The Death Predictor: A Helpful New Tool or an Ethical Morass?
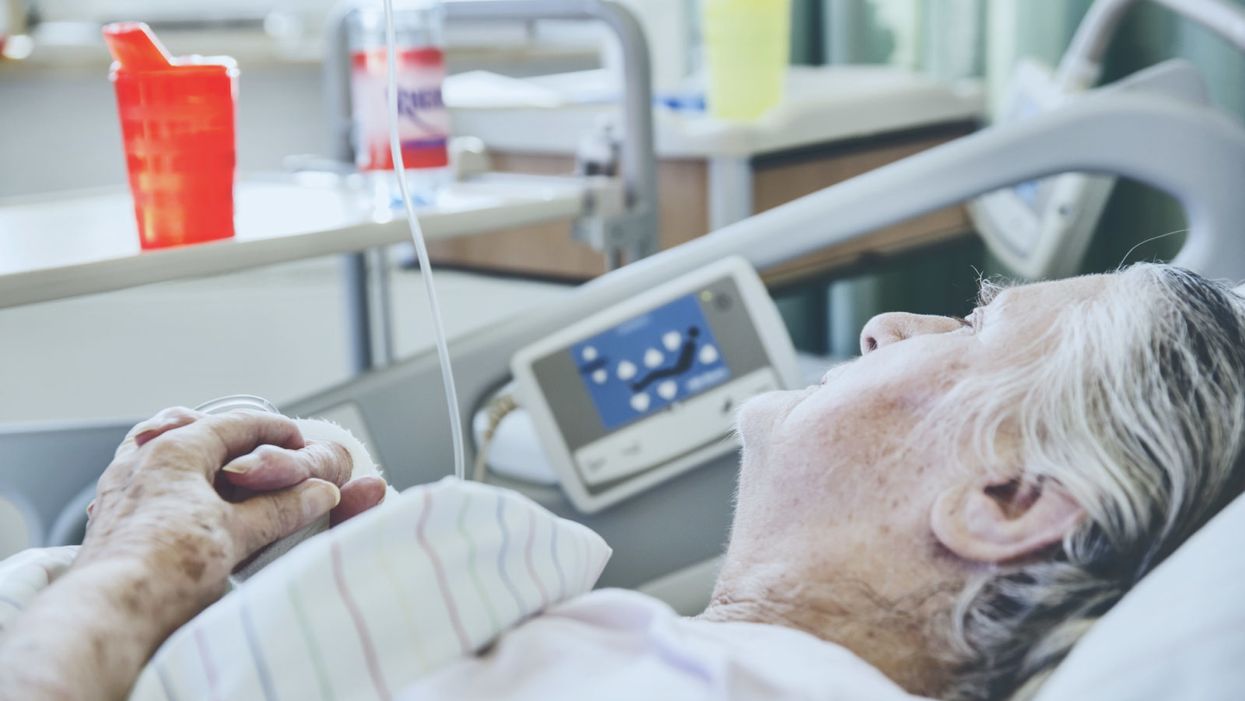
A senior in hospice care.
Whenever Eric Karl Oermann has to tell a patient about a terrible prognosis, their first question is always: "how long do I have?" Oermann would like to offer a precise answer, to provide some certainty and help guide treatment. But although he's one of the country's foremost experts in medical artificial intelligence, Oermann is still dependent on a computer algorithm that's often wrong.
Doctors are notoriously terrible at guessing how long their patients will live.
Artificial intelligence, now often called deep learning or neural networks, has radically transformed language and image processing. It's allowed computers to play chess better than the world's grand masters and outwit the best Jeopardy players. But it still can't precisely tell a doctor how long a patient has left – or how to help that person live longer.
Someday, researchers predict, computers will be able to watch a video of a patient to determine their health status. Doctors will no longer have to spend hours inputting data into medical records. And computers will do a better job than specialists at identifying tiny tumors, impending crises, and, yes, figuring out how long the patient has to live. Oermann, a neurosurgeon at Mount Sinai, says all that technology will allow doctors to spend more time doing what they do best: talking with their patients. "I want to see more deep learning and computers in a clinical setting," he says, "so there can be more human interaction." But those days are still at least three to five years off, Oermann and other researchers say.
Doctors are notoriously terrible at guessing how long their patients will live, says Nigam Shah, an associate professor at Stanford University and assistant director of the school's Center for Biomedical Informatics Research. Doctors don't want to believe that their patient – whom they've come to like – will die. "Doctors over-estimate survival many-fold," Shah says. "How do you go into work, in say, oncology, and not be delusionally optimistic? You have to be."
But patients near the end of life will get better treatment – and even live longer – if they are overseen by hospice or palliative care, research shows. So, instead of relying on human bias to select those whose lives are nearing their end, Shah and his colleagues showed that they could use a deep learning algorithm based on medical records to flag incoming patients with a life expectancy of three months to a year. They use that data to indicate who might need palliative care. Then, the palliative care team can reach out to treating physicians proactively, instead of relying on their referrals or taking the time to read extensive medical charts.
But, although the system works well, Shah isn't yet sure if such indicators actually get the appropriate patients into palliative care. He's recently partnered with a palliative care doctor to run a gold-standard clinical trial to test whether patients who are flagged by this algorithm are indeed a better match for palliative care.
"What is effective from a health system perspective might not be effective from a treating physician's perspective and might not be effective from the patient's perspective," Shah notes. "I don't have a good way to guess everybody's reaction without actually studying it." Whether palliative care is appropriate, for instance, depends on more than just the patient's health status. "If the patient's not ready, the family's not ready and the doctor's not ready, then you're just banging your head against the wall," Shah says. "Given limited capacity, it's a waste of resources" to put that person in palliative care.
The algorithm isn't perfect, but "on balance, it leads to better decisions more often."
Alexander Smith and Sei Lee, both palliative care doctors, work together at the University of California, San Francisco, to develop predictions for patients who come to the hospital with a complicated prognosis or a history of decline. Their algorithm, they say, helps decide if this patient's problems – which might include diabetes, heart disease, a slow-growing cancer, and memory issues – make them eligible for hospice. The algorithm isn't perfect, they both agree, but "on balance, it leads to better decisions more often," Smith says.
Bethany Percha, an assistant professor at Mount Sinai, says that an algorithm may tell doctors that their patient is trending downward, but it doesn't do anything to change that trajectory. "Even if you can predict something, what can you do about it?" Algorithms may be able to offer treatment suggestions – but not what specific actions will alter a patient's future, says Percha, also the chief technology officer of Precise Health Enterprise, a product development group within Mount Sinai. And the algorithms remain challenging to develop. Electronic medical records may be great at her hospital, but if the patient dies at a different one, her system won't know. If she wants to be certain a patient has died, she has to merge social security records of death with her system's medical records – a time-consuming and cumbersome process.
An algorithm that learns from biased data will be biased, Shah says. Patients who are poor or African American historically have had worse health outcomes. If researchers train an algorithm on data that includes those biases, they get baked into the algorithms, which can then lead to a self-fulfilling prophesy. Smith and Lee say they've taken race out of their algorithms to avoid this bias.
Age is even trickier. There's no question that someone's risk of illness and death goes up with age. But an 85-year-old who breaks a hip running a marathon should probably be treated very differently than an 85-year-old who breaks a hip trying to get out of a chair in a dementia care unit. That's why the doctor can never be taken out of the equation, Shah says. Human judgment will always be required in medical care and an algorithm should never be followed blindly, he says.
Experts say that the flaws in artificial intelligence algorithms shouldn't prevent people from using them – carefully.
Researchers are also concerned that their algorithms will be used to ration care, or that insurance companies will use their data to justify a rate increase. If an algorithm predicts a patient is going to end up back in the hospital soon, "who's benefitting from knowing a patient is going to be readmitted? Probably the insurance company," Percha says.
Still, Percha and others say, the flaws in artificial intelligence algorithms shouldn't prevent people from using them – carefully. "These are new and exciting tools that have a lot of potential uses. We need to be conscious about how to use them going forward, but it doesn't mean we shouldn't go down this road," she says. "I think the potential benefits outweigh the risks, especially because we've barely scratched the surface of what big data can do right now."
Thanks to safety cautions from the COVID-19 pandemic, a strain of influenza has been completely eliminated.
If you were one of the millions who masked up, washed your hands thoroughly and socially distanced, pat yourself on the back—you may have helped change the course of human history.
Scientists say that thanks to these safety precautions, which were introduced in early 2020 as a way to stop transmission of the novel COVID-19 virus, a strain of influenza has been completely eliminated. This marks the first time in human history that a virus has been wiped out through non-pharmaceutical interventions, such as vaccines.
The flu shot, explained
Influenza viruses type A and B are responsible for the majority of human illnesses and the flu season.
Centers for Disease Control
For more than a decade, flu shots have protected against two types of the influenza virus–type A and type B. While there are four different strains of influenza in existence (A, B, C, and D), only strains A, B, and C are capable of infecting humans, and only A and B cause pandemics. In other words, if you catch the flu during flu season, you’re most likely sick with flu type A or B.
Flu vaccines contain inactivated—or dead—influenza virus. These inactivated viruses can’t cause sickness in humans, but when administered as part of a vaccine, they teach a person’s immune system to recognize and kill those viruses when they’re encountered in the wild.
Each spring, a panel of experts gives a recommendation to the US Food and Drug Administration on which strains of each flu type to include in that year’s flu vaccine, depending on what surveillance data says is circulating and what they believe is likely to cause the most illness during the upcoming flu season. For the past decade, Americans have had access to vaccines that provide protection against two strains of influenza A and two lineages of influenza B, known as the Victoria lineage and the Yamagata lineage. But this year, the seasonal flu shot won’t include the Yamagata strain, because the Yamagata strain is no longer circulating among humans.
How Yamagata Disappeared
Flu surveillance data from the Global Initiative on Sharing All Influenza Data (GISAID) shows that the Yamagata lineage of flu type B has not been sequenced since April 2020.
Nature
Experts believe that the Yamagata lineage had already been in decline before the pandemic hit, likely because the strain was naturally less capable of infecting large numbers of people compared to the other strains. When the COVID-19 pandemic hit, the resulting safety precautions such as social distancing, isolating, hand-washing, and masking were enough to drive the virus into extinction completely.
Because the strain hasn’t been circulating since 2020, the FDA elected to remove the Yamagata strain from the seasonal flu vaccine. This will mark the first time since 2012 that the annual flu shot will be trivalent (three-component) rather than quadrivalent (four-component).
Should I still get the flu shot?
The flu shot will protect against fewer strains this year—but that doesn’t mean we should skip it. Influenza places a substantial health burden on the United States every year, responsible for hundreds of thousands of hospitalizations and tens of thousands of deaths. The flu shot has been shown to prevent millions of illnesses each year (more than six million during the 2022-2023 season). And while it’s still possible to catch the flu after getting the flu shot, studies show that people are far less likely to be hospitalized or die when they’re vaccinated.
Another unexpected benefit of dropping the Yamagata strain from the seasonal vaccine? This will possibly make production of the flu vaccine faster, and enable manufacturers to make more vaccines, helping countries who have a flu vaccine shortage and potentially saving millions more lives.
After his grandmother’s dementia diagnosis, one man invented a snack to keep her healthy and hydrated.
Founder Lewis Hornby and his grandmother Pat, sampling Jelly Drops—an edible gummy containing water and life-saving electrolytes.
On a visit to his grandmother’s nursing home in 2016, college student Lewis Hornby made a shocking discovery: Dehydration is a common (and dangerous) problem among seniors—especially those that are diagnosed with dementia.
Hornby’s grandmother, Pat, had always had difficulty keeping up her water intake as she got older, a common issue with seniors. As we age, our body composition changes, and we naturally hold less water than younger adults or children, so it’s easier to become dehydrated quickly if those fluids aren’t replenished. What’s more, our thirst signals diminish naturally as we age as well—meaning our body is not as good as it once was in letting us know that we need to rehydrate. This often creates a perfect storm that commonly leads to dehydration. In Pat’s case, her dehydration was so severe she nearly died.
When Lewis Hornby visited his grandmother at her nursing home afterward, he learned that dehydration especially affects people with dementia, as they often don’t feel thirst cues at all, or may not recognize how to use cups correctly. But while dementia patients often don’t remember to drink water, it seemed to Hornby that they had less problem remembering to eat, particularly candy.
Where people with dementia often forget to drink water, they're more likely to pick up a colorful snack, Hornby found. alzheimers.org.uk
Hornby wanted to create a solution for elderly people who struggled keeping their fluid intake up. He spent the next eighteen months researching and designing a solution and securing funding for his project. In 2019, Hornby won a sizable grant from the Alzheimer’s Society, a UK-based care and research charity for people with dementia and their caregivers. Together, through the charity’s Accelerator Program, they created a bite-sized, sugar-free, edible jelly drop that looked and tasted like candy. The candy, called Jelly Drops, contained 95% water and electrolytes—important minerals that are often lost during dehydration. The final product launched in 2020—and was an immediate success. The drops were able to provide extra hydration to the elderly, as well as help keep dementia patients safe, since dehydration commonly leads to confusion, hospitalization, and sometimes even death.
Not only did Jelly Drops quickly become a favorite snack among dementia patients in the UK, but they were able to provide an additional boost of hydration to hospital workers during the pandemic. In NHS coronavirus hospital wards, patients infected with the virus were regularly given Jelly Drops to keep their fluid levels normal—and staff members snacked on them as well, since long shifts and personal protective equipment (PPE) they were required to wear often left them feeling parched.
In April 2022, Jelly Drops launched in the United States. The company continues to donate 1% of its profits to help fund Alzheimer’s research.