The Death Predictor: A Helpful New Tool or an Ethical Morass?
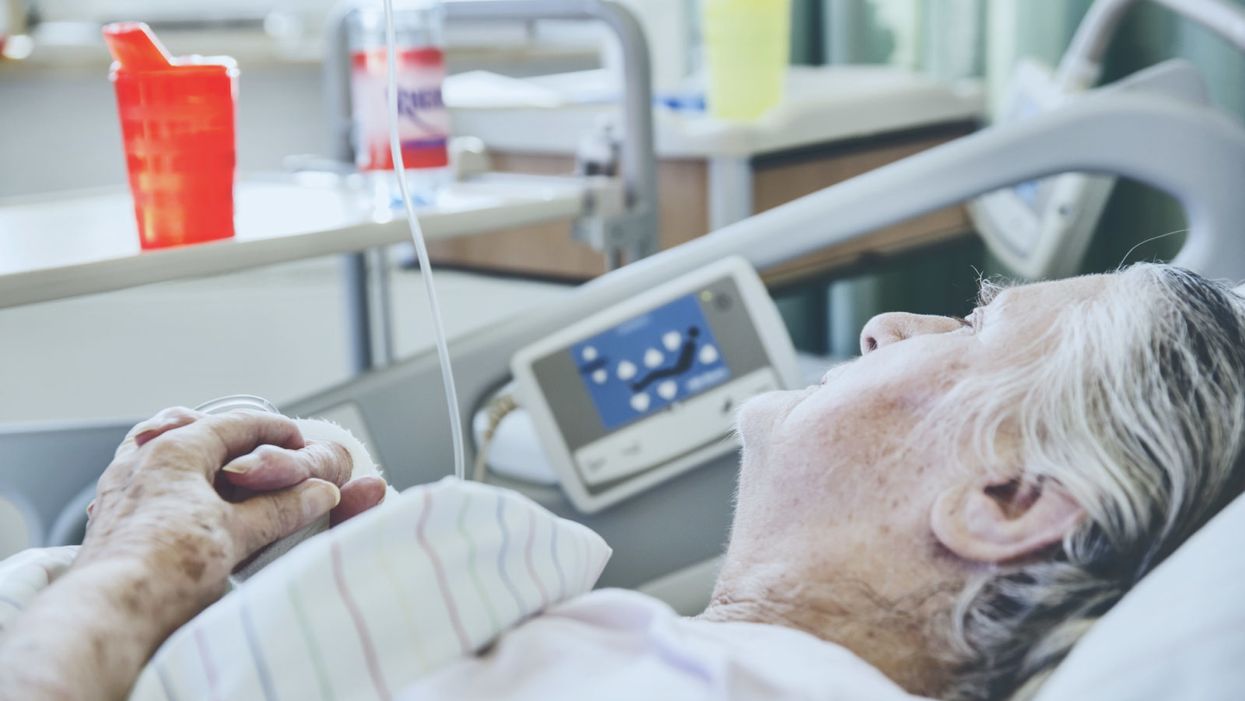
A senior in hospice care.
Whenever Eric Karl Oermann has to tell a patient about a terrible prognosis, their first question is always: "how long do I have?" Oermann would like to offer a precise answer, to provide some certainty and help guide treatment. But although he's one of the country's foremost experts in medical artificial intelligence, Oermann is still dependent on a computer algorithm that's often wrong.
Doctors are notoriously terrible at guessing how long their patients will live.
Artificial intelligence, now often called deep learning or neural networks, has radically transformed language and image processing. It's allowed computers to play chess better than the world's grand masters and outwit the best Jeopardy players. But it still can't precisely tell a doctor how long a patient has left – or how to help that person live longer.
Someday, researchers predict, computers will be able to watch a video of a patient to determine their health status. Doctors will no longer have to spend hours inputting data into medical records. And computers will do a better job than specialists at identifying tiny tumors, impending crises, and, yes, figuring out how long the patient has to live. Oermann, a neurosurgeon at Mount Sinai, says all that technology will allow doctors to spend more time doing what they do best: talking with their patients. "I want to see more deep learning and computers in a clinical setting," he says, "so there can be more human interaction." But those days are still at least three to five years off, Oermann and other researchers say.
Doctors are notoriously terrible at guessing how long their patients will live, says Nigam Shah, an associate professor at Stanford University and assistant director of the school's Center for Biomedical Informatics Research. Doctors don't want to believe that their patient – whom they've come to like – will die. "Doctors over-estimate survival many-fold," Shah says. "How do you go into work, in say, oncology, and not be delusionally optimistic? You have to be."
But patients near the end of life will get better treatment – and even live longer – if they are overseen by hospice or palliative care, research shows. So, instead of relying on human bias to select those whose lives are nearing their end, Shah and his colleagues showed that they could use a deep learning algorithm based on medical records to flag incoming patients with a life expectancy of three months to a year. They use that data to indicate who might need palliative care. Then, the palliative care team can reach out to treating physicians proactively, instead of relying on their referrals or taking the time to read extensive medical charts.
But, although the system works well, Shah isn't yet sure if such indicators actually get the appropriate patients into palliative care. He's recently partnered with a palliative care doctor to run a gold-standard clinical trial to test whether patients who are flagged by this algorithm are indeed a better match for palliative care.
"What is effective from a health system perspective might not be effective from a treating physician's perspective and might not be effective from the patient's perspective," Shah notes. "I don't have a good way to guess everybody's reaction without actually studying it." Whether palliative care is appropriate, for instance, depends on more than just the patient's health status. "If the patient's not ready, the family's not ready and the doctor's not ready, then you're just banging your head against the wall," Shah says. "Given limited capacity, it's a waste of resources" to put that person in palliative care.
The algorithm isn't perfect, but "on balance, it leads to better decisions more often."
Alexander Smith and Sei Lee, both palliative care doctors, work together at the University of California, San Francisco, to develop predictions for patients who come to the hospital with a complicated prognosis or a history of decline. Their algorithm, they say, helps decide if this patient's problems – which might include diabetes, heart disease, a slow-growing cancer, and memory issues – make them eligible for hospice. The algorithm isn't perfect, they both agree, but "on balance, it leads to better decisions more often," Smith says.
Bethany Percha, an assistant professor at Mount Sinai, says that an algorithm may tell doctors that their patient is trending downward, but it doesn't do anything to change that trajectory. "Even if you can predict something, what can you do about it?" Algorithms may be able to offer treatment suggestions – but not what specific actions will alter a patient's future, says Percha, also the chief technology officer of Precise Health Enterprise, a product development group within Mount Sinai. And the algorithms remain challenging to develop. Electronic medical records may be great at her hospital, but if the patient dies at a different one, her system won't know. If she wants to be certain a patient has died, she has to merge social security records of death with her system's medical records – a time-consuming and cumbersome process.
An algorithm that learns from biased data will be biased, Shah says. Patients who are poor or African American historically have had worse health outcomes. If researchers train an algorithm on data that includes those biases, they get baked into the algorithms, which can then lead to a self-fulfilling prophesy. Smith and Lee say they've taken race out of their algorithms to avoid this bias.
Age is even trickier. There's no question that someone's risk of illness and death goes up with age. But an 85-year-old who breaks a hip running a marathon should probably be treated very differently than an 85-year-old who breaks a hip trying to get out of a chair in a dementia care unit. That's why the doctor can never be taken out of the equation, Shah says. Human judgment will always be required in medical care and an algorithm should never be followed blindly, he says.
Experts say that the flaws in artificial intelligence algorithms shouldn't prevent people from using them – carefully.
Researchers are also concerned that their algorithms will be used to ration care, or that insurance companies will use their data to justify a rate increase. If an algorithm predicts a patient is going to end up back in the hospital soon, "who's benefitting from knowing a patient is going to be readmitted? Probably the insurance company," Percha says.
Still, Percha and others say, the flaws in artificial intelligence algorithms shouldn't prevent people from using them – carefully. "These are new and exciting tools that have a lot of potential uses. We need to be conscious about how to use them going forward, but it doesn't mean we shouldn't go down this road," she says. "I think the potential benefits outweigh the risks, especially because we've barely scratched the surface of what big data can do right now."
This man spent over 70 years in an iron lung. What he was able to accomplish is amazing.
Paul Alexander spent more than 70 years confined to an iron lung after a polio infection left him paralyzed at age 6. Here, Alexander uses a mirror attached to the top of his iron lung to view his surroundings.
It’s a sight we don’t normally see these days: A man lying prone in a big, metal tube with his head sticking out of one end. But it wasn’t so long ago that this sight was unfortunately much more common.
In the first half of the 20th century, tens of thousands of people each year were infected by polio—a highly contagious virus that attacks nerves in the spinal cord and brainstem. Many people survived polio, but a small percentage of people who did were left permanently paralyzed from the virus, requiring support to help them breathe. This support, known as an “iron lung,” manually pulled oxygen in and out of a person’s lungs by changing the pressure inside the machine.
Paul Alexander was one of several thousand who were infected and paralyzed by polio in 1952. That year, a polio epidemic swept the United States, forcing businesses to close and polio wards in hospitals all over the country to fill up with sick children. When Paul caught polio in the summer of 1952, doctors urged his parents to let him rest and recover at home, since the hospital in his home suburb of Dallas, Texas was already overrun with polio patients.
Paul rested in bed for a few days with aching limbs and a fever. But his condition quickly got worse. Within a week, Paul could no longer speak or swallow, and his parents rushed him to the local hospital where the doctors performed an emergency procedure to help him breathe. Paul woke from the surgery three days later, and found himself unable to move and lying inside an iron lung in the polio ward, surrounded by rows of other paralyzed children.
Hospitals were commonly filled with polio patients who had been paralyzed by the virus before a vaccine became widely available in 1955. Associated Press
But against all odds, Paul lived. And with help from a physical therapist, Paul was able to thrive—sometimes for small periods outside the iron lung.
The way Paul did this was to practice glossopharyngeal breathing (or as Paul called it, “frog breathing”), where he would trap air in his mouth and force it down his throat and into his lungs by flattening his tongue. This breathing technique, taught to him by his physical therapist, would allow Paul to leave the iron lung for increasing periods of time.
With help from his iron lung (and for small periods of time without it), Paul managed to live a full, happy, and sometimes record-breaking life. At 21, Paul became the first person in Dallas, Texas to graduate high school without attending class in person, owing his success to memorization rather than taking notes. After high school, Paul received a scholarship to Southern Methodist University and pursued his dream of becoming a trial lawyer and successfully represented clients in court.
Paul Alexander, pictured here in his early 20s, mastered a type of breathing technique that allowed him to spend short amounts of time outside his iron lung. Paul Alexander
Throughout his long life, Paul was also able to fly on a plane, visit the beach, adopt a dog, fall in love, and write a memoir using a plastic stick to tap out a draft on a keyboard. In recent years, Paul joined TikTok and became a viral sensation with more than 330,000 followers. In one of his first videos, Paul advocated for vaccination and warned against another polio epidemic.
Paul was reportedly hospitalized with COVID-19 at the end of February and died on March 11th, 2024. He currently holds the Guiness World Record for longest survival inside an iron lung—71 years.
Polio thankfully no longer circulates in the United States, or in most of the world, thanks to vaccines. But Paul continues to serve as a reminder of the importance of vaccination—and the power of the human spirit.
““I’ve got some big dreams. I’m not going to accept from anybody their limitations,” he said in a 2022 interview with CNN. “My life is incredible.”
When doctors couldn’t stop her daughter’s seizures, this mom earned a PhD and found a treatment herself.
Savannah Salazar (left) and her mother, Tracy Dixon-Salazaar, who earned a PhD in neurobiology in the quest for a treatment of her daughter's seizure disorder.
Twenty-eight years ago, Tracy Dixon-Salazaar woke to the sound of her daughter, two-year-old Savannah, in the midst of a medical emergency.
“I entered [Savannah’s room] to see her tiny little body jerking about violently in her bed,” Tracy said in an interview. “I thought she was choking.” When she and her husband frantically called 911, the paramedic told them it was likely that Savannah had had a seizure—a term neither Tracy nor her husband had ever heard before.
Over the next several years, Savannah’s seizures continued and worsened. By age five Savannah was having seizures dozens of times each day, and her parents noticed significant developmental delays. Savannah was unable to use the restroom and functioned more like a toddler than a five-year-old.
Doctors were mystified: Tracy and her husband had no family history of seizures, and there was no event—such as an injury or infection—that could have caused them. Doctors were also confused as to why Savannah’s seizures were happening so frequently despite trying different seizure medications.
Doctors eventually diagnosed Savannah with Lennox-Gaustaut Syndrome, or LGS, an epilepsy disorder with no cure and a poor prognosis. People with LGS are often resistant to several kinds of anti-seizure medications, and often suffer from developmental delays and behavioral problems. People with LGS also have a higher chance of injury as well as a higher chance of sudden unexpected death (SUDEP) due to the frequent seizures. In about 70 percent of cases, LGS has an identifiable cause such as a brain injury or genetic syndrome. In about 30 percent of cases, however, the cause is unknown.
Watching her daughter struggle through repeated seizures was devastating to Tracy and the rest of the family.
“This disease, it comes into your life. It’s uninvited. It’s unannounced and it takes over every aspect of your daily life,” said Tracy in an interview with Today.com. “Plus it’s attacking the thing that is most precious to you—your kid.”
Desperate to find some answers, Tracy began combing the medical literature for information about epilepsy and LGS. She enrolled in college courses to better understand the papers she was reading.
“Ironically, I thought I needed to go to college to take English classes to understand these papers—but soon learned it wasn’t English classes I needed, It was science,” Tracy said. When she took her first college science course, Tracy says, she “fell in love with the subject.”
Tracy was now a caregiver to Savannah, who continued to have hundreds of seizures a month, as well as a full-time student, studying late into the night and while her kids were at school, using classwork as “an outlet for the pain.”
“I couldn’t help my daughter,” Tracy said. “Studying was something I could do.”
Twelve years later, Tracy had earned a PhD in neurobiology.
After her post-doctoral training, Tracy started working at a lab that explored the genetics of epilepsy. Savannah’s doctors hadn’t found a genetic cause for her seizures, so Tracy decided to sequence her genome again to check for other abnormalities—and what she found was life-changing.
Tracy discovered that Savannah had a calcium channel mutation, meaning that too much calcium was passing through Savannah’s neural pathways, leading to seizures. The information made sense to Tracy: Anti-seizure medications often leech calcium from a person’s bones. When doctors had prescribed Savannah calcium supplements in the past to counteract these effects, her seizures had gotten worse every time she took the medication. Tracy took her discovery to Savannah’s doctor, who agreed to prescribe her a calcium blocker.
The change in Savannah was almost immediate.
Within two weeks, Savannah’s seizures had decreased by 95 percent. Once on a daily seven-drug regimen, she was soon weaned to just four, and then three. Amazingly, Tracy started to notice changes in Savannah’s personality and development, too.
“She just exploded in her personality and her talking and her walking and her potty training and oh my gosh she is just so sassy,” Tracy said in an interview.
Since starting the calcium blocker eleven years ago, Savannah has continued to make enormous strides. Though still unable to read or write, Savannah enjoys puzzles and social media. She’s “obsessed” with boys, says Tracy. And while Tracy suspects she’ll never be able to live independently, she and her daughter can now share more “normal” moments—something she never anticipated at the start of Savannah’s journey with LGS. While preparing for an event, Savannah helped Tracy get ready.
“We picked out a dress and it was the first time in our lives that we did something normal as a mother and a daughter,” she said. “It was pretty cool.”