have on us.
In an article recently published in the Journal of the American Medical Association, Australian researcher Kit Huckvale and colleagues examined the handling of data by 36 smartphone apps that assisted people with either depression or smoking cessation, two areas that could lend themselves to stigmatization if they fell into the wrong hands.
Out of the 36 apps, 33 shared their data with third parties, despite the fact that just 25 of those apps had a privacy policy at all and out of those, only 23 stated that data would be shared with third parties. The recipients of all that data? It went almost exclusively to Facebook and Google, to be used for advertising and marketing purposes. But there's nothing to stop it from ending up in the hands of insurers, background databases, or any other entity.
Even when data isn't voluntarily shared, any digital information can be hacked. EHRs and even wearable devices share the same vulnerability as any other digital record or device. Still, the promise of AI to radically improve efficiency and accuracy in healthcare is hard to ignore.
AI Can Help Restore Humanity to Medicine
Eric Topol, director of the Scripps Research Translational Institute and author of the new book Deep Medicine, says that AI gives doctors and nurses the most precious gift of all: time.
Topol welcomes his patients' use of the Apple Watch cardiac feature and is optimistic about the ways that AI is revolutionizing medicine. He says that the watch helps doctors monitor how well medications are working and has already helped to prevent strokes. But in addition to that, AI will help bring the humanity back to a profession that has become as cold and hard as a stainless steel dissection table.
"When I graduated from medical school in the 1970s," he says, "you had a really intimate relationship with your doctor." Over the decades, he has seen that relationship steadily erode as medical organizations demanded that doctors see more and more patients within ever-shrinking time windows.
"Doctors have no time to think, to communicate. We need to restore the mission in medicine."
In addition to that, EHRs have meant that doctors and nurses are getting buried in paperwork and administrative tasks. This is no doubt one reason why a recent study by the World Health Organization showed that worldwide, about 50 percent of doctors suffer from burnout. People who are utterly exhausted make more mistakes, and medical clinicians are no different from the rest of us. Only medical mistakes have unacceptably high stakes. According to its website, Johns Hopkins University recently announced that in the U.S. alone, 250,000 people die from medical mistakes each year.
"Doctors have no time to think, to communicate," says Topol. "We need to restore the mission in medicine." AI is giving doctors more time to devote to the thing that attracted them to medicine in the first place—connecting deeply with patients.
There is a real danger at this juncture, though, that administrators aware of the time-saving aspects of AI will simply push doctors to see more patients, read more tests, and embrace an even more crushing workload.
"We can't leave it to the administrators to just make things worse," says Topol. "Now is the time for doctors to advocate for a restoration of the human touch. We need to stand up for patients and for the patient-doctor relationship."
AI could indeed be a game changer, he says, but rather than squander the huge benefits of more time, "We need a new equation going forward."
Researchers Are Discovering How to Predict – and Maybe Treat — Pregnancy Complications Early On.
Katie Love cradles her newborn daughter, born after a bout with preeclampsia.
Katie Love wishes there was some way she could have been prepared. But there was no way to know, early in 2020, that her pregnancy would lead to terrifyingly high blood pressure and multiple hospital visits, ending in induced labor and a 56-hour-long, “nightmare” delivery at 37 weeks. Love, a social media strategist in Pittsburgh, had preeclampsia, a poorly understood and potentially deadly pregnancy complication that affects 1 in 25 pregnant women in the United States. But there was no blood test, no easy diagnostic marker to warn Love that this might happen. Even on her first visit to the emergency room, with sky-high blood pressure, doctors could not be certain preeclampsia was the cause.
In fact, the primary but imperfect indicators for preeclampsia — high blood pressure and protein in the urine — haven’t changed in decades. The Preeclampsia Foundation calls a simple, rapid test to predict or diagnose the condition “a key component needed in the fight.”
Another common pregnancy complication is preterm birth, which affects 1 in 10 U.S. pregnancies, but there are few options to predict that might happen, either.
“The best tool that obstetricians have at the moment is still a tape measure and a blood pressure cuff to diagnose whatever’s happening in your pregnancy,” says Fiona Kaper, a vice president at the DNA-sequencing company Illumina in San Diego.
The hunt for such specific biomarkers is now taking off, at Illumina and elsewhere, as scientists probe maternal blood for signs that could herald pregnancy problems. These same molecules offer clues that might lead to more specific treatments. So far, it’s clear that many complications start with the placenta, the temporary organ that transfers nutrients, oxygen and waste between mother and fetus, and that these problems often start well before symptoms arise. Researchers are using the latest stem-cell technology to better understand the causes of complications and test treatments.
Pressing Need
Obstetricians aren’t flying completely blind; medical history can point to high or low risk for pregnancy complications. But ultimately, “everybody who’s pregnant is at risk for preeclampsia,” says Sarosh Rana, chief of maternal-fetal medicine at University of Chicago Medicine and an advisor to the Preeclampsia Foundation. And the symptoms of the condition include problems like headache and swollen feet that overlap with those of pregnancy in general, complicating diagnoses.
The “holy grail" would be early, first-trimester biomarkers. If obstetricians and expecting parents could know, in the first few months of pregnancy, that preeclampsia is a risk, a pregnant woman could monitor her blood pressure at home and take-low dose aspirin that might stave it off.
There are a couple more direct tests physicians can turn to, but these are imperfect. For preterm labor, fetal fibronectin makes up a sort of glue that keeps the amniotic sac, which cushions the unborn baby, attached to the uterus. If it’s not present near a woman’s cervix, that’s a good indicator that she’s not in labor, and can be safely sent home, says Lauren Demosthenes, an obstetrician and senior medical director of the digital health company Babyscripts in Washington, D.C. But if fibronectin appears, it might or might not indicate preterm labor.
“What we want is a test that gives us a positive predictive [signal],” says Demosthenes. “I want to know, if I get it, is it really going to predict preterm birth, or is it just going to make us worry more and order more tests?” In fact, the fetal fibronectin test hasn’t been shown to improve pregnancy outcomes, and Demosthenes says it’s fallen out of favor in many clinics.
Similarly, there’s a blood test, based on the ratio of the amounts of two different proteins, that can rule out preeclampsia but not confirm it’s happening. It’s approved in many countries, though not the U.S.; studies are still ongoing. A positive test, which means “maybe preeclampsia,” still leaves doctors and parents-to-be facing excruciating decisions: If the mother’s life is in danger, delivering the baby can save her, but even a few more days in the uterus can promote the baby’s health. In Ireland, where the test is available, it’s not getting much use, says Patricia Maguire, director of the University College Dublin Institute for Discovery.
Maguire has identified proteins released by platelets that indicate pregnancy — the “most expensive pregnancy test in the world,” she jokes. She is now testing those markers in women with suspected preeclampsia.
The “holy grail,” says Maguire, would be early, first-trimester biomarkers. If obstetricians and expecting parents could know, in the first few months of pregnancy, that preeclampsia is a risk, a pregnant woman could monitor her blood pressure at home and take-low dose aspirin that might stave it off. Similarly, if a quick blood test indicated that preterm labor could happen, doctors could take further steps such as measuring the cervix and prescribing progesterone if it’s on the short side.
Biomarkers in Blood
It was fatherhood that drew Stephen Quake, a biophysicist at Stanford University in California, to the study of pregnancy biomarkers. His wife, pregnant with their first child in 2001, had a test called amniocentesis. That involves extracting a sample from within the uterus, using a 3–8-inch-long needle, for genetic testing. The test can identify genetic differences, such as Down syndrome, but also carries risks including miscarriage or infection. In this case, mom and baby were fine (Quake’s daughter is now a college student), but he found the diagnostic danger unacceptable.
Seeking a less invasive test, Quake in 2008 reported that there’s enough fetal DNA in the maternal bloodstream to diagnose Down syndrome and other genetic conditions. “Use of amniocentesis has plunged,” he says.
Then, recalling that his daughter was born three and a half weeks before her due date — and that Quake’s own mom claims he was a month late, which makes him think the due date must have been off — he started researching markers that could accurately assess a fetus’ age and predict the timing of labor. In this case, Quake was interested in RNA, not DNA, because it’s a signal of which genes the fetus’, placenta’s, and mother’s tissues are using to create proteins. Specifically, these are RNAs that have exited the cells that made them. Tissues can use such free RNAs as messages, wrapping them in membranous envelopes to travel the bloodstream to other body parts. Dying cells also release fragments containing RNAs. “A lot of information is in there,” says Kaper.
In a small study of 31 healthy pregnant women, published in 2018, Quake and collaborators discovered nine RNAs that could predict gestational age, which indicates due date, just as well as ultrasound. With another set of 38 women, including 13 who delivered early, the researchers discovered seven RNAs that predicted preterm labor up to two months in advance.
Quake notes that an RNA-based blood test is cheaper and more portable than ultrasound, so it might be useful in the developing world. A company he cofounded, Mirvie, Inc., is now analyzing RNA’s predictive value further, in thousands of diverse women. CEO and cofounder Maneesh Jain says that since preterm labor is so poorly understood, they’re sequencing RNAs that represent about 20,000 genes — essentially all the genes humans have — to find the very best biomarkers. “We don’t know enough about this field to guess what it might be,” he says. “We feel we’ve got to cast the net wide.”
Quake, and Mirvie, are now working on biomarkers for preeclampsia. In a recent preprint study, not yet reviewed by other experts, Quake’s Stanford team reported 18 RNAs that, measured before 16 weeks, correctly predicted preeclampsia 56–100% of the time.
Other researchers are taking a similar tack. Kaper’s team at Illumina was able to classify preeclampsia from bloodstream RNAs with 85 to 89% accuracy, though they didn’t attempt to predict it. And Louise Laurent, a maternal-fetal medicine specialist and researcher at the University of California, San Diego (UCSD), has defined several pairs of microRNAs — pint-sized RNAs that regulate other ones — in second-trimester blood samples that predict preeclampsia later on.
Placentas in a Dish
The RNAs that show up in these studies often come from genes used by the placenta. But they’re only signals that something’s wrong, not necessarily the root cause. “There still is not much known about what really causes major complications of pregnancy,” says Laurent.
The challenge is that placental problems likely occur early on, as the organ forms in the first trimester. For example, if the placenta did a poor job of building blood vessels through the uterine lining, it might cause preeclampsia later as the growing fetus tries to access more and more blood through insufficient vessels, leading to high blood pressure in the mother. “Everyone has kind of suspected that that is probably what goes wrong,” says Mana Parast, a pathologist and researcher at UCSD.
To see how a placenta first faltered, “you want to go back in time,” says Parast. It’s only recently become possible to do something akin to that: She and Laurent take cells from the umbilical cord (which is a genetic match for the placenta) at the end of pregnancy, and turn them into stem cells, which can become any kind of cell. They then nudge those stem cells to make new placenta cells in lab dishes. But when the researchers start with cells from an umbilical cord after preeclampsia, they find the stem cells struggle to even form proper placenta cells, or they develop abnormally. So yes, something seems to go wrong right at the beginning. Now, the team plans to use these cell cultures to study the microRNAs that indicate preeclampsia risk, and to look for medications that might reverse the problems, Parast says.
Biomarkers could lead to treatments. For example, one of the proteins that commercial preeclampsia diagnostic kits test for is called soluble Flt-1. It’s a sort of anti-growth factor, explains Rana, that can cause problems with blood vessels and thus high blood pressure. Getting rid of the extra Flt-1, then, might alleviate symptoms and keep the mother safe, giving the baby more time to develop. Indeed, a small trial that filtered this protein from the blood did lower blood pressure, allowing participants to keep their babies inside for a couple of weeks longer, researchers reported in 2011.
For pregnant women like Love, even advance warning would have been beneficial. Laurent and others envision a first-trimester blood test that would use different kinds of biomolecules — RNAs, proteins, whatever works best — to indicate whether a pregnancy is at low, medium, or high risk for common complications.
“I prefer to be prepared,” says Love, now the mother of a healthy little girl. “I just wouldn’t have been so thrown off by the whole thing.”
Dec. 17th Event: The Latest on Omicron, Boosters, and Immunity
The Omicron variant poses new uncertainty for the vaccines, which four leading experts will address during our virtual event on December 17th, 2021.
This virtual event will convene leading scientific and medical experts to discuss the most pressing questions around the new Omicron variant, including what we know so far about its ability to evade COVID-19 vaccines, the role of boosters in eliciting heightened immunity, and the science behind variants and vaccines. A public Q&A will follow the expert discussion.
EVENT INFORMATION:
Date: Friday Dec 17, 2021
2:00pm - 3:30pm EST
Dr. Céline Gounder, MD, ScM, is the CEO/President/Founder of Just Human Productions, a non-profit multimedia organization. She is also the host and producer of American Diagnosis, a podcast on health and social justice, and Epidemic, a podcast about infectious disease epidemics and pandemics. She served on the Biden-Harris Transition COVID-19 Advisory Board.
Dr. Theodora Hatziioannou, Ph.D., is a Research Associate Professor in the Laboratory of Retrovirology at The Rockefeller University. Her research includes identifying plasma samples from recovered COVID-19 patients that contain antibodies capable of neutralizing the SARS-CoV-2 coronavirus.
Dr. Onyema Ogbuagu, MBBCh, is an Associate Professor at Yale School of Medicine and an infectious disease specialist who treats COVID-19 patients and leads Yale’s clinical studies around COVID-19. He ran Yale’s trial of the Pfizer/BioNTech vaccine.
Dr. Eric Topol, M.D., is a cardiologist, scientist, professor of molecular medicine, and the director and founder of Scripps Research Translational Institute. He has led clinical trials in over 40 countries with over 200,000 patients and pioneered the development of many routinely used medications.
This event is the fourth of a four-part series co-hosted by Leaps.org, the Aspen Institute Science & Society Program, and the Sabin–Aspen Vaccine Science & Policy Group, with generous support from the Gordon and Betty Moore Foundation and the Howard Hughes Medical Institute.
Kira Peikoff was the editor-in-chief of Leaps.org from 2017 to 2021. As a journalist, her work has appeared in The New York Times, Newsweek, Nautilus, Popular Mechanics, The New York Academy of Sciences, and other outlets. She is also the author of four suspense novels that explore controversial issues arising from scientific innovation: Living Proof, No Time to Die, Die Again Tomorrow, and Mother Knows Best. Peikoff holds a B.A. in Journalism from New York University and an M.S. in Bioethics from Columbia University. She lives in New Jersey with her husband and two young sons. Follow her on Twitter @KiraPeikoff.