The Death Predictor: A Helpful New Tool or an Ethical Morass?
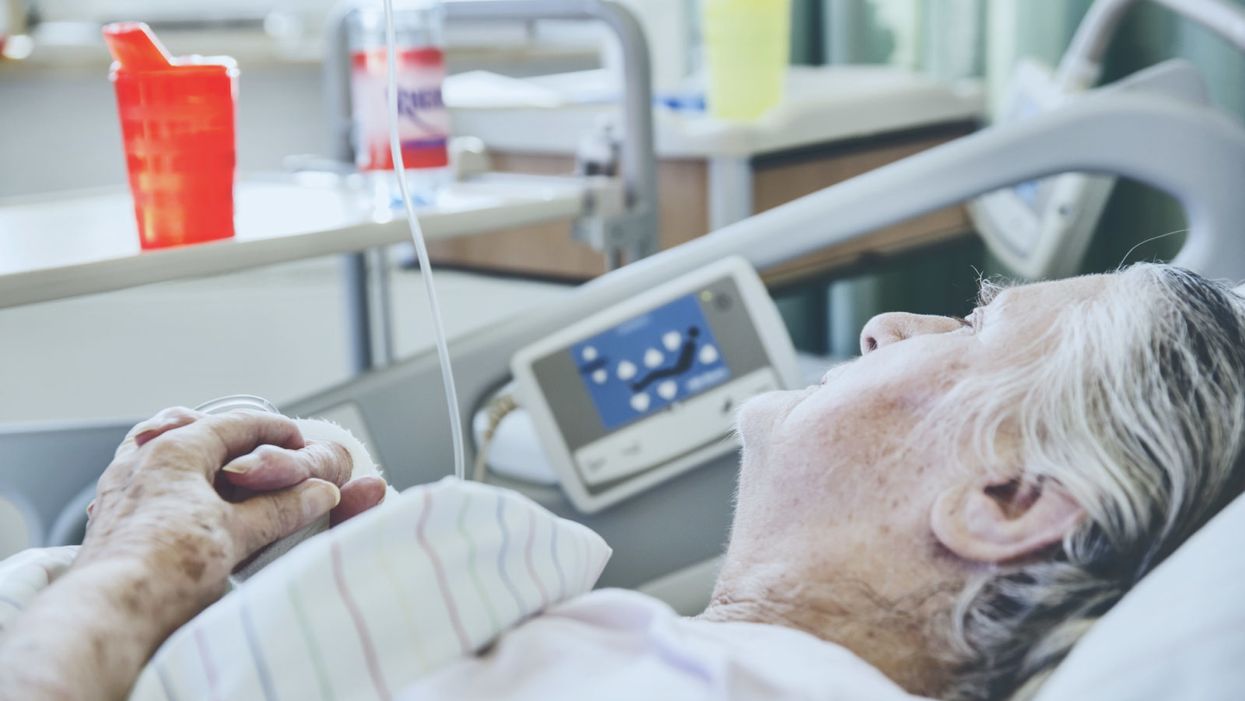
A senior in hospice care.
Whenever Eric Karl Oermann has to tell a patient about a terrible prognosis, their first question is always: "how long do I have?" Oermann would like to offer a precise answer, to provide some certainty and help guide treatment. But although he's one of the country's foremost experts in medical artificial intelligence, Oermann is still dependent on a computer algorithm that's often wrong.
Doctors are notoriously terrible at guessing how long their patients will live.
Artificial intelligence, now often called deep learning or neural networks, has radically transformed language and image processing. It's allowed computers to play chess better than the world's grand masters and outwit the best Jeopardy players. But it still can't precisely tell a doctor how long a patient has left – or how to help that person live longer.
Someday, researchers predict, computers will be able to watch a video of a patient to determine their health status. Doctors will no longer have to spend hours inputting data into medical records. And computers will do a better job than specialists at identifying tiny tumors, impending crises, and, yes, figuring out how long the patient has to live. Oermann, a neurosurgeon at Mount Sinai, says all that technology will allow doctors to spend more time doing what they do best: talking with their patients. "I want to see more deep learning and computers in a clinical setting," he says, "so there can be more human interaction." But those days are still at least three to five years off, Oermann and other researchers say.
Doctors are notoriously terrible at guessing how long their patients will live, says Nigam Shah, an associate professor at Stanford University and assistant director of the school's Center for Biomedical Informatics Research. Doctors don't want to believe that their patient – whom they've come to like – will die. "Doctors over-estimate survival many-fold," Shah says. "How do you go into work, in say, oncology, and not be delusionally optimistic? You have to be."
But patients near the end of life will get better treatment – and even live longer – if they are overseen by hospice or palliative care, research shows. So, instead of relying on human bias to select those whose lives are nearing their end, Shah and his colleagues showed that they could use a deep learning algorithm based on medical records to flag incoming patients with a life expectancy of three months to a year. They use that data to indicate who might need palliative care. Then, the palliative care team can reach out to treating physicians proactively, instead of relying on their referrals or taking the time to read extensive medical charts.
But, although the system works well, Shah isn't yet sure if such indicators actually get the appropriate patients into palliative care. He's recently partnered with a palliative care doctor to run a gold-standard clinical trial to test whether patients who are flagged by this algorithm are indeed a better match for palliative care.
"What is effective from a health system perspective might not be effective from a treating physician's perspective and might not be effective from the patient's perspective," Shah notes. "I don't have a good way to guess everybody's reaction without actually studying it." Whether palliative care is appropriate, for instance, depends on more than just the patient's health status. "If the patient's not ready, the family's not ready and the doctor's not ready, then you're just banging your head against the wall," Shah says. "Given limited capacity, it's a waste of resources" to put that person in palliative care.
The algorithm isn't perfect, but "on balance, it leads to better decisions more often."
Alexander Smith and Sei Lee, both palliative care doctors, work together at the University of California, San Francisco, to develop predictions for patients who come to the hospital with a complicated prognosis or a history of decline. Their algorithm, they say, helps decide if this patient's problems – which might include diabetes, heart disease, a slow-growing cancer, and memory issues – make them eligible for hospice. The algorithm isn't perfect, they both agree, but "on balance, it leads to better decisions more often," Smith says.
Bethany Percha, an assistant professor at Mount Sinai, says that an algorithm may tell doctors that their patient is trending downward, but it doesn't do anything to change that trajectory. "Even if you can predict something, what can you do about it?" Algorithms may be able to offer treatment suggestions – but not what specific actions will alter a patient's future, says Percha, also the chief technology officer of Precise Health Enterprise, a product development group within Mount Sinai. And the algorithms remain challenging to develop. Electronic medical records may be great at her hospital, but if the patient dies at a different one, her system won't know. If she wants to be certain a patient has died, she has to merge social security records of death with her system's medical records – a time-consuming and cumbersome process.
An algorithm that learns from biased data will be biased, Shah says. Patients who are poor or African American historically have had worse health outcomes. If researchers train an algorithm on data that includes those biases, they get baked into the algorithms, which can then lead to a self-fulfilling prophesy. Smith and Lee say they've taken race out of their algorithms to avoid this bias.
Age is even trickier. There's no question that someone's risk of illness and death goes up with age. But an 85-year-old who breaks a hip running a marathon should probably be treated very differently than an 85-year-old who breaks a hip trying to get out of a chair in a dementia care unit. That's why the doctor can never be taken out of the equation, Shah says. Human judgment will always be required in medical care and an algorithm should never be followed blindly, he says.
Experts say that the flaws in artificial intelligence algorithms shouldn't prevent people from using them – carefully.
Researchers are also concerned that their algorithms will be used to ration care, or that insurance companies will use their data to justify a rate increase. If an algorithm predicts a patient is going to end up back in the hospital soon, "who's benefitting from knowing a patient is going to be readmitted? Probably the insurance company," Percha says.
Still, Percha and others say, the flaws in artificial intelligence algorithms shouldn't prevent people from using them – carefully. "These are new and exciting tools that have a lot of potential uses. We need to be conscious about how to use them going forward, but it doesn't mean we shouldn't go down this road," she says. "I think the potential benefits outweigh the risks, especially because we've barely scratched the surface of what big data can do right now."
Scientists experiment with burning iron as a fuel source
Sparklers produce a beautiful display of light and heat by burning metal dust, which contains iron. The recent work of Canadian and Dutch researchers suggests we can use iron as a cheap, carbon-free fuel.
Story by Freethink
Try burning an iron metal ingot and you’ll have to wait a long time — but grind it into a powder and it will readily burst into flames. That’s how sparklers work: metal dust burning in a beautiful display of light and heat. But could we burn iron for more than fun? Could this simple material become a cheap, clean, carbon-free fuel?
In new experiments — conducted on rockets, in microgravity — Canadian and Dutch researchers are looking at ways of boosting the efficiency of burning iron, with a view to turning this abundant material — the fourth most common in the Earth’s crust, about about 5% of its mass — into an alternative energy source.
Iron as a fuel
Iron is abundantly available and cheap. More importantly, the byproduct of burning iron is rust (iron oxide), a solid material that is easy to collect and recycle. Neither burning iron nor converting its oxide back produces any carbon in the process.
Iron oxide is potentially renewable by reacting with electricity or hydrogen to become iron again.
Iron has a high energy density: it requires almost the same volume as gasoline to produce the same amount of energy. However, iron has poor specific energy: it’s a lot heavier than gas to produce the same amount of energy. (Think of picking up a jug of gasoline, and then imagine trying to pick up a similar sized chunk of iron.) Therefore, its weight is prohibitive for many applications. Burning iron to run a car isn’t very practical if the iron fuel weighs as much as the car itself.
In its powdered form, however, iron offers more promise as a high-density energy carrier or storage system. Iron-burning furnaces could provide direct heat for industry, home heating, or to generate electricity.
Plus, iron oxide is potentially renewable by reacting with electricity or hydrogen to become iron again (as long as you’ve got a source of clean electricity or green hydrogen). When there’s excess electricity available from renewables like solar and wind, for example, rust could be converted back into iron powder, and then burned on demand to release that energy again.
However, these methods of recycling rust are very energy intensive and inefficient, currently, so improvements to the efficiency of burning iron itself may be crucial to making such a circular system viable.
The science of discrete burning
Powdered particles have a high surface area to volume ratio, which means it is easier to ignite them. This is true for metals as well.
Under the right circumstances, powdered iron can burn in a manner known as discrete burning. In its most ideal form, the flame completely consumes one particle before the heat radiating from it combusts other particles in its vicinity. By studying this process, researchers can better understand and model how iron combusts, allowing them to design better iron-burning furnaces.
Discrete burning is difficult to achieve on Earth. Perfect discrete burning requires a specific particle density and oxygen concentration. When the particles are too close and compacted, the fire jumps to neighboring particles before fully consuming a particle, resulting in a more chaotic and less controlled burn.
Presently, the rate at which powdered iron particles burn or how they release heat in different conditions is poorly understood. This hinders the development of technologies to efficiently utilize iron as a large-scale fuel.
Burning metal in microgravity
In April, the European Space Agency (ESA) launched a suborbital “sounding” rocket, carrying three experimental setups. As the rocket traced its parabolic trajectory through the atmosphere, the experiments got a few minutes in free fall, simulating microgravity.
One of the experiments on this mission studied how iron powder burns in the absence of gravity.
In microgravity, particles float in a more uniformly distributed cloud. This allows researchers to model the flow of iron particles and how a flame propagates through a cloud of iron particles in different oxygen concentrations.
Existing fossil fuel power plants could potentially be retrofitted to run on iron fuel.
Insights into how flames propagate through iron powder under different conditions could help design much more efficient iron-burning furnaces.
Clean and carbon-free energy on Earth
Various businesses are looking at ways to incorporate iron fuels into their processes. In particular, it could serve as a cleaner way to supply industrial heat by burning iron to heat water.
For example, Dutch brewery Swinkels Family Brewers, in collaboration with the Eindhoven University of Technology, switched to iron fuel as the heat source to power its brewing process, accounting for 15 million glasses of beer annually. Dutch startup RIFT is running proof-of-concept iron fuel power plants in Helmond and Arnhem.
As researchers continue to improve the efficiency of burning iron, its applicability will extend to other use cases as well. But is the infrastructure in place for this transition?
Often, the transition to new energy sources is slowed by the need to create new infrastructure to utilize them. Fortunately, this isn’t the case with switching from fossil fuels to iron. Since the ideal temperature to burn iron is similar to that for hydrocarbons, existing fossil fuel power plants could potentially be retrofitted to run on iron fuel.
This article originally appeared on Freethink, home of the brightest minds and biggest ideas of all time.
How to Use Thoughts to Control Computers with Dr. Tom Oxley
Leaps.org talks with Dr. Tom Oxley, founding CEO of Synchron, a company that's taking a unique - and less invasive - approach to "brain-computer interfaces" for patients with ALS and other mobility challenges.
Tom Oxley is building what he calls a “natural highway into the brain” that lets people use their minds to control their phones and computers. The device, called the Stentrode, could improve the lives of hundreds of thousands of people living with spinal cord paralysis, ALS and other neurodegenerative diseases.
Leaps.org talked with Dr. Oxley for today’s podcast. A fascinating thing about the Stentrode is that it works very differently from other “brain computer interfaces” you may be familiar with, like Elon Musk’s Neuralink. Some BCIs are implanted by surgeons directly into a person’s brain, but the Stentrode is much less invasive. Dr. Oxley’s company, Synchron, opts for a “natural” approach, using stents in blood vessels to access the brain. This offers some major advantages to the handful of people who’ve already started to use the Stentrode.
The audio improves about 10 minutes into the episode. (There was a minor headset issue early on, but everything is audible throughout.) Dr. Oxley’s work creates game-changing opportunities for patients desperate for new options. His take on where we're headed with BCIs is must listening for anyone who cares about the future of health and technology.
Listen on Apple | Listen on Spotify | Listen on Stitcher | Listen on Amazon | Listen on Google
In our conversation, Dr. Oxley talks about “Bluetooth brain”; the critical role of AI in the present and future of BCIs; how BCIs compare to voice command technology; regulatory frameworks for revolutionary technologies; specific people with paralysis who’ve been able to regain some independence thanks to the Stentrode; what it means to be a neurointerventionist; how to scale BCIs for more people to use them; the risks of BCIs malfunctioning; organic implants; and how BCIs help us understand the brain, among other topics.
Dr. Oxley received his PhD in neuro engineering from the University of Melbourne in Australia. He is the founding CEO of Synchron and an associate professor and the head of the vascular bionics laboratory at the University of Melbourne. He’s also a clinical instructor in the Deepartment of Neurosurgery at Mount Sinai Hospital. Dr. Oxley has completed more than 1,600 endovascular neurosurgical procedures on patients, including people with aneurysms and strokes, and has authored over 100 peer reviewed articles.
Links:
Synchron website - https://synchron.com/
Assessment of Safety of a Fully Implanted Endovascular Brain-Computer Interface for Severe Paralysis in 4 Patients (paper co-authored by Tom Oxley) - https://jamanetwork.com/journals/jamaneurology/art...
More research related to Synchron's work - https://synchron.com/research
Tom Oxley on LinkedIn - https://www.linkedin.com/in/tomoxl
Tom Oxley on Twitter - https://twitter.com/tomoxl?lang=en
Tom Oxley TED - https://www.ted.com/talks/tom_oxley_a_brain_implant_that_turns_your_thoughts_into_text?language=en
Tom Oxley website - https://tomoxl.com/
Novel brain implant helps paralyzed woman speak using digital avatar - https://engineering.berkeley.edu/news/2023/08/novel-brain-implant-helps-paralyzed-woman-speak-using-a-digital-avatar/
Edward Chang lab - https://changlab.ucsf.edu/
BCIs convert brain activity into text at 62 words per minute - https://med.stanford.edu/neurosurgery/news/2023/he...
Leaps.org: The Mind-Blowing Promise of Neural Implants - https://leaps.org/the-mind-blowing-promise-of-neural-implants/
Tom Oxley