Your Digital Avatar May One Day Get Sick Before You Do
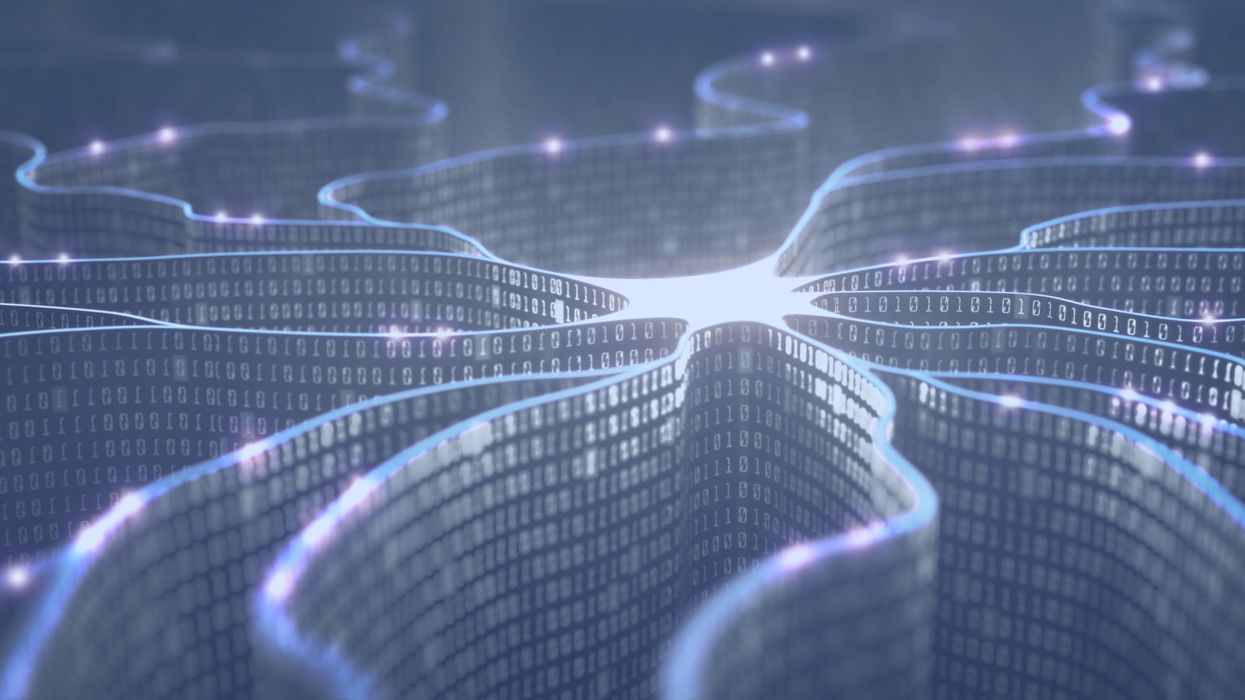
Artificial neurons in a concept of artificial intelligence.
Artificial intelligence is everywhere, just not in the way you think it is.
These networks, loosely designed after the human brain, are interconnected computers that have the ability to "learn."
"There's the perception of AI in the glossy magazines," says Anders Kofod-Petersen, a professor of Artificial Intelligence at the Norwegian University of Science and Technology. "That's the sci-fi version. It resembles the small guy in the movie AI. It might be benevolent or it might be evil, but it's generally intelligent and conscious."
"And this is, of course, as far from the truth as you can possibly get."
What Exactly Is Artificial Intelligence, Anyway?
Let's start with how you got to this piece. You likely came to it through social media. Your Facebook account, Twitter feed, or perhaps a Google search. AI influences all of those things, machine learning helping to run the algorithms that decide what you see, when, and where. AI isn't the little humanoid figure; it's the system that controls the figure.
"AI is being confused with robotics," Eleonore Pauwels, Director of the Anticipatory Intelligence Lab with the Science and Technology Innovation Program at the Wilson Center, says. "What AI is right now is a data optimization system, a very powerful data optimization system."
The revolution in recent years hasn't come from the method scientists and other researchers use. The general ideas and philosophies have been around since the late 1960s. Instead, the big change has been the dramatic increase in computing power, primarily due to the development of neural networks. These networks, loosely designed after the human brain, are interconnected computers that have the ability to "learn." An AI, for example, can be taught to spot a picture of a cat by looking at hundreds of thousands of pictures that have been labeled "cat" and "learning" what a cat looks like. Or an AI can beat a human at Go, an achievement that just five years ago Kofod-Petersen thought wouldn't be accomplished for decades.
"It's very difficult to argue that something is intelligent if it can't learn, and these algorithms are getting pretty good at learning stuff. What they are not good at is learning how to learn."
Medicine is the field where this expertise in perception tasks might have the most influence. It's already having an impact as iPhones use AI to detect cancer, Apple watches alert the wearer to a heart problem, AI spots tuberculosis and the spread of breast cancer with a higher accuracy than human doctors, and more. Every few months, another study demonstrates more possibility. (The New Yorker published an article about medicine and AI last year, so you know it's a serious topic.)
But this is only the beginning. "I personally think genomics and precision medicine is where AI is going to be the biggest game-changer," Pauwels says. "It's going to completely change how we think about health, our genomes, and how we think about our relationship between our genotype and phenotype."
The Fundamental Breakthrough That Must Be Solved
To get there, however, researchers will need to make another breakthrough, and there's debate about how long that will take. Kofod-Petersen explains: "If we want to move from this narrow intelligence to this broader intelligence, that's a very difficult problem. It basically boils down to that we haven't got a clue about what intelligence actually is. We don't know what intelligence means in a biological sense. We think we might recognize it but we're not completely sure. There isn't a working definition. We kind of agree with the biologists that learning is an aspect of it. It's very difficult to argue that something is intelligent if it can't learn, and these algorithms are getting pretty good at learning stuff. What they are not good at is learning how to learn. They can learn specific tasks but we haven't approached how to teach them to learn to learn."
In other words, current AI is very, very good at identifying that a picture of a cat is, in fact, a cat – and getting better at doing so at an incredibly rapid pace – but the system only knows what a "cat" is because that's what a programmer told it a furry thing with whiskers and two pointy ears is called. If the programmer instead decided to label the training images as "dogs," the AI wouldn't say "no, that's a cat." Instead, it would simply call a furry thing with whiskers and two pointy ears a dog. AI systems lack the explicit inference that humans do effortlessly, almost without thinking.
Pauwels believes that the next step is for AI to transition from supervised to unsupervised learning. The latter means that the AI isn't answering questions that a programmer asks it ("Is this a cat?"). Instead, it's almost like it's looking at the data it has, coming up with its own questions and hypothesis, and answering them or putting them to the test. Combining this ability with the frankly insane processing power of the computer system could result in game-changing discoveries.
In the not-too-distant future, a doctor could run diagnostics on a digital avatar, watching which medical conditions present themselves before the person gets sick in real life.
One company in China plans to develop a way to create a digital avatar of an individual person, then simulate that person's health and medical information into the future. In the not-too-distant future, a doctor could run diagnostics on a digital avatar, watching which medical conditions presented themselves – cancer or a heart condition or anything, really – and help the real-life version prevent those conditions from beginning or treating them before they became a life-threatening issue.
That, obviously, would be an incredibly powerful technology, and it's just one of the many possibilities that unsupervised AI presents. It's also terrifying in the potential for misuse. Even the term "unsupervised AI" brings to mind a dystopian landscape where AI takes over and enslaves humanity. (Pick your favorite movie. There are dozens.) This is a concern, something for developers, programmers, and scientists to consider as they build the systems of the future.
The Ethical Problem That Deserves More Attention
But the more immediate concern about AI is much more mundane. We think of AI as an unbiased system. That's incorrect. Algorithms, after all, are designed by someone or a team, and those people have explicit or implicit biases. Intentionally, or more likely not, they introduce these biases into the very code that forms the basis for the AI. Current systems have a bias against people of color. Facebook tried to rectify the situation and failed. These are two small examples of a larger, potentially systemic problem.
It's vital and necessary for the people developing AI today to be aware of these issues. And, yes, avoid sending us to the brink of a James Cameron movie. But AI is too powerful a tool to ignore. Today, it's identifying cats and on the verge of detecting cancer. In not too many tomorrows, it will be on the forefront of medical innovation. If we are careful, aware, and smart, it will help simulate results, create designer drugs, and revolutionize individualize medicine. "AI is the only way to get there," Pauwels says.
Jurassic Park Without the Scary Parts: How Stem Cells May Rescue the Near-Extinct Rhinoceros
The Northern white rhinoceros Nola, the last one in the U.S. at that time in 2015, pictured here with author Jeanne Loring and Oliver Ryder (in truck), with a film crew and keepers in the San Diego Zoo's savanna. Nola sadly passed away that year.
I am a stem cell scientist. In my day job I work on developing ways to use stem cells to treat neurological disease – human disease. This is the story about how I became part of a group dedicated to rescuing the northern white rhinoceros from extinction.
The earth is now in an era that is called the "sixth mass extinction." The first extinction, 400 million years ago, put an end to 86 percent of the existing species, including most of the trilobites. When the earth grew hotter, dustier, or darker, it lost fish, amphibians, reptiles, plants, dinosaurs, mammals and birds. Each extinction event wiped out 80 to 90 percent of the life on the planet at the time. The first 5 mass extinctions were caused by natural disasters: volcanoes, fires, a meteor. But humans can take credit for the 6th.
Because of human activities that destroy habitats, creatures are now becoming extinct at a rate that is higher than any previously experienced. Some animals, like the giant panda and the California condor, have been pulled back from the brink of extinction by conserving their habitats, breeding in captivity, and educating the public about their plight.
But not the northern white rhino. This gentle giant is a vegetarian that can weigh up to 5,000 pounds. The rhino's weakness is its horn, which has become a valuable commodity because of the mistaken idea that it grants power and has medicinal value. Horns are not medicine; the horns are made of keratin, the same protein that is in fingernails. But as recently as 2017 more than 1,000 rhinos were slaughtered each year to harvest their horns.
All 6 rhino species are endangered. But the northern white has been devastated. Only two members of this species are alive now: Najin, age 32, and her daughter Fatu, 21, live in a protected park in Kenya. They are social animals and would prefer the company of other rhinos of their kind; but they can't know that they are the last two survivors of their entire species. No males exist anymore. The last male, Sudan, died in 2018 at age 45.
We are celebrating a huge milestone in the efforts to use stem cells to rescue the rhino.
I became involved in the rhino rescue project on a sunny day in February, 2008 at the San Diego Wild Animal Park in Escondido, about 30 miles north of my lab in La Jolla. My lab had relocated a couple of months earlier to Scripps Research Institute to start the Center for Regenerative Medicine for human stem cell research. To thank my staff for their hard work, I wanted to arrange a special treat. I contacted my friend Oliver Ryder, who is director of the Institute for Conservation Research at the zoo, to see if I could take them on a safari, a tour in a truck through the savanna habitat at the park.
This was the first of the "stem cell safaris" that the lab would enjoy over the next few years. On the safari we saw elands and cape buffalo, and fed giraffes and rhinos. And we talked about stem cells; in particular, we discussed a surprising technological breakthrough recently reported by the Japanese scientist Shinya Yamanaka that enabled conversion of ordinary skin cells into pluripotent stem cells.
Pluripotent stem cells can develop into virtually any cell type in the body. They exist when we are very young embryos; five days after we were just fertilized eggs, we became blastocysts, invisible tiny balls of a few hundred cells packed with the power to develop into an entire human being. Long before we are born, these cells of vast potential transform into highly specialized cells that generate our brains, our hearts, and everything else.
Human pluripotent stem cells from blastocysts can be cultured in the lab, and are called embryonic stem cells. But thanks to Dr. Yamanaka, anyone can have their skin cells reprogrammed into pluripotent stem cells, just like the ones we had when we were embryos. Dr. Yamanaka won the Nobel Prize for these cells, called "induced pluripotent stem cells" (iPSCs) several years later.
On our safari we realized that if we could make these reprogrammed stem cells from human skin cells, why couldn't we make them from animals' cells? How about endangered animals? Could such stem cells be made from animals whose skin cells had been being preserved since the 1970s in the San Diego Zoo's Frozen Zoo®? Our safari leader, Oliver Ryder, was the curator of the Frozen Zoo and knew what animal cells were stored in its giant liquid nitrogen tanks at −196°C (-320° F). The Frozen Zoo was established by Dr. Kurt Benirschke in 1975 in the hope that someday the collection would aid in rescue of animals that were on the brink of extinction. The frozen collection reached 10,000 cell lines this year.
We returned to the lab after the safari, and I asked my scientists if any of them would like to take on the challenge of making reprogrammed stem cells from endangered species. My new postdoctoral fellow, Inbar Friedrich Ben-Nun, raised her hand. Inbar had arrived only a few weeks earlier from Israel, and she was excited about doing something that had never been done before. Oliver picked the animals we would use. He chose his favorite animal, the critically endangered northern white rhinoceros, and the drill, which is an endangered primate related to the mandrill monkey,
When Inbar started work on reprogramming cells from the Frozen Zoo, there were 8 living northern rhinoceros around the world: Nola, Angalifu, Nesari, Nabire, Suni, Sudan, Najin, and Fatu. We chose to reprogram Fatu, the youngest of the remaining animals.
Through sheer determination and trial and error, Inbar got the reprogramming technique to work, and in 2011 we published the first report of iPSCs from endangered species in the scientific journal Nature Methods. The cover of the journal featured a drawing of an ark packed with animals that might someday be rescued through iPSC technology. By 2011, one of the 8 rhinos, Nesari, had died.
This kernel of hope for using iPSCs to rescue rhinos grew over the next 10 years. The zoo built the Rhino Rescue Center, and brought in 6 females of the closely related species, the southern white rhinoceros, from Africa. Southern white rhino populations are on the rise, and it appears that this species will survive, at least in captivity. The females are destined to be surrogate mothers for embryos made from northern white rhino cells, when eventually we hope to generate sperm and eggs from the reprogrammed stem cells, and fertilize the eggs in vitro, much the same as human IVF.
The author, Jeanne Loring, at the Rhino Rescue Center with one of the southern white rhino surrogates.
David Barker
As this project has progressed, we've been saddened by the loss of all but the last two remaining members of the species. Nola, the last northern white rhino in the U.S., who was at the San Diego Zoo, died in 2015.
But we are celebrating a huge milestone in the efforts to use stem cells to rescue the rhino. Just over a month ago, we reported that by reprogramming cells preserved in the Frozen Zoo, we produced iPSCs from stored cells of 9 northern white rhinos: Fatu, Najin, Nola, Suni, Nadi, Dinka, Nasima, Saut, and Angalifu. We also reprogrammed cells from two of the southern white females, Amani and Wallis.
We don't know when it will be possible to make a northern white rhino embryo; we have to figure out how to use methods already developed for laboratory mice to generate sperm and eggs from these cells. The male rhino Angalifu died in 2014, but ever since I saw beating heart cells derived from his very own cells in a culture dish, I've felt hope that he will one day have children who will seed a thriving new herd of northern white rhinos.
A leading virologist reflects on the past year and the unknowns about COVID-19 that we still need to solve.
On the one-year anniversary of the World Health Organization declaring SARS-CoV-2 a global pandemic, it's hard to believe that so much and yet so little time has passed. The past twelve months seem to have dragged by, with each day feeling like an eternity, yet also it feels as though it has flashed by in a blur.
Nearly everyone I've spoken with, from recent acquaintances to my closest friends and family, have reported feeling the same odd sense of disconnectedness, which I've taken to calling "pandemic relativity." Just this week, Ellen Cushing published a piece in The Atlantic about the effects of "late-stage pandemic" on memory and cognitive function. Perhaps, thanks to twelve months of living this way, we have all found it that much more difficult to distill the key lessons that will allow us to emerge from the relentless, disconnected grind of our current reality, return to some semblance of normalcy, and take the decisive steps needed to ensure the mistakes of this pandemic are not repeated in the next one.
As a virologist who studies SARS-CoV-2 and other emerging viruses, and who sometimes writes and publicly comments on my thoughts, I've been asked frequently about what we've learned as we approach a year of living in suspension. While I always come up with an answer, the truth is similar to my perception of time: we've learned a lot, but at the same time, that's only served to highlight how much we still don't know. We have uncovered and clarified many scientific truths, but also revealed the limits of our scientific knowledge.
The Most Important Lessons Learned
The dangers of false dichotomies.
From the early days, we have been guilty of binary thinking, and this has touched nearly every aspect of the pandemic. The following statements are not true, but the narratives are all too common: The only outcomes of COVID-19 are full recovery or death. Masks either work perfectly or they don't work at all. Transmission only occurs entirely by droplets or is entirely airborne. Children are either complete immune or they are equally as susceptible as adults. Vaccines either completely protect against infection and illness or they are completely useless. Any true student of biology can tell you that there are very rarely binary certainties that apply to every situation, but sensible public health advice can be rapidly derailed by discussing biological realities that exist on a continuum as if they are all categorically true or false.
It's a natural impulse to reduce complex systems to a choice of two options, and also to seek absolute certainty. A challenge for all scientists is how to communicate uncertainty when many people are understandably frustrated at this point and sick of hearing "we don't know." If we don't know now, when will we know? How much do we need to know to make good decisions? When will we get back to "normal"? In trying to simplify complex scientific concepts, we've made them hopelessly complicated. An important lesson going forward is that we should move away from black and white conversations about the emerging science and embrace the shades of gray, with all the nuance and uncertainty that entails.
Novel pandemic viruses can be controlled without a vaccine or effective antiviral therapeutics, and there is no one right way to do so.
Coronaviruses are very different from influenza.
Since the beginning of the pandemic, the superficial similarities between SARS-CoV-2 and influenza viruses have inevitably led to comparisons: both are primarily respiratory viruses with some symptoms in common, both have a relatively low overall mortality rate, both are zoonotic viruses that spilled over into the human population from animals, both are enveloped viruses that use RNA, rather than DNA, as their genetic material.
But these similarities disguise the fact that these are two fundamentally different pathogens. They have very different biology at virtually every step of the viral replication cycle, or the process that a virus goes through when it infects a cell and transforms it into a virus factory. SARS-CoV-2 enters cells by interacting with a protein on cell surfaces called ACE-2, while influenza viruses interact with a sugar molecule called sialic acid that "decorates" cell surface proteins. This means the viruses infect different types of cells in the respiratory tract and throughout the body. They also encode vastly different types of viral proteins meant to subvert and hijack the cells they infect: the genome of influenza virus is less than half the size of the genome of SARS-CoV-2, and encodes fewer than half as many viral proteins that can interact with the host cell.
As a result, these viruses each interact with host cells in unique ways and induce different responses to infection. The host response to infection is critically important for determining disease severity in both influenza and COVID-19, with the most severe disease associated with an uncontrolled inflammatory response that results in damaging the lungs and other affected tissues. Indeed, comparative studies have now shown that COVID-19 and influenza infection induce very different host response profiles in infected cells, leading to fundamentally different diseases. Our early reliance on pandemic response plans and public health strategies designed for influenza virus was a mistake, and this will be critical to preparedness and improved response plans going forward.
Transmission is situational.
Another way in which SARS-CoV-2 is very different from influenza is how it spreads through a population, which is relevant to how it is transmitted. Early on, many people focused on the fact that the basic reproduction number (R0) of SARS-CoV-2 was between 2 and 4, similar to the 1918 influenza pandemic. R0 describes the number of people that an infected person will transmit the virus to, but this is an average.
Another key measurement epidemiologists use to look at spread is dispersion, or patterns of transmission. If R0 is 2, and you have a population of 10 people, does that mean that all 10 people transmitted the virus to exactly 2 people? Or did 4 of the people each transmit to 5 people, with the other 6 of the 10 transmitting to nobody? In both situations, the average number of new infections is still 2, but the latter situation is described as overdispersion. While influenza is typically not very overdispersed, SARS-CoV-2 is heavily overdispersed. This is reflected in the high frequency of "superspreading events", where many people are infected at the same time.
Superspreading events are highly dependent on circumstances that need to align to create a conducive environment for transmission. SARS-CoV-2 is primarily transmitted by either inhalation of infectious aerosols (smaller respiratory particles suspended in the air) or direct contact with infectious droplets (larger respiratory particles that can be transferred from the body to the nose or mouth). This means that transmission is more likely to occur in situations with increased exposure risk. The risk is additive, with the likelihood of transmission being higher with more potential sources of virus (people from different households), higher respiratory particle emissions (lack of masks and/or shouting or singing), a physical environment that concentrates potentially infectious particles (an enclosed, poorly ventilated indoor space), close physical proximity (crowding), and increased exposure time.
We have seen repeatedly that when these conditions are met, such as in crowded bars or restaurants, gyms, cruise ships, buses, or weddings, superspreading can occur. The good news, however, is that identifying all these different risk factors has also allowed us to identify methods to mitigate transmission, and these are also additive: masks, physical distancing, avoiding enclosed spaces, limiting interactions with people outside your household, improving ventilation, and practicing good hand hygiene all reduce exposure risk.
Presymptomatic and asymptomatic transmission are critical to controlling a pandemic.
Another critical early mistake was assuming that SARS-CoV-2 would be transmitted only by symptomatic people. This was an understandable assumption to make, as people infected with "classic" SARS-CoV reliably developed fevers and could be identified based on body temperature and symptom screening. However, by March 2020, it was apparent that symptom-based screening was inadequate. The symptoms of COVID-19 fall along a very broad spectrum, ranging from completely asymptomatic infection to lethal pneumonia, with everything from loss of taste and smell to "COVID toes" to diarrhea to kidney failure to strokes in between.
Furthermore, last spring several studies showed that viral loads in the nose and throat were highest at the time of symptom onset, suggesting that people were likely to be contagious before they would be aware that they were sick. This created a tremendous challenge that repeatedly thwarted efforts to control community transmission in many countries, including the U.S. Without sufficient testing and surveillance, and with prevalence too high to enable robust contact tracing, efforts to identify and quarantine exposed people were unsuccessful. While the percentage of cases resulting from silent asymptomatic or presymptomatic transmission is still not precisely determined, it may account for nearly half of new infections and has been observed repeatedly. However, our policies have not caught up, and overeager reopening and blanket lifting of mask mandates often fail to account for contagious people who don't realize they are infected. Unfortunately, it's now also well-established that prematurely letting up on precautions can drive new surges in case numbers.
There's more than one way to stop a pandemic. While we've certainly seen examples of failed pandemic responses by looking at the U.S. and most of Western Europe, there have been a number of other countries that have very effectively controlled the pandemic within their borders. This hasn't been a one-size-fits-all approach, either. China infamously instituted a draconian lockdown in late January after the pandemic quickly spread from Wuhan to the rest of the country. A number of other countries, including Taiwan, Hong Kong, South Korea, Vietnam, Australia, New Zealand, and Japan, have implemented various combinations of policy measures (travel restrictions, lockdowns), epidemiological approaches (contact tracing, isolation and quarantine), data collection (testing capacity and surveillance), and mitigation measures (mask availability and mandates, exposure risk reduction education campaigns), that have effectively kept prevalence low and in some cases eliminated COVID-19 altogether. It shows that novel pandemic viruses can be controlled without a vaccine or effective antiviral therapeutics, and also that there is no one right way to do so.
We can develop safe, effective vaccines in record time.
Last March, Dr. Anthony S. Fauci estimated that a vaccine might be available in 12 to 18 months. At the time this was thought to be an extremely optimistic estimate, given that vaccines typically take years to design, develop, and test to ensure they are safe and effective. So how did we go from the drawing board to authorized vaccines, which so far appear to be very safe and effective, in less than a year? In part this is due to streamlining the clinical trial process, allowing previously sequential steps in the pipeline to occur simultaneously, such as phase 3 clinical trials and manufacturing.
The expedited trial process also built upon previous studies with the vaccine technologies, including extensive preclinical studies and clinical trials that tested mRNA (Pfizer/BioNTech and Moderna) and adenovirus-vectored (Johnson and Johnson and AstraZeneca) vaccines against other viruses, including MERS-CoV, a cousin of SARS-CoV-2. Prior to the phase 3 clinical trials "reading out" (amassing enough data to enable a statistically robust appraisal of their safety and efficacy), our expectations were modest, hoping for 50 to 60% protection against COVID-19. Thus far, all the vaccines that have completed phase 3 trials have exceeded that expectation. While future vaccines will likely still take years to fully evaluate, we can apply the achievements of the SARS-CoV-2 vaccines to make the regulatory process more efficient for other vaccines, as well as develop ways to further expedite the process in emergencies without compromising safety or effectiveness. A more efficient regulatory environment could improve access to other technologies, such as promising new tests and therapeutics, as well.
The Biggest Unknowns
While we have made extraordinary strides forward in better understanding SARS-CoV-2 and both the triumphs and the failures of the response to the greatest public health challenge of our lifetime, the lessons we've learned have highlighted the many questions that remain. We will be studying many aspects of the pandemic for decades. Long after SARS-CoV-2 is finished with humanity on a global scale, we will not be finished with it. Some of these remaining questions won't have easy answers, and in fact may not even be answerable. But it is critical to engage with these questions as we move into a post-pandemic future.
The origin of SARS-CoV-2.
This topic is as confusing and murky as it is contentious, proving to be as confounding to science as it is disruptive to geopolitics. Multiple hypotheses abound: SARS-CoV-2 emerged into the human population naturally, passing from an infected animal to an unlucky human in the wrong place at the wrong time in a process called zoonotic spillover. This natural origin hypothesis is considered the most likely, as this is overwhelmingly the most common path for novel viruses to emerge in the human population.
Tracing SARS-CoV-2 back to its source is critical for both understanding how this pandemic began and preventing the emergence of SARS-CoV-3, which almost certainly is circulating in wildlife along with a frighteningly large number of other potential pandemic pathogens.
However, the evidence supporting this hypothesis is scant, and limited to genetic analyses that don't indicate anything artificial or engineered about the SARS-CoV-2 genome, as well as some very small studies suggesting that people who live close to bat caves in southern China have antibodies to closely related viruses. Such uncertainty has led to several other hypotheses, including that the virus emerged from a laboratory at the Wuhan Institute of Virology, either through accident or design. While there is far more speculation than evidence affirming any laboratory origin hypothesis, neither can be definitively excluded and both should be fairly investigated. In addition, the Chinese government has suggested that SARS-CoV-2 was imported via frozen seafood from Europe or North America. This hypothesis strains credulity, given that the most closely related viruses have been identified in China and transmission by indirect contact (with contaminated objects, or fomites, is thought to be uncommon), but it still should be ruled out objectively.
About the only thing most experts agree on is that SARS-CoV-2 evolved from an ancestral betacoronavirus that likely was circulating in bats. However, because we have not yet found that ancestral virus in nature, we are left still looking. Sometimes origin investigations into zoonotic origins can take decades, since we live in a big world, with many wild animals carrying many different viruses at different times in their lives. Trying to find the immediate forbear of SARS-CoV-2 in wildlife is like seeking a very specific tiny needle in a planet-sized haystack that is also littered with other tiny needles.
To further complicate matters, there is the possibility that SARS-CoV-2 did not spill over from bats to humans directly, but stopped off in another species along the way. Intermediary species have been involved in the transmission of both SARS-CoV and MERS-CoV, and we already know that SARS-CoV-2 can infect other animal species, including minks, dogs, and cats.
And if the science weren't complex enough, conducting any type of origin investigation, but particularly a rigorous independent investigation of lab origin theories, depends on other countries maintaining a productive diplomatic relationship with the Chinese government. That relationship erodes every time another piece is published outside China that treats laboratory origin as a foregone conclusion. Tracing SARS-CoV-2 back to its source is critical for both understanding how this pandemic began and preventing the emergence of SARS-CoV-3, which almost certainly is circulating in wildlife along with a frighteningly large number of other potential pandemic pathogens. But it won't be easy and we need to prepare ourselves for the possibility of a very long and arduous search for answers.
The long-term consequences of COVID-19.
While it is not clear how common "long COVID" is, one thing is certain: it has impacted a substantial number of COVID-19 survivors' lives. It remains unknown what predisposes a person to this outcome, now dubbed post-acute sequelae of COVID-19 (PASC). Nor does anyone truly know how long it lasts, or even what the most common presentation of it looks like. Many patients have reported a diverse array of symptoms, some very severe, that have persisted for months.
PASC can range from recurring neurological problems to hair and tooth loss to permanent lung injury. Some people have reported relapsing pain and severe fatigue similar to myalgic encephalomyelitis or chronic fatigue syndrome. Even more troubling, PASC can be severe in patients who reported having extremely mild acute COVID-19. Last month, the National Institutes of Health announced plans to study PASC in detail, but it may be some time before we know the cause (or causes) of PASC, much less how to treat it and ameliorate its impact on those suffering from it. But the potential for long-term debilitating illness persisting long after the resolution of acute SARS-CoV-2 infection suggests that even when the pandemic is behind us, public health will continue to struggle with the legacy of COVID-19.
Immune correlates of protection and durability.
While vaccine trials were designed to sacrifice little in the way of assessing short-term efficacy, they did not assess the length of time that protective immunity will last. This was because of the urgency of the situation, and allowed us to begin vaccinating as soon as we learned that the vaccines were safe and effective in the short term. Durability studies are one reason why normally vaccine trials can take over a decade, as unfortunately the only way to assess how long a vaccine lasts is to wait and see when protection begins to wane.
Furthermore, because the virus is novel and the technologies underlying the vaccine platforms are being used for the first time at population scale, we haven't yet defined correlates of protection for the vaccines. Correlates of protection are easily measurable features, such as antibody levels or cell counts, that can be used as surrogates for vaccine function. In other words, what we are missing is the knowledge of how many antibodies, or T-cells, does your immune system actually need to protect you from infection? We know that a high number is protective, but the question is how high.
Until we have enough data to define these correlates, we have to continue to follow trial participants and analyze observational studies of vaccinated individuals, which can be tedious as well as time-consuming. So it may be some time before we can advise people confidently about how long vaccine protection will last beyond a year or so, based on the duration of immune function in people who have recovered from natural SARS-CoV-2 infection. The good news is that protective immune responses can be easily restored with a booster shot, but that will present major logistical challenges if needed while global immunization efforts are still underway.
What price will we pay for nationalizing vaccine responses?
Finally, one of the biggest questions as we move into the post-pandemic future in the developed world is what the decision to respond nationally, rather than as a cooperative global community, will cost us in terms of truly ending the pandemic. Without question, in countries like the U.S., which will have enough vaccine doses in the next few months to vaccinate every American who wants one, the pandemic will end for most people's daily lives. But globally, the reality is very different. Many countries have yet to administer a single dose of any vaccine. While this may not seem relevant to people who do not intend to travel to those countries, it is relevant to every human being on earth. None of us are safe until all of us are safe.
Viruses infect their hosts regardless of what passport they carry. Pandemics, by definition, are global epidemics, and thus impact the global population. If people are vaccinated only in certain countries, SARS-CoV-2 can continue to circulate in populations with less immunization and fewer barriers to infection. As the U.S. today reaches this grim anniversary along with the rest of the world, we would do well to remember the lessons we've learned as we forge ahead with filling the remaining gaps in our knowledge.