This App Helps Diagnose Rare Genetic Disorders from a Picture
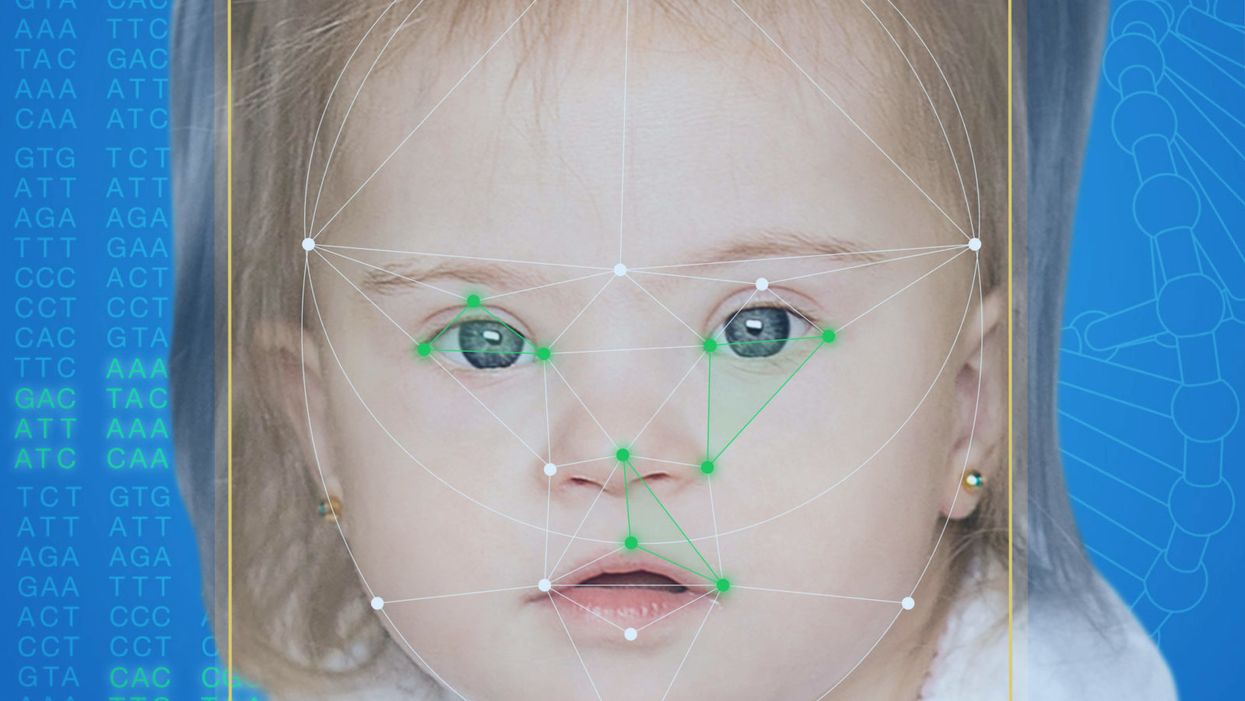
FDNA's Face2Gene technology analyzes patient biometric data using artificial intelligence, identifying correlations with disease-causing genetic variations.
Medical geneticist Omar Abdul-Rahman had a hunch. He thought that the three-year-old boy with deep-set eyes, a rounded nose, and uplifted earlobes might have Mowat-Wilson syndrome, but he'd never seen a patient with the rare disorder before.
"If it weren't for the app I'm not sure I would have had the confidence to say 'yes you should spend $1000 on this test."
Rahman had already ordered genetic tests for three different conditions without any luck, and he didn't want to cost the family any more money—or hope—if he wasn't sure of the diagnosis. So he took a picture of the boy and uploaded the photo to Face2Gene, a diagnostic aid for rare genetic disorders. Sure enough, Mowat-Wilson came up as a potential match. The family agreed to one final genetic test, which was positive for the syndrome.
"If it weren't for the app I'm not sure I would have had the confidence to say 'yes you should spend $1000 on this test,'" says Rahman, who is now the director of Genetic Medicine at the University of Nebraska Medical Center, but saw the boy when he was in the Department of Pediatrics at the University of Mississippi Medical Center in 2012.
"Families who are dealing with undiagnosed diseases never know what's going to come around the corner, what other organ system might be a problem next week," Rahman says. With a diagnosis, "You don't have to wait for the other shoe to drop because now you know the extent of the condition."
A diagnosis is the first and most important step for patients to attain medical care. Disease prognosis, treatment plans, and emotional coping all stem from this critical phase. But diagnosis can also be the trickiest part of the process, particularly for rare disorders. According to one European survey, 40 percent of rare diseases are initially misdiagnosed.
Healthcare professionals and medical technology companies hope that facial recognition software will help prevent families from facing difficult disruptions due to misdiagnoses.
"Patients with rare diseases or genetic disorders go through a long period of diagnostic odyssey, and just putting a name to a syndrome or finding a diagnosis can be very helpful and relieve a lot of tension for the family," says Dekel Gelbman, CEO of FDNA.
Consequently, a misdiagnosis can be devastating for families. Money and time may have been wasted on fruitless treatments, while opportunities for potentially helpful therapies or clinical trials were missed. Parents led down the wrong path must change their expectations of their child's long-term prognosis and care. In addition, they may be misinformed regarding future decisions about family planning.
Healthcare professionals and medical technology companies hope that facial recognition software will help prevent families from facing these difficult disruptions by improving the accuracy and ease of diagnosing genetic disorders. Traditionally, doctors diagnose these types of conditions by identifying unique patterns of facial features, a practice called dysmorphology. Trained physicians can read a child's face like a map and detect any abnormal ridges or plateaus—wide-set eyes, broad forehead, flat nose, rotated ears—that, combined with other symptoms such as intellectual disability or abnormal height and weight, signify a specific genetic disorder.
These morphological changes can be subtle, though, and often only specialized medical geneticists are able to detect and interpret these facial clues. What's more, some genetic disorders are so rare that even a specialist may not have encountered it before, much less a general practitioner. Diagnosing rare conditions has improved thanks to genomic testing that can confirm (or refute) a doctor's suspicion. Yet with thousands of variants in each person's genome, identifying the culprit mutation or deletion can be extremely difficult if you don't know what you're looking for.
Facial recognition technology is trying to take some of the guesswork out of this process. Software such as the Face2Gene app use machine learning to compare a picture of a patient against images of thousands of disorders and come back with suggestions of possible diagnoses.
"This is a classic field for artificial intelligence because no human being can really have enough knowledge and enough experience to be able to do this for thousands of different disorders."
"When we met a geneticist for the first time we were pretty blown away with the fact that they actually use their own human pattern recognition" to diagnose patients, says Gelbman. "This is a classic field for AI [artificial intelligence], for machine learning because no human being can really have enough knowledge and enough experience to be able to do this for thousands of different disorders."
When a physician uploads a photo to the app, they are given a list of different diagnostic suggestions, each with a heat map to indicate how similar the facial features are to a classic representation of the syndrome. The physician can hone the suggestions by adding in other symptoms or family history. Gelbman emphasized that the app is a "search and reference tool" and should not "be used to diagnose or treat medical conditions." It is not approved by the FDA as a diagnostic.
"As a tool, we've all been waiting for this, something that can help everyone," says Julian Martinez-Agosto, an associate professor in human genetics and pediatrics at UCLA. He sees the greatest benefit of facial recognition technology in its ability to empower non-specialists to make a diagnosis. Many areas, including rural communities or resource-poor countries, do not have access to either medical geneticists trained in these types of diagnostics or genomic screens. Apps like Face2Gene can help guide a general practitioner or flag diseases they might not be familiar with.
One concern is that most textbook images of genetic disorders come from the West, so the "classic" face of a condition is often a child of European descent.
Maximilian Muenke, a senior investigator at the National Human Genome Research Institute (NHGRI), agrees that in many countries, facial recognition programs could be the only way for a doctor to make a diagnosis.
"There are only geneticists in countries like the U.S., Canada, Europe, Japan. In most countries, geneticists don't exist at all," Muenke says. "In Nigeria, the most populous country in all of Africa with 160 million people, there's not a single clinical geneticist. So in a country like that, facial recognition programs will be sought after and will be extremely useful to help make a diagnosis to the non-geneticists."
One concern about providing this type of technology to a global population is that most textbook images of genetic disorders come from the West, so the "classic" face of a condition is often a child of European descent. However, the defining facial features of some of these disorders manifest differently across ethnicities, leaving clinicians from other geographic regions at a disadvantage.
"Every syndrome is either more easy or more difficult to detect in people from different geographic backgrounds," explains Muenke. For example, "in some countries of Southeast Asia, the eyes are slanted upward, and that happens to be one of the findings that occurs mostly with children with Down Syndrome. So then it might be more difficult for some individuals to recognize Down Syndrome in children from Southeast Asia."
There is a risk that providing this type of diagnostic information online will lead to parents trying to classify their own children.
To combat this issue, Muenke helped develop the Atlas of Human Malformation Syndromes, a database that incorporates descriptions and pictures of patients from every continent. By providing examples of rare genetic disorders in children from outside of the United States and Europe, Muenke hopes to provide clinicians with a better understanding of what to look for in each condition, regardless of where they practice.
There is a risk that providing this type of diagnostic information online will lead to parents trying to classify their own children. Face2Gene is free to download in the app store, although users must be authenticated by the company as a healthcare professional before they can access the database. The NHGRI Atlas can be accessed by anyone through their website. However, Martinez and Muenke say parents already use Google and WebMD to look up their child's symptoms; facial recognition programs and databases are just an extension of that trend. In fact, Martinez says, "Empowering families is another way to facilitate access to care. Some families live in rural areas and have no access to geneticists. If they can use software to get a diagnosis and then contact someone at a large hospital, it can help facilitate the process."
Martinez also says the app could go further by providing greater transparency about how the program makes its assessments. Giving clinicians feedback about why a diagnosis fits certain facial features would offer a valuable teaching opportunity in addition to a diagnostic aid.
Both Martinez and Muenke think the technology is an innovation that could vastly benefit patients. "In the beginning, I was quite skeptical and I could not believe that a machine could replace a human," says Muenke. "However, I am a convert that it actually can help tremendously in making a diagnosis. I think there is a place for facial recognition programs, and I am a firm believer that this will spread over the next five years."
Autonomous, indoor farming gives a boost to crops
Artificial Intelligence is already helping to grow some of the food we eat.
The glass-encased cabinet looks like a display meant to hold reasonably priced watches, or drugstore beauty creams shipped from France. But instead of this stagnant merchandise, each of its five shelves is overgrown with leaves — moss-soft pea sprouts, spikes of Lolla rosa lettuces, pale bok choy, dark kale, purple basil or red-veined sorrel or green wisps of dill. The glass structure isn’t a cabinet, but rather a “micro farm.”
The gadget is on display at the Richmond, Virginia headquarters of Babylon Micro-Farms, a company that aims to make indoor farming in the U.S. more accessible and sustainable. Babylon’s soilless hydroponic growing system, which feeds plants via nutrient-enriched water, allows chefs on cruise ships, cafeterias and elsewhere to provide home-grown produce to patrons, just seconds after it’s harvested. Currently, there are over 200 functioning systems, either sold or leased to customers, and more of them are on the way.
The chef-farmers choose from among 45 types of herb and leafy-greens seeds, plop them into grow trays, and a few weeks later they pick and serve. While success is predicated on at least a small amount of these humans’ care, the systems are autonomously surveilled round-the-clock from Babylon’s base of operations. And artificial intelligence is helping to run the show.
Babylon piloted the use of specialized cameras that take pictures in different spectrums to gather some less-obvious visual data about plants’ wellbeing and alert people if something seems off.
Imagine consistently perfect greens and tomatoes and strawberries, grown hyper-locally, using less water, without chemicals or environmental contaminants. This is the hefty promise of controlled environment agriculture (CEA) — basically, indoor farms that can be hydroponic, aeroponic (plant roots are suspended and fed through misting), or aquaponic (where fish play a role in fertilizing vegetables). But whether they grow 4,160 leafy-green servings per year, like one Babylon farm, or millions of servings, like some of the large, centralized facilities starting to supply supermarkets across the U.S., they seek to minimize failure as much as possible.
Babylon’s soilless hydroponic growing system
Courtesy Babylon Micro-Farms
Here, AI is starting to play a pivotal role. CEA growers use it to help “make sense of what’s happening” to the plants in their care, says Scott Lowman, vice president of applied research at the Institute for Advanced Learning and Research (IALR) in Virginia, a state that’s investing heavily in CEA companies. And although these companies say they’re not aiming for a future with zero human employees, AI is certainly poised to take a lot of human farming intervention out of the equation — for better and worse.
Most of these companies are compiling their own data sets to identify anything that might block the success of their systems. Babylon had already integrated sensor data into its farms to measure heat and humidity, the nutrient content of water, and the amount of light plants receive. Last year, they got a National Science Foundation grant that allowed them to pilot the use of specialized cameras that take pictures in different spectrums to gather some less-obvious visual data about plants’ wellbeing and alert people if something seems off. “Will this plant be healthy tomorrow? Are there things…that the human eye can't see that the plant starts expressing?” says Amandeep Ratte, the company’s head of data science. “If our system can say, Hey, this plant is unhealthy, we can reach out to [users] preemptively about what they’re doing wrong, or is there a disease at the farm?” Ratte says. The earlier the better, to avoid crop failures.
Natural light accounts for 70 percent of Greenswell Growers’ energy use on a sunny day.
Courtesy Greenswell Growers
IALR’s Lowman says that other CEA companies are developing their AI systems to account for the different crops they grow — lettuces come in all shapes and sizes, after all, and each has different growing needs than, for example, tomatoes. The ways they run their operations differs also. Babylon is unusual in its decentralized structure. But centralized growing systems with one main location have variabilities, too. AeroFarms, which recently declared bankruptcy but will continue to run its 140,000-square foot vertical operation in Danville, Virginia, is entirely enclosed and reliant on the intense violet glow of grow lights to produce microgreens.
Different companies have different data needs. What data is essential to AeroFarms isn’t quite the same as for Greenswell Growers located in Goochland County, Virginia. Raising four kinds of lettuce in a 77,000-square-foot automated hydroponic greenhouse, the vagaries of naturally available light, which accounts for 70 percent of Greenswell’s energy use on a sunny day, affect operations. Their tech needs to account for “outside weather impacts,” says president Carl Gupton. “What adjustments do we have to make inside of the greenhouse to offset what's going on outside environmentally, to give that plant optimal conditions? When it's 85 percent humidity outside, the system needs to do X, Y and Z to get the conditions that we want inside.”
AI will help identify diseases, as well as when a plant is thirsty or overly hydrated, when it needs more or less calcium, phosphorous, nitrogen.
Nevertheless, every CEA system has the same core needs — consistent yield of high quality crops to keep up year-round supply to customers. Additionally, “Everybody’s got the same set of problems,” Gupton says. Pests may come into a facility with seeds. A disease called pythium, one of the most common in CEA, can damage plant roots. “Then you have root disease pressures that can also come internally — a change in [growing] substrate can change the way the plant performs,” Gupton says.
AI will help identify diseases, as well as when a plant is thirsty or overly hydrated, when it needs more or less calcium, phosphorous, nitrogen. So, while companies amass their own hyper-specific data sets, Lowman foresees a time within the next decade “when there will be some type of [open-source] database that has the most common types of plant stress identified” that growers will be able to tap into. Such databases will “create a community and move the science forward,” says Lowman.
In fact, IALR is working on assembling images for just such a database now. On so-called “smart tables” inside an Institute lab, a team is growing greens and subjects them to various stressors. Then, they’re administering treatments while taking images of every plant every 15 minutes, says Lowman. Some experiments generate 80,000 images; the challenge lies in analyzing and annotating the vast trove of them, marking each one to reflect outcome—for example increasing the phosphate delivery and the plant’s response to it. Eventually, they’ll be fed into AI systems to help them learn.
For all the enthusiasm surrounding this technology, it’s not without downsides. Training just one AI system can emit over 250,000 pounds of carbon dioxide, according to MIT Technology Review. AI could also be used “to enhance environmental benefit for CEA and optimize [its] energy consumption,” says Rozita Dara, a computer science professor at the University of Guelph in Canada, specializing in AI and data governance, “but we first need to collect data to measure [it].”
The chef-farmers can choose from 45 types of herb and leafy-greens seeds.
Courtesy Babylon Micro-Farms
Any system connected to the Internet of Things is also vulnerable to hacking; if CEA grows to the point where “there are many of these similar farms, and you're depending on feeding a population based on those, it would be quite scary,” Dara says. And there are privacy concerns, too, in systems where imaging is happening constantly. It’s partly for this reason, says Babylon’s Ratte, that the company’s in-farm cameras all “face down into the trays, so the only thing [visible] is pictures of plants.”
Tweaks to improve AI for CEA are happening all the time. Greenswell made its first harvest in 2022 and now has annual data points they can use to start making more intelligent choices about how to feed, water, and supply light to plants, says Gupton. Ratte says he’s confident Babylon’s system can already “get our customers reliable harvests. But in terms of how far we have to go, it's a different problem,” he says. For example, if AI could detect whether the farm is mostly empty—meaning the farm’s user hasn’t planted a new crop of greens—it can alert Babylon to check “what's going on with engagement with this user?” Ratte says. “Do they need more training? Did the main person responsible for the farm quit?”
Lowman says more automation is coming, offering greater ability for systems to identify problems and mitigate them on the spot. “We still have to develop datasets that are specific, so you can have a very clear control plan, [because] artificial intelligence is only as smart as what we tell it, and in plant science, there's so much variation,” he says. He believes AI’s next level will be “looking at those first early days of plant growth: when the seed germinates, how fast it germinates, what it looks like when it germinates.” Imaging all that and pairing it with AI, “can be a really powerful tool, for sure.”
Scientists make progress with growing organs for transplants
Researchers from the University of Cambridge have laid the foundations for growing synthetic embryos that could develop a beating heart, gut and brain.
Story by Big Think
For over a century, scientists have dreamed of growing human organs sans humans. This technology could put an end to the scarcity of organs for transplants. But that’s just the tip of the iceberg. The capability to grow fully functional organs would revolutionize research. For example, scientists could observe mysterious biological processes, such as how human cells and organs develop a disease and respond (or fail to respond) to medication without involving human subjects.
Recently, a team of researchers from the University of Cambridge has laid the foundations not just for growing functional organs but functional synthetic embryos capable of developing a beating heart, gut, and brain. Their report was published in Nature.
The organoid revolution
In 1981, scientists discovered how to keep stem cells alive. This was a significant breakthrough, as stem cells have notoriously rigorous demands. Nevertheless, stem cells remained a relatively niche research area, mainly because scientists didn’t know how to convince the cells to turn into other cells.
Then, in 1987, scientists embedded isolated stem cells in a gelatinous protein mixture called Matrigel, which simulated the three-dimensional environment of animal tissue. The cells thrived, but they also did something remarkable: they created breast tissue capable of producing milk proteins. This was the first organoid — a clump of cells that behave and function like a real organ. The organoid revolution had begun, and it all started with a boob in Jello.
For the next 20 years, it was rare to find a scientist who identified as an “organoid researcher,” but there were many “stem cell researchers” who wanted to figure out how to turn stem cells into other cells. Eventually, they discovered the signals (called growth factors) that stem cells require to differentiate into other types of cells.
For a human embryo (and its organs) to develop successfully, there needs to be a “dialogue” between these three types of stem cells.
By the end of the 2000s, researchers began combining stem cells, Matrigel, and the newly characterized growth factors to create dozens of organoids, from liver organoids capable of producing the bile salts necessary for digesting fat to brain organoids with components that resemble eyes, the spinal cord, and arguably, the beginnings of sentience.
Synthetic embryos
Organoids possess an intrinsic flaw: they are organ-like. They share some characteristics with real organs, making them powerful tools for research. However, no one has found a way to create an organoid with all the characteristics and functions of a real organ. But Magdalena Żernicka-Goetz, a developmental biologist, might have set the foundation for that discovery.
Żernicka-Goetz hypothesized that organoids fail to develop into fully functional organs because organs develop as a collective. Organoid research often uses embryonic stem cells, which are the cells from which the developing organism is created. However, there are two other types of stem cells in an early embryo: stem cells that become the placenta and those that become the yolk sac (where the embryo grows and gets its nutrients in early development). For a human embryo (and its organs) to develop successfully, there needs to be a “dialogue” between these three types of stem cells. In other words, Żernicka-Goetz suspected the best way to grow a functional organoid was to produce a synthetic embryoid.
As described in the aforementioned Nature paper, Żernicka-Goetz and her team mimicked the embryonic environment by mixing these three types of stem cells from mice. Amazingly, the stem cells self-organized into structures and progressed through the successive developmental stages until they had beating hearts and the foundations of the brain.
“Our mouse embryo model not only develops a brain, but also a beating heart [and] all the components that go on to make up the body,” said Żernicka-Goetz. “It’s just unbelievable that we’ve got this far. This has been the dream of our community for years and major focus of our work for a decade and finally we’ve done it.”
If the methods developed by Żernicka-Goetz’s team are successful with human stem cells, scientists someday could use them to guide the development of synthetic organs for patients awaiting transplants. It also opens the door to studying how embryos develop during pregnancy.
This article originally appeared on Big Think, home of the brightest minds and biggest ideas of all time.