Clever Firm Predicts Patients Most at Risk, Then Tries to Intervene Before They Get Sicker
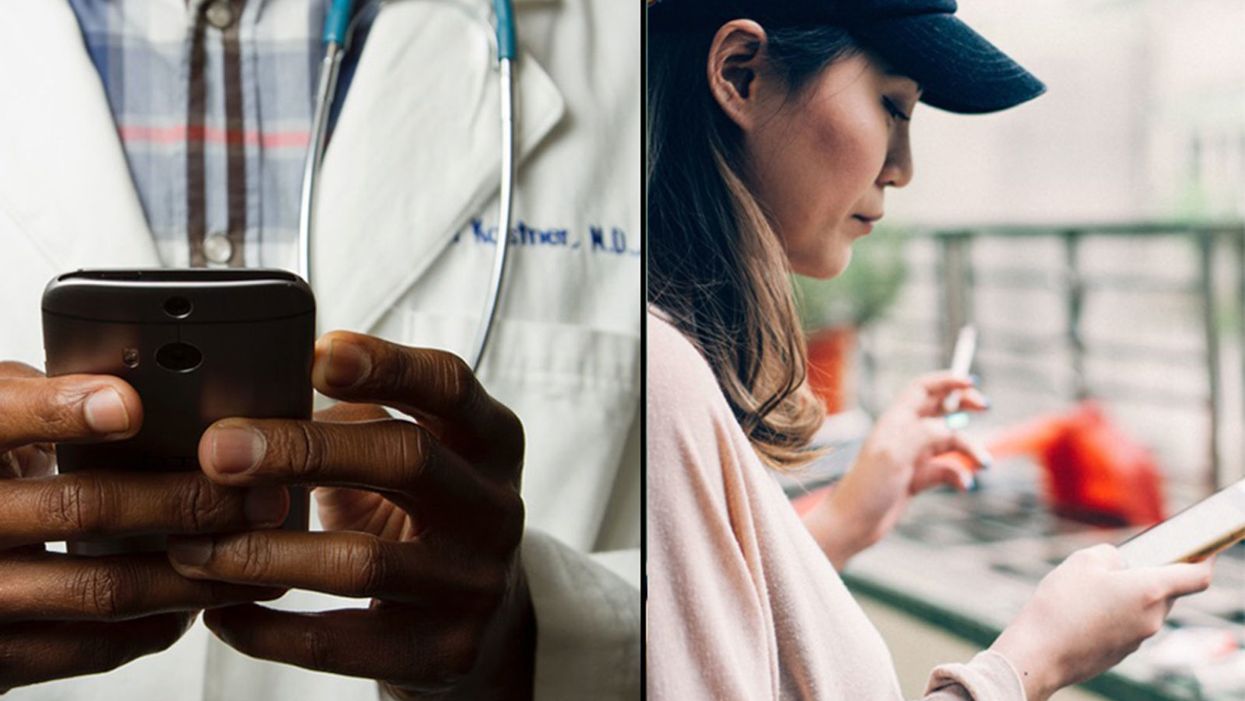
Health firm Populytics tracks and analyzes patient data, and makes care suggestions based on that data.
The diabetic patient hit the danger zone.
Ideally, blood sugar, measured by an A1C test, rests at 5.9 or less. A 7 is elevated, according to the Diabetes Council. Over 10, and you're into the extreme danger zone, at risk of every diabetic crisis from kidney failure to blindness.
In three months of working with a case manager, Jen's blood sugar had dropped to 7.2, a much safer range.
This patient's A1C was 10. Let's call her Jen for the sake of this story. (Although the facts of her case are real, the patient's actual name wasn't released due to privacy laws.).
Jen happens to live in Pennsylvania's Lehigh Valley, home of the nonprofit Lehigh Valley Health Network, which has eight hospital campuses and various clinics and other services. This network has invested more than $1 billion in IT infrastructure and founded Populytics, a spin-off firm that tracks and analyzes patient data, and makes care suggestions based on that data.
When Jen left the doctor's office, the Populytics data machine started churning, analyzing her data compared to a wealth of information about future likely hospital visits if she did not comply with recommendations, as well as the potential positive impacts of outreach and early intervention.
About a month after Jen received the dangerous blood test results, a community outreach specialist with psychological training called her. She was on a list generated by Populytics of follow-up patients to contact.
"It's a very gentle conversation," says Cathryn Kelly, who manages a care coordination team at Populytics. "The case manager provides them understanding and support and coaching." The goal, in this case, was small behavioral changes that would actually stick, like dietary ones.
In three months of working with a case manager, Jen's blood sugar had dropped to 7.2, a much safer range. The odds of her cycling back to the hospital ER or veering into kidney failure, or worse, had dropped significantly.
While the health network is extremely localized to one area of one state, using data to inform precise medical decision-making appears to be the wave of the future, says Ann Mongovern, the associate director of Health Care Ethics at the Markkula Center for Applied Ethics at Santa Clara University in California.
"Many hospitals and hospital systems don't yet try to do this at all, which is striking given where we're at in terms of our general technical ability in this society," Mongovern says.
How It Happened
While many hospitals make money by filling beds, the Lehigh Valley Health Network, as a nonprofit, accepts many patients on Medicaid and other government insurances that don't cover some of the costs of a hospitalization. The area's population is both poorer and older than national averages, according to the U.S. Census data, meaning more people with higher medical needs that may not have the support to care for themselves. They end up in the ER, or worse, again and again.
In the early 2000s, LVHN CEO Dr. Brian Nester started wondering if his health network could develop a way to predict who is most likely to land themselves a pricey ICU stay -- and offer support before those people end up needing serious care.
Embracing data use in such specific ways also brings up issues of data security and patient safety.
"There was an early understanding, even if you go back to the (federal) balanced budget act of 1997, that we were just kicking the can down the road to having a functional financial model to deliver healthcare to everyone with a reasonable price," Nester says. "We've got a lot of people living longer without more of an investment in the healthcare trust."
Popultyics, founded in 2013, was the result of years of planning and agonizing over those population numbers and cost concerns.
"We looked at our own health plan," Nester says. Out of all the employees and dependants on the LVHN's own insurance network, "roughly 1.5 percent of our 25,000 people — under 400 people — drove $30 million of our $130 million on insurance costs -- about 25 percent."
"You don't have to boil the ocean to take cost out of the system," he says. "You just have to focus on that 1.5%."
Take Jen, the diabetic patient. High blood sugar can lead to kidney failure, which can mean weekly expensive dialysis for 20 years. Investing in the data and staff to reach patients, he says, is "pennies compared to $100 bills."
For most doctors, "there's no awareness for providers to know who they should be seeing vs. who they are seeing. There's no incentive, because the incentive is to see as many patients as you can," he says.
To change that, first the LVHN invested in the popular medical management system, Epic. Then, they negotiated with the top 18 insurance companies that cover patients in the region to allow access to their patient care data, which means they have reams of patient history to feed the analytics machine in order to make predictions about outcomes. Nester admits not every hospital could do that -- with 52 percent of the market share, LVHN had a very strong negotiating position.
Third party services take that data and churn out analytics that feeds models and care management plans. All identifying information is stripped from the data.
"We can do predictive modeling in patients," says Populytics President and CEO Gregory Kile. "We can identify care gaps. Those care gaps are noted as alerts when the patient presents at the office."
Kile uses himself as a hypothetical patient.
"I pull up Gregory Kile, and boom, I see a flag or an alert. I see he hasn't been in for his last blood test. There is a care gap there we need to complete."
"There's just so much more you can do with that information," he says, envisioning a future where follow-up for, say, knee replacement surgery and outcomes could be tracked, and either validated or changed.
Ethical Issues at the Forefront
Of course, embracing data use in such specific ways also brings up issues of security and patient safety. For example, says medical ethicist Mongovern, there are many touchpoints where breaches could occur. The public has a growing awareness of how data used to personalize their experiences, such as social media analytics, can also be monetized and sold in ways that benefit a company, but not the user. That's not to say data supporting medical decisions is a bad thing, she says, just one with potential for public distrust if not handled thoughtfully.
"You're going to need to do this to stay competitive," she says. "But there's obviously big challenges, not the least of which is patient trust."
So far, a majority of the patients targeted – 62 percent -- appear to embrace the effort.
Among the ways the LVHN uses the data is monthly reports they call registries, which include patients who have just come in contact with the health network, either through the hospital or a doctor that works with them. The community outreach team members at Populytics take the names from the list, pull their records, and start calling. So far, a majority of the patients targeted – 62 percent -- appear to embrace the effort.
Says Nester: "Most of these are vulnerable people who are thrilled to have someone care about them. So they engage, and when a person engages in their care, they take their insulin shots. It's not rocket science. The rocket science is in identifying who the people are — the delivery of care is easy."
Should You Bank Your Kid’s Teeth for Stem Cells?
When Karen Davis attended a presentation at a dental conference in 2013, she unexpectedly discovered a service that could help her daughter, Madeline: storing stem cells derived from her teeth that potentially could be used in the future to treat her Crohn's disease.
"Even though this isn't a viable option today, I know how rapidly things can change."
Throughout high school, Madeline suffered from the painful autoimmune disorder, which wreaks havoc on the gastrointestinal system and can lead to life-threatening complications.
"I leave no stone unturned when it comes to medical care and this resonated with me," says Davis, a Dallas-based dental hygienist who was encouraged by advances in stem cell research. Later that year, when Madeline got her wisdom teeth extracted, Davis shipped them off to the Store-A-Tooth company in Massachusetts, where they will be kept frozen until needed. "Even though this isn't a viable option today, I know how rapidly things can change," says Davis. "To me, this was a worthwhile investment—I didn't want to miss out on an opportunity that would provide a pathway to a cure."
Karen Davis pictured with her daughter Madeline.
(Courtesy of Karen Davis)
The process itself was straightforward. Madeline's newly extracted wisdom teeth--baby teeth can be saved, too—were bathed in a special solution, loaded into a Styrofoam container lined with cold packs and sent to the stem cell company. There, a team harvested the dental stem cells from the pulp, then grew them in culture and cryogenically preserved them. Store-A-Tooth charges $1500-1749 for tooth collection and $120 per year for storage, while other dental pulp stem cell tissue banks cost $500-$600 upfront and in the $120 range annually for storage.
The rationale here is that if you missed out on banking your baby's umbilical cord blood, this gives you another chance to harvest their stem cells. "If their child later develops an illness that could be managed or even cured with stem cell therapy, this is an insurance policy," says Amr Moursi, DDS, PhD, chair of the department of pediatric dentistry at New York University College of Dentistry.
But is there a genuine potential here for some effective treatments in the relatively near future—or is this just another trendy fad? Scientific opinion is decidedly mixed. Stem cells have been heralded as the next frontier in medicine because of their versatility: with a little chemical coaxing, they can be transformed into different cell types, such as heart, blood or brain cells, to create tissue that can mend damaged body parts. Because they're taken from your own body, there's little chance of rejection, which means patients don't have to take strong antirejection drugs that can have all sorts of unpleasant side effects for the rest of their lives.
However, while stem cells are immature cells found in different tissues, ranging from abdominal fat to bone marrow, there is a vast difference between the stem cells found in cord blood and in teeth. Cord blood, which is culled from the umbilical cord when a baby is born, contains what are called hematopoietic stem cells (HSCs), which can mature into other blood cells. These type of stem cells have already been approved by the U.S. Food and Drug Administration to treat patients—especially children--with blood cancers, such as leukemias and lymphomas, and certain blood disorders like sickle cell anemia.
In contrast, stem cells in teeth are called mesenchymal stem cells (MSCs), which are found in dental pulp, the tissue in the center of the tooth that's filled with nerves and blood vessels. MSCs are adult stem cells normally found in the bone marrow that can transform into bone, fat, and cartilage cells, and also aid in the formation of blood stem cells.
"Right now we just don't have rigorous evidence that they can be used in that fashion and have real benefit."
Small studies on lab animals suggest that MSCs secrete growth factors—hormonal steroids or proteins—that can nurture ailing cells, act as powerful anti-inflammatory agents that could tame autoimmune disorders like the one that plagues Karen Davis's daughter, and may even generate new nerve and muscle tissue. Preliminary research suggests they potentially could treat medical conditions as varied as heart disease, spinal cord injury and type 1 diabetes by generating new cells, which can replace damaged or dead cells.
But this is all very early research and there's a vast difference between how cells behave in the tightly controlled environment of a lab versus the real world in a diverse population of human patients. "Right now we just don't have rigorous evidence that they can be used in that fashion and have real benefit," says Pamela G. Robey, PhD, chief of the skeletal biology section at the National Institute of Dental and Craniofacial Research at the National Institutes of Health.
Robey should know—she headed the research team that discovered stem cells in human baby teeth and in wisdom teeth more than fifteen years ago. She believes prospects are better using these stem cells for tooth repair: research suggests they may be able to fix cracked teeth, repair bone defects caused by gum disease, or in root canal therapy, where they can be used to replace infected tissue with regenerated healthy pulp.
In the meantime, though, there are no clinical applications for MSCs. "These tooth banking companies aren't doing their own research," says Leigh Turner, a bioethicist at the University of Minnesota who monitors stem cell clinics. "They cobble together reports of early research in humans or from animal studies in an effort to provide a narrative to make it seem like it is evidence based."
Still, in all fairness, tooth banking companies aren't making the kind of extravagant claims made by stem cell clinics, which operate in a gray area of the law and purport to treat everything from chronic lung disease to Alzheimer's. "We don't know when therapies will be available using these cells because the pace of research is hard to predict," says Peter Verlander, PhD, a molecular geneticist and chief scientific officer of Provia Laboratories, the parent company of Store-A-Tooth. "But for parents who regretted not banking their child's cord blood, especially if they later develop a disease like diabetes, this is another opportunity."
But the jury is still out if this is truly a good investment. Moursi, a national spokesperson for the American Academy of Pediatric Dentistry who fields queries about this practice from a dozen or so families a year, concludes: "If you could afford it, and know the risks, benefits and current limitations, then it is something to consider."
The Death Predictor: A Helpful New Tool or an Ethical Morass?
A senior in hospice care.
Whenever Eric Karl Oermann has to tell a patient about a terrible prognosis, their first question is always: "how long do I have?" Oermann would like to offer a precise answer, to provide some certainty and help guide treatment. But although he's one of the country's foremost experts in medical artificial intelligence, Oermann is still dependent on a computer algorithm that's often wrong.
Doctors are notoriously terrible at guessing how long their patients will live.
Artificial intelligence, now often called deep learning or neural networks, has radically transformed language and image processing. It's allowed computers to play chess better than the world's grand masters and outwit the best Jeopardy players. But it still can't precisely tell a doctor how long a patient has left – or how to help that person live longer.
Someday, researchers predict, computers will be able to watch a video of a patient to determine their health status. Doctors will no longer have to spend hours inputting data into medical records. And computers will do a better job than specialists at identifying tiny tumors, impending crises, and, yes, figuring out how long the patient has to live. Oermann, a neurosurgeon at Mount Sinai, says all that technology will allow doctors to spend more time doing what they do best: talking with their patients. "I want to see more deep learning and computers in a clinical setting," he says, "so there can be more human interaction." But those days are still at least three to five years off, Oermann and other researchers say.
Doctors are notoriously terrible at guessing how long their patients will live, says Nigam Shah, an associate professor at Stanford University and assistant director of the school's Center for Biomedical Informatics Research. Doctors don't want to believe that their patient – whom they've come to like – will die. "Doctors over-estimate survival many-fold," Shah says. "How do you go into work, in say, oncology, and not be delusionally optimistic? You have to be."
But patients near the end of life will get better treatment – and even live longer – if they are overseen by hospice or palliative care, research shows. So, instead of relying on human bias to select those whose lives are nearing their end, Shah and his colleagues showed that they could use a deep learning algorithm based on medical records to flag incoming patients with a life expectancy of three months to a year. They use that data to indicate who might need palliative care. Then, the palliative care team can reach out to treating physicians proactively, instead of relying on their referrals or taking the time to read extensive medical charts.
But, although the system works well, Shah isn't yet sure if such indicators actually get the appropriate patients into palliative care. He's recently partnered with a palliative care doctor to run a gold-standard clinical trial to test whether patients who are flagged by this algorithm are indeed a better match for palliative care.
"What is effective from a health system perspective might not be effective from a treating physician's perspective and might not be effective from the patient's perspective," Shah notes. "I don't have a good way to guess everybody's reaction without actually studying it." Whether palliative care is appropriate, for instance, depends on more than just the patient's health status. "If the patient's not ready, the family's not ready and the doctor's not ready, then you're just banging your head against the wall," Shah says. "Given limited capacity, it's a waste of resources" to put that person in palliative care.
The algorithm isn't perfect, but "on balance, it leads to better decisions more often."
Alexander Smith and Sei Lee, both palliative care doctors, work together at the University of California, San Francisco, to develop predictions for patients who come to the hospital with a complicated prognosis or a history of decline. Their algorithm, they say, helps decide if this patient's problems – which might include diabetes, heart disease, a slow-growing cancer, and memory issues – make them eligible for hospice. The algorithm isn't perfect, they both agree, but "on balance, it leads to better decisions more often," Smith says.
Bethany Percha, an assistant professor at Mount Sinai, says that an algorithm may tell doctors that their patient is trending downward, but it doesn't do anything to change that trajectory. "Even if you can predict something, what can you do about it?" Algorithms may be able to offer treatment suggestions – but not what specific actions will alter a patient's future, says Percha, also the chief technology officer of Precise Health Enterprise, a product development group within Mount Sinai. And the algorithms remain challenging to develop. Electronic medical records may be great at her hospital, but if the patient dies at a different one, her system won't know. If she wants to be certain a patient has died, she has to merge social security records of death with her system's medical records – a time-consuming and cumbersome process.
An algorithm that learns from biased data will be biased, Shah says. Patients who are poor or African American historically have had worse health outcomes. If researchers train an algorithm on data that includes those biases, they get baked into the algorithms, which can then lead to a self-fulfilling prophesy. Smith and Lee say they've taken race out of their algorithms to avoid this bias.
Age is even trickier. There's no question that someone's risk of illness and death goes up with age. But an 85-year-old who breaks a hip running a marathon should probably be treated very differently than an 85-year-old who breaks a hip trying to get out of a chair in a dementia care unit. That's why the doctor can never be taken out of the equation, Shah says. Human judgment will always be required in medical care and an algorithm should never be followed blindly, he says.
Experts say that the flaws in artificial intelligence algorithms shouldn't prevent people from using them – carefully.
Researchers are also concerned that their algorithms will be used to ration care, or that insurance companies will use their data to justify a rate increase. If an algorithm predicts a patient is going to end up back in the hospital soon, "who's benefitting from knowing a patient is going to be readmitted? Probably the insurance company," Percha says.
Still, Percha and others say, the flaws in artificial intelligence algorithms shouldn't prevent people from using them – carefully. "These are new and exciting tools that have a lot of potential uses. We need to be conscious about how to use them going forward, but it doesn't mean we shouldn't go down this road," she says. "I think the potential benefits outweigh the risks, especially because we've barely scratched the surface of what big data can do right now."