Your Digital Avatar May One Day Get Sick Before You Do
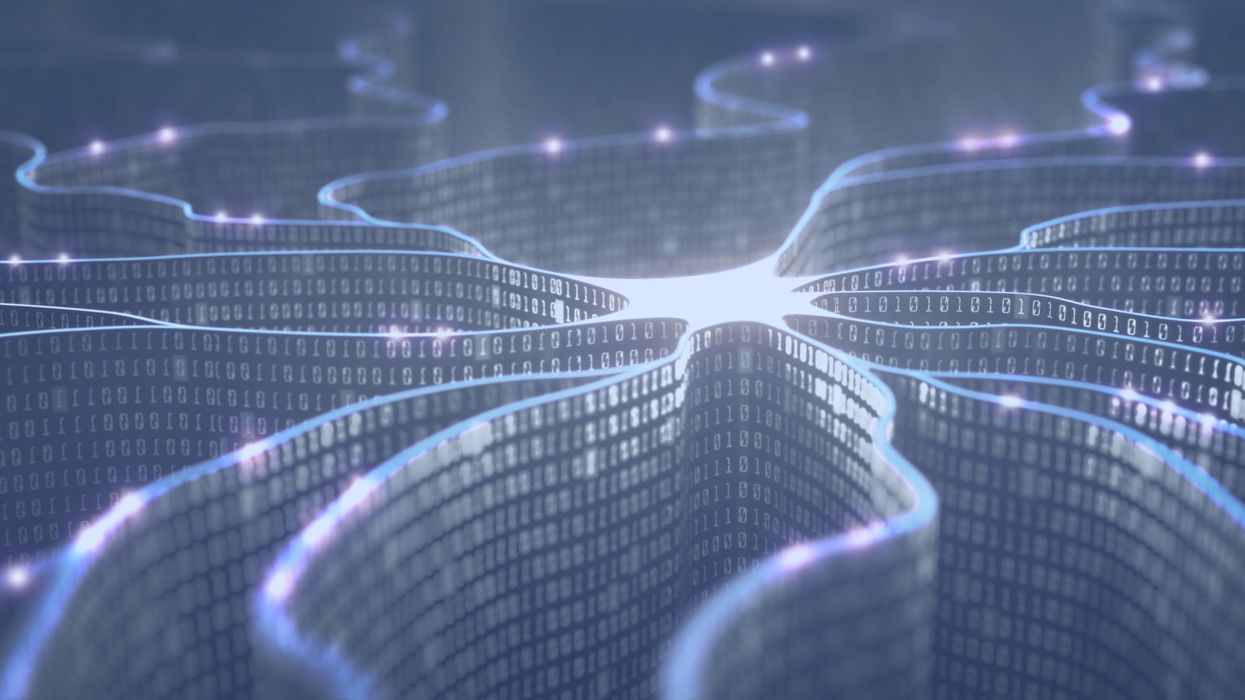
Artificial neurons in a concept of artificial intelligence.
Artificial intelligence is everywhere, just not in the way you think it is.
These networks, loosely designed after the human brain, are interconnected computers that have the ability to "learn."
"There's the perception of AI in the glossy magazines," says Anders Kofod-Petersen, a professor of Artificial Intelligence at the Norwegian University of Science and Technology. "That's the sci-fi version. It resembles the small guy in the movie AI. It might be benevolent or it might be evil, but it's generally intelligent and conscious."
"And this is, of course, as far from the truth as you can possibly get."
What Exactly Is Artificial Intelligence, Anyway?
Let's start with how you got to this piece. You likely came to it through social media. Your Facebook account, Twitter feed, or perhaps a Google search. AI influences all of those things, machine learning helping to run the algorithms that decide what you see, when, and where. AI isn't the little humanoid figure; it's the system that controls the figure.
"AI is being confused with robotics," Eleonore Pauwels, Director of the Anticipatory Intelligence Lab with the Science and Technology Innovation Program at the Wilson Center, says. "What AI is right now is a data optimization system, a very powerful data optimization system."
The revolution in recent years hasn't come from the method scientists and other researchers use. The general ideas and philosophies have been around since the late 1960s. Instead, the big change has been the dramatic increase in computing power, primarily due to the development of neural networks. These networks, loosely designed after the human brain, are interconnected computers that have the ability to "learn." An AI, for example, can be taught to spot a picture of a cat by looking at hundreds of thousands of pictures that have been labeled "cat" and "learning" what a cat looks like. Or an AI can beat a human at Go, an achievement that just five years ago Kofod-Petersen thought wouldn't be accomplished for decades.
"It's very difficult to argue that something is intelligent if it can't learn, and these algorithms are getting pretty good at learning stuff. What they are not good at is learning how to learn."
Medicine is the field where this expertise in perception tasks might have the most influence. It's already having an impact as iPhones use AI to detect cancer, Apple watches alert the wearer to a heart problem, AI spots tuberculosis and the spread of breast cancer with a higher accuracy than human doctors, and more. Every few months, another study demonstrates more possibility. (The New Yorker published an article about medicine and AI last year, so you know it's a serious topic.)
But this is only the beginning. "I personally think genomics and precision medicine is where AI is going to be the biggest game-changer," Pauwels says. "It's going to completely change how we think about health, our genomes, and how we think about our relationship between our genotype and phenotype."
The Fundamental Breakthrough That Must Be Solved
To get there, however, researchers will need to make another breakthrough, and there's debate about how long that will take. Kofod-Petersen explains: "If we want to move from this narrow intelligence to this broader intelligence, that's a very difficult problem. It basically boils down to that we haven't got a clue about what intelligence actually is. We don't know what intelligence means in a biological sense. We think we might recognize it but we're not completely sure. There isn't a working definition. We kind of agree with the biologists that learning is an aspect of it. It's very difficult to argue that something is intelligent if it can't learn, and these algorithms are getting pretty good at learning stuff. What they are not good at is learning how to learn. They can learn specific tasks but we haven't approached how to teach them to learn to learn."
In other words, current AI is very, very good at identifying that a picture of a cat is, in fact, a cat – and getting better at doing so at an incredibly rapid pace – but the system only knows what a "cat" is because that's what a programmer told it a furry thing with whiskers and two pointy ears is called. If the programmer instead decided to label the training images as "dogs," the AI wouldn't say "no, that's a cat." Instead, it would simply call a furry thing with whiskers and two pointy ears a dog. AI systems lack the explicit inference that humans do effortlessly, almost without thinking.
Pauwels believes that the next step is for AI to transition from supervised to unsupervised learning. The latter means that the AI isn't answering questions that a programmer asks it ("Is this a cat?"). Instead, it's almost like it's looking at the data it has, coming up with its own questions and hypothesis, and answering them or putting them to the test. Combining this ability with the frankly insane processing power of the computer system could result in game-changing discoveries.
In the not-too-distant future, a doctor could run diagnostics on a digital avatar, watching which medical conditions present themselves before the person gets sick in real life.
One company in China plans to develop a way to create a digital avatar of an individual person, then simulate that person's health and medical information into the future. In the not-too-distant future, a doctor could run diagnostics on a digital avatar, watching which medical conditions presented themselves – cancer or a heart condition or anything, really – and help the real-life version prevent those conditions from beginning or treating them before they became a life-threatening issue.
That, obviously, would be an incredibly powerful technology, and it's just one of the many possibilities that unsupervised AI presents. It's also terrifying in the potential for misuse. Even the term "unsupervised AI" brings to mind a dystopian landscape where AI takes over and enslaves humanity. (Pick your favorite movie. There are dozens.) This is a concern, something for developers, programmers, and scientists to consider as they build the systems of the future.
The Ethical Problem That Deserves More Attention
But the more immediate concern about AI is much more mundane. We think of AI as an unbiased system. That's incorrect. Algorithms, after all, are designed by someone or a team, and those people have explicit or implicit biases. Intentionally, or more likely not, they introduce these biases into the very code that forms the basis for the AI. Current systems have a bias against people of color. Facebook tried to rectify the situation and failed. These are two small examples of a larger, potentially systemic problem.
It's vital and necessary for the people developing AI today to be aware of these issues. And, yes, avoid sending us to the brink of a James Cameron movie. But AI is too powerful a tool to ignore. Today, it's identifying cats and on the verge of detecting cancer. In not too many tomorrows, it will be on the forefront of medical innovation. If we are careful, aware, and smart, it will help simulate results, create designer drugs, and revolutionize individualize medicine. "AI is the only way to get there," Pauwels says.
Biohackers Made a Cheap and Effective Home Covid Test -- But No One Is Allowed to Use It
A stock image of a home test for COVID-19.
Last summer, when fast and cheap Covid tests were in high demand and governments were struggling to manufacture and distribute them, a group of independent scientists working together had a bit of a breakthrough.
Working on the Just One Giant Lab platform, an online community that serves as a kind of clearing house for open science researchers to find each other and work together, they managed to create a simple, one-hour Covid test that anyone could take at home with just a cup of hot water. The group tested it across a network of home and professional laboratories before being listed as a semi-finalist team for the XPrize, a competition that rewards innovative solutions-based projects. Then, the group hit a wall: they couldn't commercialize the test.
They wanted to keep their project open source, making it accessible to people around the world, so they decided to forgo traditional means of intellectual property protection and didn't seek patents. (They couldn't afford lawyers anyway). And, as a loose-knit group that was not supported by a traditional scientific institution, working in community labs and homes around the world, they had no access to resources or financial support for manufacturing or distributing their test at scale.
But without ethical and regulatory approval for clinical testing, manufacture, and distribution, they were legally unable to create field tests for real people, leaving their inexpensive, $16-per-test, innovative product languishing behind, while other, more expensive over-the-counter tests made their way onto the market.
Who Are These Radical Scientists?
Independent, decentralized biomedical research has come of age. Also sometimes called DIYbio, biohacking, or community biology, depending on whom you ask, open research is today a global movement with thousands of members, from scientists with advanced degrees to middle-grade students. Their motivations and interests vary across a wide spectrum, but transparency and accessibility are key to the ethos of the movement. Teams are agile, focused on shoestring-budget R&D, and aim to disrupt business as usual in the ivory towers of the scientific establishment.
Ethics oversight is critical to ensuring that research is conducted responsibly, even by biohackers.
Initiatives developed within the community, such as Open Insulin, which hopes to engineer processes for affordable, small-batch insulin production, "Slybera," a provocative attempt to reverse engineer a $1 million dollar gene therapy, and the hundreds of projects posted on the collaboration platform Just One Giant Lab during the pandemic, all have one thing in common: to pursue testing in humans, they need an ethics oversight mechanism.
These groups, most of which operate collaboratively in community labs, homes, and online, recognize that some sort of oversight or guidance is useful—and that it's the right thing to do.
But also, and perhaps more immediately, they need it because federal rules require ethics oversight of any biomedical research that's headed in the direction of the consumer market. In addition, some individuals engaged in this work do want to publish their research in traditional scientific journals, which—you guessed it—also require that research has undergone an ethics evaluation. Ethics oversight is critical to ensuring that research is conducted responsibly, even by biohackers.
Bridging the Ethics Gap
The problem is that traditional oversight mechanisms, such as institutional review boards at government or academic research institutions, as well as the private boards utilized by pharmaceutical companies, are not accessible to most independent researchers. Traditional review boards are either closed to the public, or charge fees that are out of reach for many citizen science initiatives. This has created an "ethics gap" in nontraditional scientific research.
Biohackers are seen in some ways as the direct descendents of "white hat" computer hackers, or those focused on calling out security holes and contributing solutions to technical problems within self-regulating communities. In the case of health and biotechnology, those problems include both the absence of treatments and the availability of only expensive treatments for certain conditions. As the DIYbio community grows, there needs to be a way to provide assurance that, when the work is successful, the public is able to benefit from it eventually. The team that developed the one-hour Covid test found a potential commercial partner and so might well overcome the oversight hurdle, but it's been 14 months since they developed the test--and counting.
In short, without some kind of oversight mechanism for the work of independent biomedical researchers, the solutions they innovate will never have the opportunity to reach consumers.
In a new paper in the journal Citizen Science: Theory & Practice, we consider the issue of the ethics gap and ask whether ethics oversight is something nontraditional researchers want, and if so, what forms it might take. Given that individuals within these communities sometimes vehemently disagree with each other, is consensus on these questions even possible?
We learned that there is no "one size fits all" solution for ethics oversight of nontraditional research. Rather, the appropriateness of any oversight model will depend on each initiative's objectives, needs, risks, and constraints.
We also learned that nontraditional researchers are generally willing (and in some cases eager) to engage with traditional scientific, legal, and bioethics experts on ethics, safety, and related questions.
We suggest that these experts make themselves available to help nontraditional researchers build infrastructure for ethics self-governance and identify when it might be necessary to seek outside assistance.
Independent biomedical research has promise, but like any emerging science, it poses novel ethical questions and challenges. Existing research ethics and oversight frameworks may not be well-suited to answer them in every context, so we need to think outside the box about what we can create for the future. That process should begin by talking to independent biomedical researchers about their activities, priorities, and concerns with an eye to understanding how best to support them.
Christi Guerrini, JD, MPH studies biomedical citizen science and is an Associate Professor at Baylor College of Medicine. Alex Pearlman, MA, is a science journalist and bioethicist who writes about emerging issues in biotechnology. They have recently launched outlawbio.org, a place for discussion about nontraditional research.
Sept. 13th Event: Delta, Vaccines, and Breakthrough Infections
A visualization of the virus that causes COVID-19.
This virtual event will convene leading scientific and medical experts to address the public's questions and concerns about COVID-19 vaccines, Delta, and breakthrough infections. Audience Q&A will follow the panel discussion. Your questions can be submitted in advance at the registration link.
DATE:
Monday, September 13th, 2021
12:30 p.m. - 1:45 p.m. EDT
REGISTER:
Dr. Amesh Adalja, M.D., FIDSA, Senior Scholar, Johns Hopkins Center for Health Security; Adjunct Assistant Professor, Johns Hopkins Bloomberg School of Public Health; Affiliate of the Johns Hopkins Center for Global Health. His work is focused on emerging infectious disease, pandemic preparedness, and biosecurity.
Dr. Nahid Bhadelia, M.D., MALD, Founding Director, Boston University Center for Emerging Infectious Diseases Policy and Research (CEID); Associate Director, National Emerging Infectious Diseases Laboratories (NEIDL), Boston University; Associate Professor, Section of Infectious Diseases, Boston University School of Medicine. She is an internationally recognized leader in highly communicable and emerging infectious diseases (EIDs) with clinical, field, academic, and policy experience in pandemic preparedness.
Dr. Akiko Iwasaki, Ph.D., Waldemar Von Zedtwitz Professor of Immunobiology and Molecular, Cellular and Developmental Biology and Professor of Epidemiology (Microbial Diseases), Yale School of Medicine; Investigator, Howard Hughes Medical Institute. Her laboratory researches how innate recognition of viral infections lead to the generation of adaptive immunity, and how adaptive immunity mediates protection against subsequent viral challenge.
Dr. Marion Pepper, Ph.D., Associate Professor, Department of Immunology, University of Washington. Her lab studies how cells of the adaptive immune system, called CD4+ T cells and B cells, form immunological memory by visualizing their differentiation, retention, and function.
This event is the third of a four-part series co-hosted by Leaps.org, the Aspen Institute Science & Society Program, and the Sabin–Aspen Vaccine Science & Policy Group, with generous support from the Gordon and Betty Moore Foundation and the Howard Hughes Medical Institute.
Kira Peikoff was the editor-in-chief of Leaps.org from 2017 to 2021. As a journalist, her work has appeared in The New York Times, Newsweek, Nautilus, Popular Mechanics, The New York Academy of Sciences, and other outlets. She is also the author of four suspense novels that explore controversial issues arising from scientific innovation: Living Proof, No Time to Die, Die Again Tomorrow, and Mother Knows Best. Peikoff holds a B.A. in Journalism from New York University and an M.S. in Bioethics from Columbia University. She lives in New Jersey with her husband and two young sons. Follow her on Twitter @KiraPeikoff.