Clever Firm Predicts Patients Most at Risk, Then Tries to Intervene Before They Get Sicker
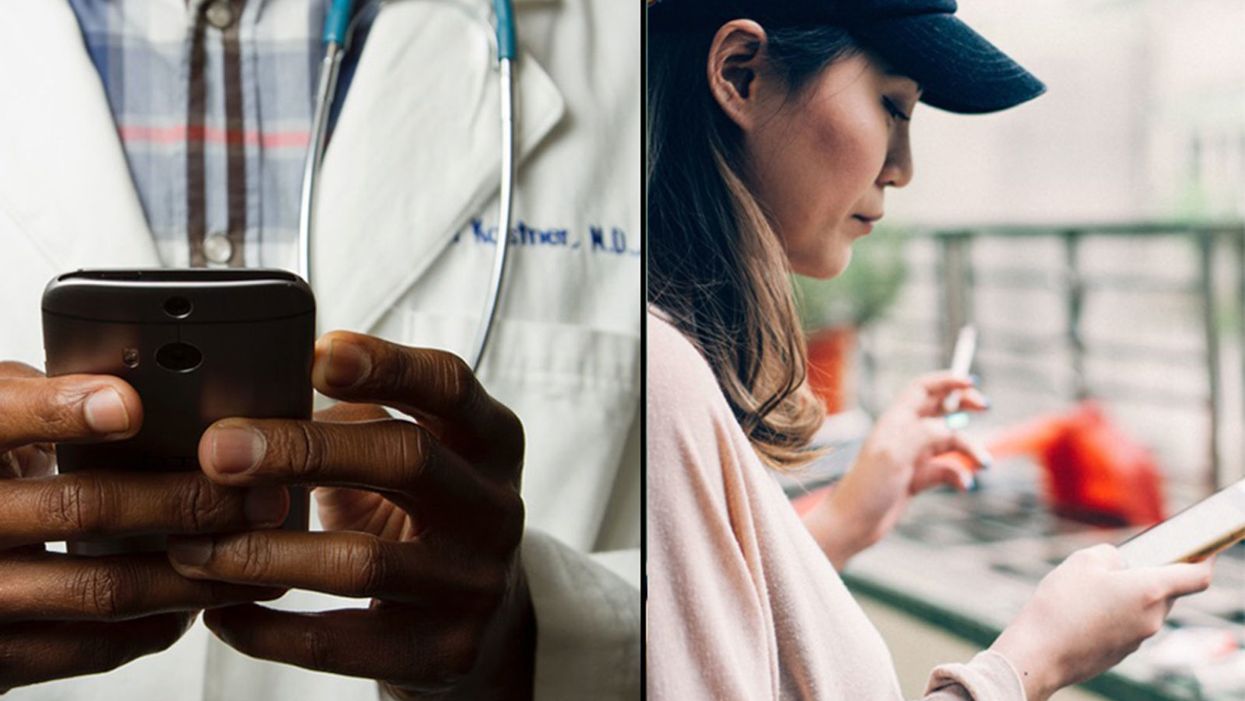
Health firm Populytics tracks and analyzes patient data, and makes care suggestions based on that data.
The diabetic patient hit the danger zone.
Ideally, blood sugar, measured by an A1C test, rests at 5.9 or less. A 7 is elevated, according to the Diabetes Council. Over 10, and you're into the extreme danger zone, at risk of every diabetic crisis from kidney failure to blindness.
In three months of working with a case manager, Jen's blood sugar had dropped to 7.2, a much safer range.
This patient's A1C was 10. Let's call her Jen for the sake of this story. (Although the facts of her case are real, the patient's actual name wasn't released due to privacy laws.).
Jen happens to live in Pennsylvania's Lehigh Valley, home of the nonprofit Lehigh Valley Health Network, which has eight hospital campuses and various clinics and other services. This network has invested more than $1 billion in IT infrastructure and founded Populytics, a spin-off firm that tracks and analyzes patient data, and makes care suggestions based on that data.
When Jen left the doctor's office, the Populytics data machine started churning, analyzing her data compared to a wealth of information about future likely hospital visits if she did not comply with recommendations, as well as the potential positive impacts of outreach and early intervention.
About a month after Jen received the dangerous blood test results, a community outreach specialist with psychological training called her. She was on a list generated by Populytics of follow-up patients to contact.
"It's a very gentle conversation," says Cathryn Kelly, who manages a care coordination team at Populytics. "The case manager provides them understanding and support and coaching." The goal, in this case, was small behavioral changes that would actually stick, like dietary ones.
In three months of working with a case manager, Jen's blood sugar had dropped to 7.2, a much safer range. The odds of her cycling back to the hospital ER or veering into kidney failure, or worse, had dropped significantly.
While the health network is extremely localized to one area of one state, using data to inform precise medical decision-making appears to be the wave of the future, says Ann Mongovern, the associate director of Health Care Ethics at the Markkula Center for Applied Ethics at Santa Clara University in California.
"Many hospitals and hospital systems don't yet try to do this at all, which is striking given where we're at in terms of our general technical ability in this society," Mongovern says.
How It Happened
While many hospitals make money by filling beds, the Lehigh Valley Health Network, as a nonprofit, accepts many patients on Medicaid and other government insurances that don't cover some of the costs of a hospitalization. The area's population is both poorer and older than national averages, according to the U.S. Census data, meaning more people with higher medical needs that may not have the support to care for themselves. They end up in the ER, or worse, again and again.
In the early 2000s, LVHN CEO Dr. Brian Nester started wondering if his health network could develop a way to predict who is most likely to land themselves a pricey ICU stay -- and offer support before those people end up needing serious care.
Embracing data use in such specific ways also brings up issues of data security and patient safety.
"There was an early understanding, even if you go back to the (federal) balanced budget act of 1997, that we were just kicking the can down the road to having a functional financial model to deliver healthcare to everyone with a reasonable price," Nester says. "We've got a lot of people living longer without more of an investment in the healthcare trust."
Popultyics, founded in 2013, was the result of years of planning and agonizing over those population numbers and cost concerns.
"We looked at our own health plan," Nester says. Out of all the employees and dependants on the LVHN's own insurance network, "roughly 1.5 percent of our 25,000 people — under 400 people — drove $30 million of our $130 million on insurance costs -- about 25 percent."
"You don't have to boil the ocean to take cost out of the system," he says. "You just have to focus on that 1.5%."
Take Jen, the diabetic patient. High blood sugar can lead to kidney failure, which can mean weekly expensive dialysis for 20 years. Investing in the data and staff to reach patients, he says, is "pennies compared to $100 bills."
For most doctors, "there's no awareness for providers to know who they should be seeing vs. who they are seeing. There's no incentive, because the incentive is to see as many patients as you can," he says.
To change that, first the LVHN invested in the popular medical management system, Epic. Then, they negotiated with the top 18 insurance companies that cover patients in the region to allow access to their patient care data, which means they have reams of patient history to feed the analytics machine in order to make predictions about outcomes. Nester admits not every hospital could do that -- with 52 percent of the market share, LVHN had a very strong negotiating position.
Third party services take that data and churn out analytics that feeds models and care management plans. All identifying information is stripped from the data.
"We can do predictive modeling in patients," says Populytics President and CEO Gregory Kile. "We can identify care gaps. Those care gaps are noted as alerts when the patient presents at the office."
Kile uses himself as a hypothetical patient.
"I pull up Gregory Kile, and boom, I see a flag or an alert. I see he hasn't been in for his last blood test. There is a care gap there we need to complete."
"There's just so much more you can do with that information," he says, envisioning a future where follow-up for, say, knee replacement surgery and outcomes could be tracked, and either validated or changed.
Ethical Issues at the Forefront
Of course, embracing data use in such specific ways also brings up issues of security and patient safety. For example, says medical ethicist Mongovern, there are many touchpoints where breaches could occur. The public has a growing awareness of how data used to personalize their experiences, such as social media analytics, can also be monetized and sold in ways that benefit a company, but not the user. That's not to say data supporting medical decisions is a bad thing, she says, just one with potential for public distrust if not handled thoughtfully.
"You're going to need to do this to stay competitive," she says. "But there's obviously big challenges, not the least of which is patient trust."
So far, a majority of the patients targeted – 62 percent -- appear to embrace the effort.
Among the ways the LVHN uses the data is monthly reports they call registries, which include patients who have just come in contact with the health network, either through the hospital or a doctor that works with them. The community outreach team members at Populytics take the names from the list, pull their records, and start calling. So far, a majority of the patients targeted – 62 percent -- appear to embrace the effort.
Says Nester: "Most of these are vulnerable people who are thrilled to have someone care about them. So they engage, and when a person engages in their care, they take their insulin shots. It's not rocket science. The rocket science is in identifying who the people are — the delivery of care is easy."
New Tech Can Predict Breast Cancer Years in Advance
Breast cancer survivors rally in a support group.
Every two minutes, a woman is diagnosed with breast cancer. The question is, can those at high risk be identified early enough to survive?
New AI software has predicted risk equally well in both white and black women for the first time.
The current standard practice in medicine is not exactly precise. It relies on age, family history of cancer, and breast density, among other factors, to determine risk. But these factors do not always tell the whole story, leaving many women to slip through the cracks. In addition, a racial gap persists in breast cancer treatment and survival. African-American women are 42 percent more likely to die from the disease despite relatively equal rates of diagnosis.
But now those grim statistics could be changing. A team of researchers from MIT's Computer Science and Artificial Intelligence Laboratory have developed a deep learning model that can more accurately predict a patient's breast cancer risk compared to established clinical guidelines – and it has predicted risk equally well in both white and black women for the first time.
The Lowdown
Study results published in Radiology described how the AI software read mammogram images from more than 60,000 patients at Massachusetts General Hospital to identify subtle differences in breast tissue that pointed to potential risk factors, even in their earliest stages. The team accessed the patients' actual diagnoses and determined that the AI model was able to correctly place 31 percent of all cancer patients in the highest-risk category of developing breast cancer within five years of the examination, compared to just 18 percent for existing models.
"Each image has hundreds of thousands of pixels identifying something that may not necessarily be detected by the human eye," said MIT professor Regina Barzilay, one of the study's lead authors. "We all have limited visual capacities so it seems some machines trained on hundreds of thousands of images with a known outcome can capture correlations the human eye might not notice."
Barzilay, a breast cancer survivor herself, had abnormal tissue patterns on mammograms in 2012 and 2013, but wasn't diagnosed until after a 2014 image reading, illustrating the limitations of human processing alone.
MIT professor Regina Barzilay, a lead author on the new study and a breast cancer survivor herself.
(Courtesy MIT)
Next up: The MIT team is looking at training the model to detect other cancers and health risks. Barzilay recalls how a cardiologist told her during a conference that women with heart diseases had a different pattern of calcification on their mammograms, demonstrating how already existing images can be used to extract other pieces of information about a person's health status.
Integration of the AI model in standard care could help doctors better tailor screening and prevention programs based on actual instead of perceived risk. Patients who might register as higher risk by current guidelines could be identified as lower risk, helping resolve conflicting opinions about how early and how often women should receive mammograms.
Open Questions: While the results were promising, it's unknown how well the model will work on a larger scale, as the study looked at data from just one institution and used mammograms supplied by just one hospital. Some risk factor information was also unavailable for certain patients during the study, leaving researchers unable to fully compare the AI model's performance to that of the traditional standard.
One incentive to wider implementation and study, however, is the bonus that no new hardware is required to use the AI model. With other institutions now showing interest, this software could lead to earlier routine detection and treatment of breast cancer — resulting in more lives saved.
A pregnant woman is uncertain which medicine is safe to take.
Sarah Mancoll was 22 years old when she noticed a bald spot on the back of her head. A dermatologist confirmed that it was alopecia aerata, an autoimmune disorder that causes hair loss.
Of 213 new drugs approved from 2003 to 2012, only five percent included any data from pregnant women.
She successfully treated the condition with corticosteroid shots for nearly 10 years. Then Mancoll and her husband began thinking about starting a family. Would the shots be safe for her while pregnant? For the fetus? What about breastfeeding?
Mancoll consulted her primary care physician, her dermatologist, even a pediatrician. Without clinical data, no one could give her a definitive answer, so she stopped treatment to be "on the safe side." By the time her son was born, she'd lost at least half her hair. She returned to her Washington, D.C., public policy job two months later entirely bald—and without either eyebrows or eyelashes.
After having two more children in quick succession, Mancoll recently resumed the shots but didn't forget her experience. Today, she is an advocate for including more pregnant and lactating women in clinical studies so they can have more information about therapies than she did.
"I live a very privileged life, and I'll do just fine with or without hair, but it's not just about me," Mancoll said. "It's about a huge population of women who are being disenfranchised…They're invisible."
About 4 million women give birth each year in the United States, and many face medical conditions, from hypertension and diabetes to psychiatric disorders. A 2011 study showed that most women reported taking at least one medication while pregnant between 1976 and 2008. But for decades, pregnant and lactating women have been largely excluded from clinical drug studies that rigorously test medications for safety and effectiveness.
An estimated 98 percent of government-approved drug treatments between 2000 and 2010 had insufficient data to determine risk to the fetus, and close to 75 percent had no human pregnancy data at all. All told, of 213 new pharmaceuticals approved from 2003 to 2012, only five percent included any data from pregnant women.
But recent developments suggest that could be changing. Amid widespread concerns about increased maternal mortality rates, women's health advocates, physicians, and researchers are sensing and encouraging a cultural shift toward protecting women through responsible research instead of from research.
"The question is not whether to do research with pregnant women, but how," Anne Drapkin Lyerly, professor and associate director of the Center for Bioethics at the University of North Carolina at Chapel Hill, wrote last year in an op-ed. "These advances are essential. It is well past time—and it is morally imperative—for research to benefit pregnant women."
"In excluding pregnant women from drug trials to protect them from experimentation, we subject them to uncontrolled experimentation."
To that end, the American College of Obstetricians and Gynecologists' Committee on Ethics acknowledged that research trials need to be better designed so they don't "inappropriately constrain the reproductive choices of study participants or unnecessarily exclude pregnant women." A federal task force also called for significantly expanded research and the removal of regulatory barriers that make it difficult for pregnant and lactating women to participate in research.
Several months ago, a government change to a regulation known as the Common Rule took effect, removing pregnant women as a "vulnerable population" in need of special protections -- a designation that had made it more difficult to enroll them in clinical drug studies. And just last week, the U.S. Food and Drug Administration (FDA) issued new draft guidances for industry on when and how to include pregnant and lactating women in clinical trials.
Inclusion is better than the absence of data on their treatment, said Catherine Spong, former chair of the federal task force.
"It's a paradox," said Spong, professor of obstetrics and gynecology and chief of maternal fetal medicine at University of Texas Southwestern Medical Center. "There is a desire to protect women and fetuses from harm, which is translated to a reluctance to include them in research. By excluding them, the evidence for their care is limited."
Jacqueline Wolf, a professor of the history of medicine at Ohio University, agreed.
"In excluding pregnant women from drug trials to protect them from experimentation, we subject them to uncontrolled experimentation," she said. "We give them the medication without doing any research, and that's dangerous."
Women, of course, don't stop getting sick or having chronic medical conditions just because they are pregnant or breastfeeding, and conditions during pregnancy can affect a baby's health later in life. Evidence-based data is important for other reasons, too.
Pregnancy can dramatically change a woman's physiology, affecting how drugs act on her body and how her body acts or reacts to drugs. For instance, pregnant bodies can more quickly clear out medications such as glyburide, used during diabetes in pregnancy to stabilize high blood-sugar levels, which can be toxic to the fetus and harmful to women. That means a regular dose of the drug may not be enough to control blood sugar and prevent poor outcomes.
Pregnant patients also may be reluctant to take needed drugs for underlying conditions (and doctors may be hesitant to prescribe them), which in turn can cause more harm to the woman and fetus than had they been treated. For example, women who have severe asthma attacks while pregnant are at a higher risk of having low-birthweight babies, and pregnant women with uncontrolled diabetes in early pregnancy have more than four times the risk of birth defects.
Current clinical trials involving pregnant women are assessing treatments for obstructive sleep apnea, postpartum hemorrhage, lupus, and diabetes.
For Kate O'Brien, taking medication during her pregnancy was a matter of life and death. A freelance video producer who lives in New Jersey, O'Brien was diagnosed with tuberculosis in 2015 after she became pregnant with her second child, a boy. Even as she signed hospital consent forms, she had no idea if the treatment would harm him.
"It's a really awful experience," said O'Brien, who now is active with We are TB, an advocacy and support network. "All they had to tell me about the medication was just that women have been taking it for a really long time all over the world. That was the best they could do."
More and more doctors, researchers and women's health organizations and advocates are calling that unacceptable.
By indicating that filling current knowledge gaps is "a critical public health need," the FDA is signaling its support for advancing research with pregnant women, said Lyerly, also co-founder of the Second Wave Initiative, which promotes fair representation of the health interests of pregnant women in biomedical research and policies. "It's a very important shift."
Research with pregnant women can be done ethically, Lyerly said, whether by systematically collecting data from those already taking medications or enrolling pregnant women in studies of drugs or vaccines in development.
Current clinical trials involving pregnant women are assessing treatments for obstructive sleep apnea, postpartum hemorrhage, lupus, and diabetes. Notable trials in development target malaria and HIV prevention in pregnancy.
"It clearly is doable to do this research, and test trials are important to provide evidence for treatment," Spong said. "If we don't have that evidence, we aren't making the best educated decisions for women."