The Death Predictor: A Helpful New Tool or an Ethical Morass?
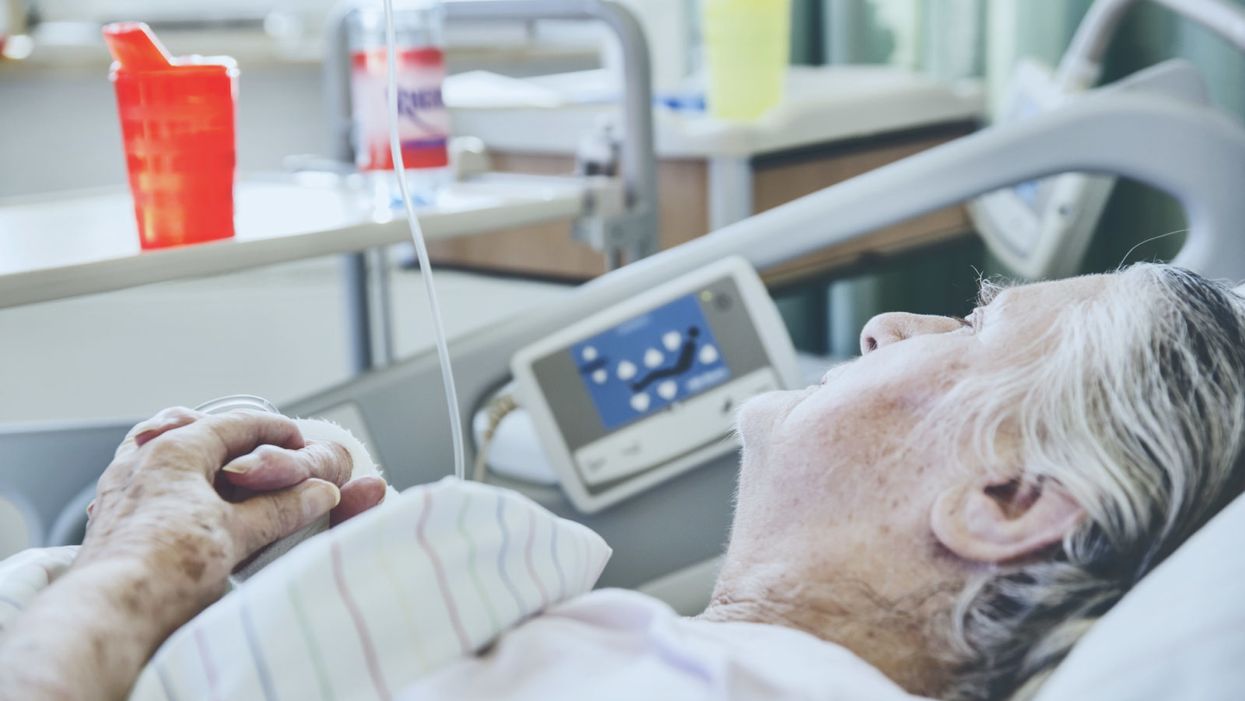
A senior in hospice care.
Whenever Eric Karl Oermann has to tell a patient about a terrible prognosis, their first question is always: "how long do I have?" Oermann would like to offer a precise answer, to provide some certainty and help guide treatment. But although he's one of the country's foremost experts in medical artificial intelligence, Oermann is still dependent on a computer algorithm that's often wrong.
Doctors are notoriously terrible at guessing how long their patients will live.
Artificial intelligence, now often called deep learning or neural networks, has radically transformed language and image processing. It's allowed computers to play chess better than the world's grand masters and outwit the best Jeopardy players. But it still can't precisely tell a doctor how long a patient has left – or how to help that person live longer.
Someday, researchers predict, computers will be able to watch a video of a patient to determine their health status. Doctors will no longer have to spend hours inputting data into medical records. And computers will do a better job than specialists at identifying tiny tumors, impending crises, and, yes, figuring out how long the patient has to live. Oermann, a neurosurgeon at Mount Sinai, says all that technology will allow doctors to spend more time doing what they do best: talking with their patients. "I want to see more deep learning and computers in a clinical setting," he says, "so there can be more human interaction." But those days are still at least three to five years off, Oermann and other researchers say.
Doctors are notoriously terrible at guessing how long their patients will live, says Nigam Shah, an associate professor at Stanford University and assistant director of the school's Center for Biomedical Informatics Research. Doctors don't want to believe that their patient – whom they've come to like – will die. "Doctors over-estimate survival many-fold," Shah says. "How do you go into work, in say, oncology, and not be delusionally optimistic? You have to be."
But patients near the end of life will get better treatment – and even live longer – if they are overseen by hospice or palliative care, research shows. So, instead of relying on human bias to select those whose lives are nearing their end, Shah and his colleagues showed that they could use a deep learning algorithm based on medical records to flag incoming patients with a life expectancy of three months to a year. They use that data to indicate who might need palliative care. Then, the palliative care team can reach out to treating physicians proactively, instead of relying on their referrals or taking the time to read extensive medical charts.
But, although the system works well, Shah isn't yet sure if such indicators actually get the appropriate patients into palliative care. He's recently partnered with a palliative care doctor to run a gold-standard clinical trial to test whether patients who are flagged by this algorithm are indeed a better match for palliative care.
"What is effective from a health system perspective might not be effective from a treating physician's perspective and might not be effective from the patient's perspective," Shah notes. "I don't have a good way to guess everybody's reaction without actually studying it." Whether palliative care is appropriate, for instance, depends on more than just the patient's health status. "If the patient's not ready, the family's not ready and the doctor's not ready, then you're just banging your head against the wall," Shah says. "Given limited capacity, it's a waste of resources" to put that person in palliative care.
The algorithm isn't perfect, but "on balance, it leads to better decisions more often."
Alexander Smith and Sei Lee, both palliative care doctors, work together at the University of California, San Francisco, to develop predictions for patients who come to the hospital with a complicated prognosis or a history of decline. Their algorithm, they say, helps decide if this patient's problems – which might include diabetes, heart disease, a slow-growing cancer, and memory issues – make them eligible for hospice. The algorithm isn't perfect, they both agree, but "on balance, it leads to better decisions more often," Smith says.
Bethany Percha, an assistant professor at Mount Sinai, says that an algorithm may tell doctors that their patient is trending downward, but it doesn't do anything to change that trajectory. "Even if you can predict something, what can you do about it?" Algorithms may be able to offer treatment suggestions – but not what specific actions will alter a patient's future, says Percha, also the chief technology officer of Precise Health Enterprise, a product development group within Mount Sinai. And the algorithms remain challenging to develop. Electronic medical records may be great at her hospital, but if the patient dies at a different one, her system won't know. If she wants to be certain a patient has died, she has to merge social security records of death with her system's medical records – a time-consuming and cumbersome process.
An algorithm that learns from biased data will be biased, Shah says. Patients who are poor or African American historically have had worse health outcomes. If researchers train an algorithm on data that includes those biases, they get baked into the algorithms, which can then lead to a self-fulfilling prophesy. Smith and Lee say they've taken race out of their algorithms to avoid this bias.
Age is even trickier. There's no question that someone's risk of illness and death goes up with age. But an 85-year-old who breaks a hip running a marathon should probably be treated very differently than an 85-year-old who breaks a hip trying to get out of a chair in a dementia care unit. That's why the doctor can never be taken out of the equation, Shah says. Human judgment will always be required in medical care and an algorithm should never be followed blindly, he says.
Experts say that the flaws in artificial intelligence algorithms shouldn't prevent people from using them – carefully.
Researchers are also concerned that their algorithms will be used to ration care, or that insurance companies will use their data to justify a rate increase. If an algorithm predicts a patient is going to end up back in the hospital soon, "who's benefitting from knowing a patient is going to be readmitted? Probably the insurance company," Percha says.
Still, Percha and others say, the flaws in artificial intelligence algorithms shouldn't prevent people from using them – carefully. "These are new and exciting tools that have a lot of potential uses. We need to be conscious about how to use them going forward, but it doesn't mean we shouldn't go down this road," she says. "I think the potential benefits outweigh the risks, especially because we've barely scratched the surface of what big data can do right now."
A robot server, controlled remotely by a disabled worker, delivers drinks to patrons at the DAWN cafe in Tokyo.
A sleek, four-foot tall white robot glides across a cafe storefront in Tokyo’s Nihonbashi district, holding a two-tiered serving tray full of tea sandwiches and pastries. The cafe’s patrons smile and say thanks as they take the tray—but it’s not the robot they’re thanking. Instead, the patrons are talking to the person controlling the robot—a restaurant employee who operates the avatar from the comfort of their home.
It’s a typical scene at DAWN, short for Diverse Avatar Working Network—a cafe that launched in Tokyo six years ago as an experimental pop-up and quickly became an overnight success. Today, the cafe is a permanent fixture in Nihonbashi, staffing roughly 60 remote workers who control the robots remotely and communicate to customers via a built-in microphone.
More than just a creative idea, however, DAWN is being hailed as a life-changing opportunity. The workers who control the robots remotely (known as “pilots”) all have disabilities that limit their ability to move around freely and travel outside their homes. Worldwide, an estimated 16 percent of the global population lives with a significant disability—and according to the World Health Organization, these disabilities give rise to other problems, such as exclusion from education, unemployment, and poverty.
These are all problems that Kentaro Yoshifuji, founder and CEO of Ory Laboratory, which supplies the robot servers at DAWN, is looking to correct. Yoshifuji, who was bedridden for several years in high school due to an undisclosed health problem, launched the company to help enable people who are house-bound or bedridden to more fully participate in society, as well as end the loneliness, isolation, and feelings of worthlessness that can sometimes go hand-in-hand with being disabled.
“It’s heartbreaking to think that [people with disabilities] feel they are a burden to society, or that they fear their families suffer by caring for them,” said Yoshifuji in an interview in 2020. “We are dedicating ourselves to providing workable, technology-based solutions. That is our purpose.”
Shota Kuwahara, a DAWN employee with muscular dystrophy. Ory Labs, Inc.
Shota, Kuwahara, a DAWN employee with muscular dystrophy, agrees. "There are many difficulties in my daily life, but I believe my life has a purpose and is not being wasted," he says. "Being useful, able to help other people, even feeling needed by others, is so motivational."
A woman receives a mammogram, which can detect the presence of tumors in a patient's breast.
When a patient is diagnosed with early-stage breast cancer, having surgery to remove the tumor is considered the standard of care. But what happens when a patient can’t have surgery?
Whether it’s due to high blood pressure, advanced age, heart issues, or other reasons, some breast cancer patients don’t qualify for a lumpectomy—one of the most common treatment options for early-stage breast cancer. A lumpectomy surgically removes the tumor while keeping the patient’s breast intact, while a mastectomy removes the entire breast and nearby lymph nodes.
Fortunately, a new technique called cryoablation is now available for breast cancer patients who either aren’t candidates for surgery or don’t feel comfortable undergoing a surgical procedure. With cryoablation, doctors use an ultrasound or CT scan to locate any tumors inside the patient’s breast. They then insert small, needle-like probes into the patient's breast which create an “ice ball” that surrounds the tumor and kills the cancer cells.
Cryoablation has been used for decades to treat cancers of the kidneys and liver—but only in the past few years have doctors been able to use the procedure to treat breast cancer patients. And while clinical trials have shown that cryoablation works for tumors smaller than 1.5 centimeters, a recent clinical trial at Memorial Sloan Kettering Cancer Center in New York has shown that it can work for larger tumors, too.
In this study, doctors performed cryoablation on patients whose tumors were, on average, 2.5 centimeters. The cryoablation procedure lasted for about 30 minutes, and patients were able to go home on the same day following treatment. Doctors then followed up with the patients after 16 months. In the follow-up, doctors found the recurrence rate for tumors after using cryoablation was only 10 percent.
For patients who don’t qualify for surgery, radiation and hormonal therapy is typically used to treat tumors. However, said Yolanda Brice, M.D., an interventional radiologist at Memorial Sloan Kettering Cancer Center, “when treated with only radiation and hormonal therapy, the tumors will eventually return.” Cryotherapy, Brice said, could be a more effective way to treat cancer for patients who can’t have surgery.
“The fact that we only saw a 10 percent recurrence rate in our study is incredibly promising,” she said.