Can Genetic Testing Help Shed Light on the Autism Epidemic?
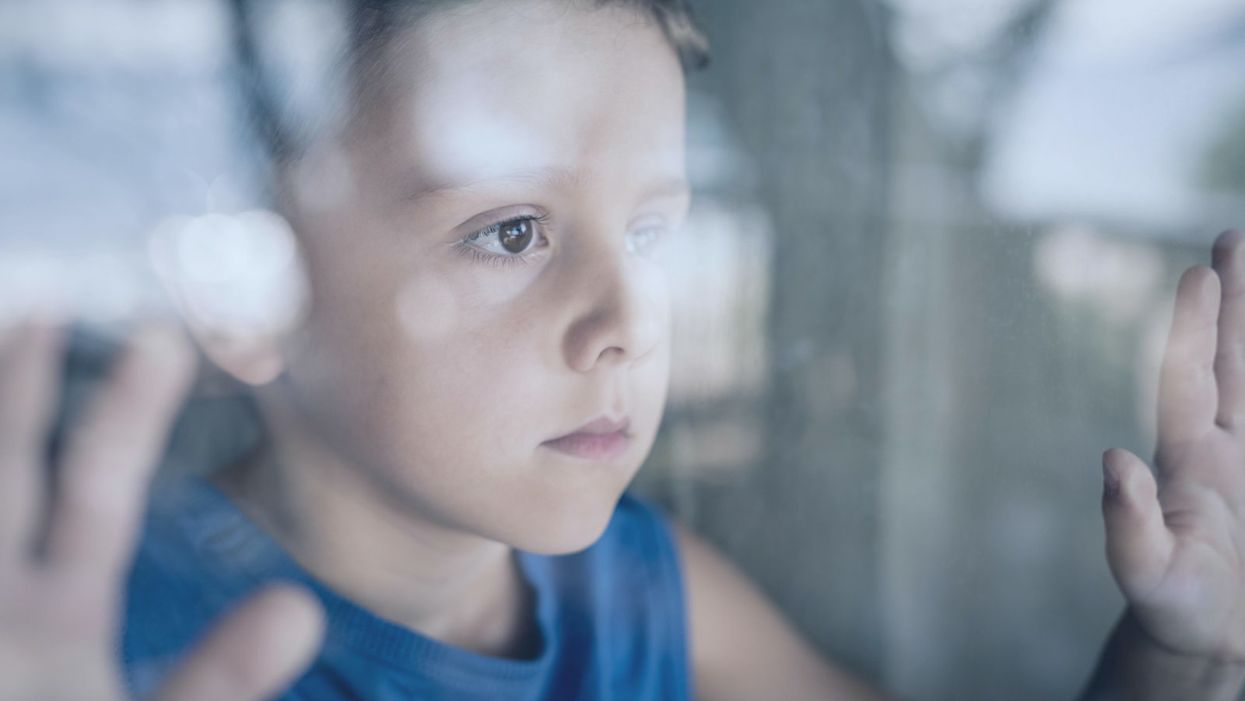
A little boy standing by a window in contemplation. (© altanaka/Fotolia)
Autism cases are still on the rise, and scientists don't know why. In April, the Centers for Disease Control (CDC) reported that rates of autism had increased once again, now at an estimated 1 in 59 children up from 1 in 68 just two years ago. Rates have been climbing steadily since 2007 when the CDC initially estimated that 1 in 150 children were on the autism spectrum.
Some clinicians are concerned that the creeping expansion of autism is causing the diagnosis to lose its meaning.
The standard explanation for this increase has been the expansion of the definition of autism to include milder forms like Asperger's, as well as a heightened awareness of the condition that has improved screening efforts. For example, the most recent jump is attributed to children in minority communities being diagnosed who might have previously gone under the radar. In addition, more federally funded resources are available to children with autism than other types of developmental disorders, which may prompt families or physicians to push harder for a diagnosis.
Some clinicians are concerned that the creeping expansion of autism is causing the diagnosis to lose its meaning. William Graf, a pediatric neurologist at Connecticut Children's Medical Center, says that when a nurse tells him that a new patient has a history of autism, the term is no longer a useful description. "Even though I know this topic extremely well, I cannot picture the child anymore," he says. "Use the words mild, moderate, or severe. Just give me a couple more clues, because when you say autism today, I have no idea what people are talking about anymore."
Genetic testing has emerged as one potential way to remedy the overly broad label by narrowing down a heterogeneous diagnosis to a specific genetic disorder. According to Suma Shankar, a medical geneticist at the University of California, Davis, up to 60 percent of autism cases could be attributed to underlying genetic causes. Common examples include Fragile X Syndrome or Rett Syndrome—neurodevelopmental disorders that are caused by mutations in individual genes and are behaviorally classified as autism.
With more than 500 different mutations associated with autism, very few additional diagnoses provide meaningful information.
Having a genetic diagnosis in addition to an autism diagnosis can help families in several ways, says Shankar. Knowing the genetic origin can alert families to other potential health problems that are linked to the mutation, such as heart defects or problems with the immune system. It may also help clinicians provide more targeted behavioral therapies and could one day lead to the development of drug treatments for underlying neurochemical abnormalities. "It will pave the way to begin to tease out treatments," Shankar says.
When a doctor diagnoses a child as having a specific genetic condition, the label of autism is still kept because it is more well-known and gives the child access to more state-funded resources. Children can thus be diagnosed with multiple conditions: autism spectrum disorder and their specific gene mutation. However, with more than 500 different mutations associated with autism, very few additional diagnoses provide meaningful information. What's more, the presence or absence of a mutation doesn't necessarily indicate whether the child is on the mild or severe end of the autism spectrum.
Because of this, Graf doubts that genetic classifications are really that useful. He tells the story of a boy with epilepsy and severe intellectual disabilities who was diagnosed with autism as a young child. Years later, Graf ordered genetic testing for the boy and discovered that he had a mutation in the gene SYNGAP1. However, this knowledge didn't change the boy's autism status. "That diagnosis [SYNGAP1] turns out to be very specific for him, but it will never be a household name. Biologically it's good to know, and now it's all over his chart. But on a societal level he still needs this catch-all label [of autism]," Graf says.
"It gives some information, but to what degree does that change treatment or prognosis?"
Jennifer Singh, a sociologist at Georgia Tech who wrote the book Multiple Autisms: Spectrums of Advocacy and Genomic Science, agrees. "I don't know that the knowledge gained from just having a gene that's linked to autism," is that beneficial, she says. "It gives some information, but to what degree does that change treatment or prognosis? Because at the end of the day you have to address the issues that are at hand, whatever they might be."
As more children are diagnosed with autism, knowledge of the underlying genetic mutation causing the condition could help families better understand the diagnosis and anticipate their child's developmental trajectory. However, for the vast majority, an additional label provides little clarity or consolation.
Instead of spending money on genetic screens, Singh thinks the resources would be better used on additional services for people who don't have access to behavioral, speech, or occupational therapy. "Things that are really going to matter for this child in their future," she says.
The Death Predictor: A Helpful New Tool or an Ethical Morass?
A senior in hospice care.
Whenever Eric Karl Oermann has to tell a patient about a terrible prognosis, their first question is always: "how long do I have?" Oermann would like to offer a precise answer, to provide some certainty and help guide treatment. But although he's one of the country's foremost experts in medical artificial intelligence, Oermann is still dependent on a computer algorithm that's often wrong.
Doctors are notoriously terrible at guessing how long their patients will live.
Artificial intelligence, now often called deep learning or neural networks, has radically transformed language and image processing. It's allowed computers to play chess better than the world's grand masters and outwit the best Jeopardy players. But it still can't precisely tell a doctor how long a patient has left – or how to help that person live longer.
Someday, researchers predict, computers will be able to watch a video of a patient to determine their health status. Doctors will no longer have to spend hours inputting data into medical records. And computers will do a better job than specialists at identifying tiny tumors, impending crises, and, yes, figuring out how long the patient has to live. Oermann, a neurosurgeon at Mount Sinai, says all that technology will allow doctors to spend more time doing what they do best: talking with their patients. "I want to see more deep learning and computers in a clinical setting," he says, "so there can be more human interaction." But those days are still at least three to five years off, Oermann and other researchers say.
Doctors are notoriously terrible at guessing how long their patients will live, says Nigam Shah, an associate professor at Stanford University and assistant director of the school's Center for Biomedical Informatics Research. Doctors don't want to believe that their patient – whom they've come to like – will die. "Doctors over-estimate survival many-fold," Shah says. "How do you go into work, in say, oncology, and not be delusionally optimistic? You have to be."
But patients near the end of life will get better treatment – and even live longer – if they are overseen by hospice or palliative care, research shows. So, instead of relying on human bias to select those whose lives are nearing their end, Shah and his colleagues showed that they could use a deep learning algorithm based on medical records to flag incoming patients with a life expectancy of three months to a year. They use that data to indicate who might need palliative care. Then, the palliative care team can reach out to treating physicians proactively, instead of relying on their referrals or taking the time to read extensive medical charts.
But, although the system works well, Shah isn't yet sure if such indicators actually get the appropriate patients into palliative care. He's recently partnered with a palliative care doctor to run a gold-standard clinical trial to test whether patients who are flagged by this algorithm are indeed a better match for palliative care.
"What is effective from a health system perspective might not be effective from a treating physician's perspective and might not be effective from the patient's perspective," Shah notes. "I don't have a good way to guess everybody's reaction without actually studying it." Whether palliative care is appropriate, for instance, depends on more than just the patient's health status. "If the patient's not ready, the family's not ready and the doctor's not ready, then you're just banging your head against the wall," Shah says. "Given limited capacity, it's a waste of resources" to put that person in palliative care.
The algorithm isn't perfect, but "on balance, it leads to better decisions more often."
Alexander Smith and Sei Lee, both palliative care doctors, work together at the University of California, San Francisco, to develop predictions for patients who come to the hospital with a complicated prognosis or a history of decline. Their algorithm, they say, helps decide if this patient's problems – which might include diabetes, heart disease, a slow-growing cancer, and memory issues – make them eligible for hospice. The algorithm isn't perfect, they both agree, but "on balance, it leads to better decisions more often," Smith says.
Bethany Percha, an assistant professor at Mount Sinai, says that an algorithm may tell doctors that their patient is trending downward, but it doesn't do anything to change that trajectory. "Even if you can predict something, what can you do about it?" Algorithms may be able to offer treatment suggestions – but not what specific actions will alter a patient's future, says Percha, also the chief technology officer of Precise Health Enterprise, a product development group within Mount Sinai. And the algorithms remain challenging to develop. Electronic medical records may be great at her hospital, but if the patient dies at a different one, her system won't know. If she wants to be certain a patient has died, she has to merge social security records of death with her system's medical records – a time-consuming and cumbersome process.
An algorithm that learns from biased data will be biased, Shah says. Patients who are poor or African American historically have had worse health outcomes. If researchers train an algorithm on data that includes those biases, they get baked into the algorithms, which can then lead to a self-fulfilling prophesy. Smith and Lee say they've taken race out of their algorithms to avoid this bias.
Age is even trickier. There's no question that someone's risk of illness and death goes up with age. But an 85-year-old who breaks a hip running a marathon should probably be treated very differently than an 85-year-old who breaks a hip trying to get out of a chair in a dementia care unit. That's why the doctor can never be taken out of the equation, Shah says. Human judgment will always be required in medical care and an algorithm should never be followed blindly, he says.
Experts say that the flaws in artificial intelligence algorithms shouldn't prevent people from using them – carefully.
Researchers are also concerned that their algorithms will be used to ration care, or that insurance companies will use their data to justify a rate increase. If an algorithm predicts a patient is going to end up back in the hospital soon, "who's benefitting from knowing a patient is going to be readmitted? Probably the insurance company," Percha says.
Still, Percha and others say, the flaws in artificial intelligence algorithms shouldn't prevent people from using them – carefully. "These are new and exciting tools that have a lot of potential uses. We need to be conscious about how to use them going forward, but it doesn't mean we shouldn't go down this road," she says. "I think the potential benefits outweigh the risks, especially because we've barely scratched the surface of what big data can do right now."
“Young Blood” Transfusions Are Not Ready For Primetime – Yet
A young woman donates blood.
The world of dementia research erupted into cheers when news of the first real victory in a clinical trial against Alzheimer's Disease in over a decade was revealed last October.
By connecting the circulatory systems of a young and an old mouse, the regenerative potential of the young mouse decreased, and the old mouse became healthier.
Alzheimer's treatments have been famously difficult to develop; 99 percent of the 200-plus such clinical trials since 2000 have utterly failed. Even the few slight successes have failed to produce what is called 'disease modifying' agents that really help people with the disease. This makes the success, by the midsize Spanish pharma company Grifols, worthy of special attention.
However, the specifics of the Grifols treatment, a process called plasmapheresis, are atypical for another reason - they did not give patients a small molecule or an elaborate gene therapy, but rather simply the most common component of normal human blood plasma, a protein called albumin. A large portion of the patients' normal plasma was removed, and then a sterile solution of albumin was infused back into them to keep their overall blood volume relatively constant.
So why does replacing Alzheimer's patients' plasma with albumin seem to help their brains? One theory is that the action is direct. Alzheimer's patients have low levels of serum albumin, which is needed to clear out the plaques of amyloid that slowly build up in the brain. Supplementing those patients with extra albumin boosts their ability to clear the plaques and improves brain health. However, there is also evidence suggesting that the problem may be something present in the plasma of the sick person and pulling their plasma out and replacing it with a filler, like an albumin solution, may be what creates the purported benefit.
This scientific question is the tip of an iceberg that goes far beyond Alzheimer's Disease and albumin, to a debate that has been waged on the pages of scientific journals about the secrets of using young, healthy blood to extend youth and health.
This debate started long before the Grifols data was released, in 2014 when a group of researchers at Stanford found that by connecting the circulatory systems of a young and an old mouse, the regenerative potential of the young mouse decreased, and the old mouse became healthier. There was something either present in young blood that allowed tissues to regenerate, or something present in old blood that prevented regeneration. Whatever the biological reason, the effects in the experiment were extraordinary, providing a startling boost in health in the older mouse.
After the initial findings, multiple research groups got to work trying to identify the "active factor" of regeneration (or the inhibitor of that regeneration). They soon uncovered a variety of compounds such as insulin-like growth factor 1 (IGF1), CCL11, and GDF11, but none seemed to provide all the answers researchers were hoping for, with a number of high-profile retractions based on unsound experimental practices, or inconclusive data.
Years of research later, the simplest conclusion is that the story of plasma regeneration is not simple - there isn't a switch in our blood we can flip to turn back our biological clocks. That said, these hypotheses are far from dead, and many researchers continue to explore the possibility of using the rejuvenating ability of youthful plasma to treat a variety of diseases of aging.
But the bold claims of improved vigor thanks to young blood are so far unsupported by clinical evidence.
The data remain intriguing because of the astounding results from the conjoined circulatory system experiments. The current surge in interest in studying the biology of aging is likely to produce a new crop of interesting results in the next few years. Both CCL11 and GDF11 are being researched as potential drug targets by two startups, Alkahest and Elevian, respectively.
Without clarity on a single active factor driving rejuvenation, it's tempting to try a simpler approach: taking actual blood plasma provided by young people and infusing it into elderly subjects. This is what at least one startup company, Ambrosia, is now offering in five commercial clinics across the U.S. -- for $8,000 a liter.
By using whole plasma, the idea is to sidestep our ignorance, reaping the benefits of young plasma transfusion without knowing exactly what the active factors are that make the treatment work in mice. This space has attracted both established players in the plasmapheresis field – Alkahest and Grifols have teamed up to test fractions of whole plasma in Alzheimer's and Parkinson's – but also direct-to-consumer operations like Ambrosia that just want to offer patients access to treatments without regulatory oversight.
But the bold claims of improved vigor thanks to young blood are so far unsupported by clinical evidence. We simply haven't performed trials to test whether dosing a mostly healthy person with plasma can slow down aging, at least not yet. There is some evidence that plasma replacement works in mice, yes, but those experiments are all done in very different systems than what a human receiving young plasma might experience. To date, I have not seen any plasma transfusion clinic doing young blood plasmapheresis propose a clinical trial that is anything more than a shallow advertisement for their procedures.
The efforts I have seen to perform prophylactic plasmapheresis will fail to impact societal health. Without clearly defined endpoints and proper clinical trials, we won't know whether the procedure really lowers the risk of disease or helps with conditions of aging. So even if their hypothesis is correct, the lack of strong evidence to fall back on means that the procedure will never spread beyond the fringe groups willing to take the risk. If their hypothesis is wrong, then people are paying a huge amount of money for false hope, just as they do, sadly, at the phony stem cell clinics that started popping up all through the 2000s when stem cell hype was at its peak.
Until then, prophylactic plasma transfusions will be the domain of the optimistic and the gullible.
The real progress in the field will be made slowly, using carefully defined products either directly isolated from blood or targeting a bloodborne factor, just as the serious pharma and biotech players are doing already.
The field will progress in stages, first creating and carefully testing treatments for well-defined diseases, and only then will it progress to large-scale clinical trials in relatively healthy people to look for the prevention of disease. Most of us will choose to wait for this second stage of trials before undergoing any new treatments. Until then, prophylactic plasma transfusions will be the domain of the optimistic and the gullible.