The Algorithm Will See You Now
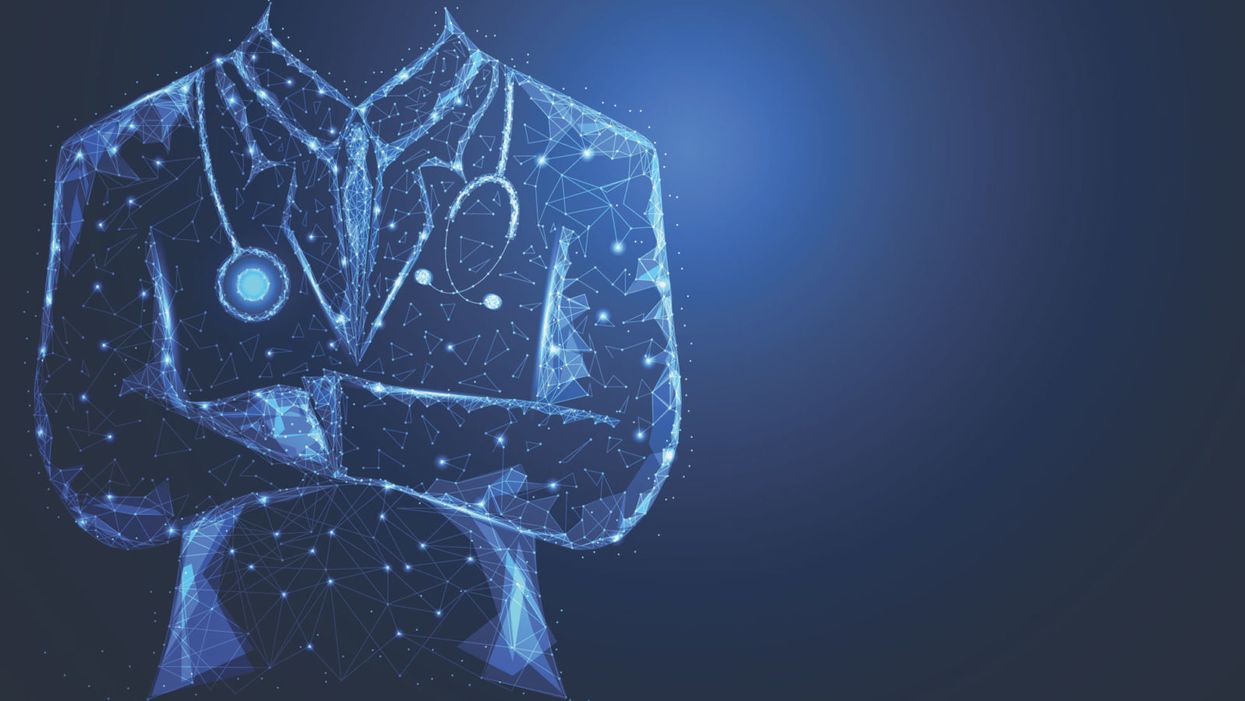
Artificial intelligence in medicine, still in an early phase, stands to transform how doctors and nurses spend their time.
There's a quiet revolution going on in medicine. It's driven by artificial intelligence, but paradoxically, new technology may put a more human face on healthcare.
AI's usefulness in healthcare ranges far and wide.
Artificial intelligence is software that can process massive amounts of information and learn over time, arriving at decisions with striking accuracy and efficiency. It offers greater accuracy in diagnosis, exponentially faster genome sequencing, the mining of medical literature and patient records at breathtaking speed, a dramatic reduction in administrative bureaucracy, personalized medicine, and even the democratization of healthcare.
The algorithms that bring these advantages won't replace doctors; rather, by offloading some of the most time-consuming tasks in healthcare, providers will be able to focus on personal interactions with patients—listening, empathizing, educating and generally putting the care back in healthcare. The relationship can focus on the alleviation of suffering, both the physical and emotional kind.
Challenges of Getting AI Up and Running
The AI revolution, still in its early phase in medicine, is already spurring some amazing advances, despite the fact that some experts say it has been overhyped. IBM's Watson Health program is a case in point. IBM capitalized on Watson's ability to process natural language by designing algorithms that devour data like medical articles and analyze images like MRIs and medical slides. The algorithms help diagnose diseases and recommend treatment strategies.
But Technology Review reported that a heavily hyped partnership with the MD Anderson Cancer Center in Houston fell apart in 2017 because of a lack of data in the proper format. The data existed, just not in a way that the voraciously data-hungry AI could use to train itself.
The hiccup certainly hasn't dampened the enthusiasm for medical AI among other tech giants, including Google and Apple, both of which have invested billions in their own healthcare projects. At this point, the main challenge is the need for algorithms to interpret a huge diversity of data mined from medical records. This can include everything from CT scans, MRIs, electrocardiograms, x-rays, and medical slides, to millions of pages of medical literature, physician's notes, and patient histories. It can even include data from implantables and wearables such as the Apple Watch and blood sugar monitors.
None of this information is in anything resembling a standard format across and even within hospitals, clinics, and diagnostic centers. Once the algorithms are trained, however, they can crunch massive amounts of data at blinding speed, with an accuracy that matches and sometimes even exceeds that of highly experienced doctors.
Genome sequencing, for example, took years to accomplish as recently as the early 2000s. The Human Genome Project, the first sequencing of the human genome, was an international effort that took 13 years to complete. In April of this year, Rady Children's Institute for Genomic Medicine in San Diego used an AI-powered genome sequencing algorithm to diagnose rare genetic diseases in infants in about 20 hours, according to ScienceDaily.
"Patient care will always begin and end with the doctor."
Dr. Stephen Kingsmore, the lead author of an article published in Science Translational Medicine, emphasized that even though the algorithm helped guide the treatment strategies of neonatal intensive care physicians, the doctor was still an indispensable link in the chain. "Some people call this artificial intelligence, we call it augmented intelligence," he says. "Patient care will always begin and end with the doctor."
One existing trend is helping to supply a great amount of valuable data to algorithms—the electronic health record. Initially blamed for exacerbating the already crushing workload of many physicians, the EHR is emerging as a boon for algorithms because it consolidates all of a patient's data in one record.
Examples of AI in Action Around the Globe
If you're a parent who has ever taken a child to the doctor with flulike symptoms, you know the anxiety of wondering if the symptoms signal something serious. Kang Zhang, M.D., Ph.D., the founding director of the Institute for Genomic Medicine at the University of California at San Diego, and colleagues developed an AI natural language processing model that used deep learning to analyze the EHRs of 1.3 million pediatric visits to a clinic in Guanzhou, China.
The AI identified common childhood diseases with about the same accuracy as human doctors, and it was even able to split the diagnoses into two categories—common conditions such as flu, and serious, life-threatening conditions like meningitis. Zhang has emphasized that the algorithm didn't replace the human doctor, but it did streamline the diagnostic process and could be used in a triage capacity when emergency room personnel need to prioritize the seriously ill over those suffering from common, less dangerous ailments.
AI's usefulness in healthcare ranges far and wide. In Uganda and several other African nations, AI is bringing modern diagnostics to remote villages that have no access to traditional technologies such as x-rays. The New York Times recently reported that there, doctors are using a pocket-sized, hand-held ultrasound machine that works in concert with a cell phone to image and diagnose everything from pneumonia (a common killer of children) to cancerous tumors.
The beauty of the highly portable, battery-powered device is that ultrasound images can be uploaded on computers so that physicians anywhere in the world can review them and weigh in with their advice. And the images are instantly incorporated into the patient's EHR.
Jonathan Rothberg, the founder of Butterfly Network, the Connecticut company that makes the device, told The New York Times that "Two thirds of the world's population gets no imaging at all. When you put something on a chip, the price goes down and you democratize it." The Butterfly ultrasound machine, which sells for $2,000, promises to be a game-changer in remote areas of Africa, South America, and Asia, as well as at the bedsides of patients in developed countries.
AI algorithms are rapidly emerging in healthcare across the U.S. and the world. China has become a major international player, set to surpass the U.S. this year in AI capital investment, the translation of AI research into marketable products, and even the number of often-cited research papers on AI. So far the U.S. is still the leader, but some experts describe the relationship between the U.S. and China as an AI cold war.
"The future of machine learning isn't sentient killer robots. It's longer human lives."
The U.S. Food and Drug Administration expanded its approval of medical algorithms from two in all of 2017 to about two per month throughout 2018. One of the first fields to be impacted is ophthalmology.
One algorithm, developed by the British AI company DeepMind (owned by Alphabet, the parent company of Google), instantly scans patients' retinas and is able to diagnose diabetic retinopathy without needing an ophthalmologist to interpret the scans. This means diabetics can get the test every year from their family physician without having to see a specialist. The Financial Times reported in March that the technology is now being used in clinics throughout Europe.
In Copenhagen, emergency service dispatchers are using a new voice-processing AI called Corti to analyze the conversations in emergency phone calls. The algorithm analyzes the verbal cues of callers, searches its huge database of medical information, and provides dispatchers with onscreen diagnostic information. Freddy Lippert, the CEO of EMS Copenhagen, notes that the algorithm has already saved lives by expediting accurate diagnoses in high-pressure situations where time is of the essence.
Researchers at the University of Nottingham in the UK have even developed a deep learning algorithm that predicts death more accurately than human clinicians. The algorithm incorporates data from a huge range of factors in a chronically ill population, including how many fruits and vegetables a patient eats on a daily basis. Dr. Stephen Weng, lead author of the study, published in PLOS ONE, said in a press release, "We found machine learning algorithms were significantly more accurate in predicting death than the standard prediction models developed by a human expert."
New digital technologies are allowing patients to participate in their healthcare as never before. A feature of the new Apple Watch is an app that detects cardiac arrhythmias and even produces an electrocardiogram if an abnormality is detected. The technology, approved by the FDA, is helping cardiologists monitor heart patients and design interventions for those who may be at higher risk of a cardiac event like a stroke.
If having an algorithm predict your death sends a shiver down your spine, consider that algorithms may keep you alive longer. In 2018, technology reporter Tristan Greene wrote for Medium that "…despite the unending deluge of panic-ridden articles declaring AI the path to apocalypse, we're now living in a world where algorithms save lives every day. The future of machine learning isn't sentient killer robots. It's longer human lives."
The Risks of AI Compiling Your Data
To be sure, the advent of AI-infused medical technology is not without its risks. One risk is that the use of AI wearables constantly monitoring our vital signs could turn us into a nation of hypochondriacs, racing to our doctors every time there's a blip in some vital sign. Such a development could stress an already overburdened system that suffers from, among other things, a shortage of doctors and nurses. Another risk has to do with the privacy protections on the massive repository of intimately personal information that AI will have on us.
In an article recently published in the Journal of the American Medical Association, Australian researcher Kit Huckvale and colleagues examined the handling of data by 36 smartphone apps that assisted people with either depression or smoking cessation, two areas that could lend themselves to stigmatization if they fell into the wrong hands.
Out of the 36 apps, 33 shared their data with third parties, despite the fact that just 25 of those apps had a privacy policy at all and out of those, only 23 stated that data would be shared with third parties. The recipients of all that data? It went almost exclusively to Facebook and Google, to be used for advertising and marketing purposes. But there's nothing to stop it from ending up in the hands of insurers, background databases, or any other entity.
Even when data isn't voluntarily shared, any digital information can be hacked. EHRs and even wearable devices share the same vulnerability as any other digital record or device. Still, the promise of AI to radically improve efficiency and accuracy in healthcare is hard to ignore.
AI Can Help Restore Humanity to Medicine
Eric Topol, director of the Scripps Research Translational Institute and author of the new book Deep Medicine, says that AI gives doctors and nurses the most precious gift of all: time.
Topol welcomes his patients' use of the Apple Watch cardiac feature and is optimistic about the ways that AI is revolutionizing medicine. He says that the watch helps doctors monitor how well medications are working and has already helped to prevent strokes. But in addition to that, AI will help bring the humanity back to a profession that has become as cold and hard as a stainless steel dissection table.
"When I graduated from medical school in the 1970s," he says, "you had a really intimate relationship with your doctor." Over the decades, he has seen that relationship steadily erode as medical organizations demanded that doctors see more and more patients within ever-shrinking time windows.
"Doctors have no time to think, to communicate. We need to restore the mission in medicine."
In addition to that, EHRs have meant that doctors and nurses are getting buried in paperwork and administrative tasks. This is no doubt one reason why a recent study by the World Health Organization showed that worldwide, about 50 percent of doctors suffer from burnout. People who are utterly exhausted make more mistakes, and medical clinicians are no different from the rest of us. Only medical mistakes have unacceptably high stakes. According to its website, Johns Hopkins University recently announced that in the U.S. alone, 250,000 people die from medical mistakes each year.
"Doctors have no time to think, to communicate," says Topol. "We need to restore the mission in medicine." AI is giving doctors more time to devote to the thing that attracted them to medicine in the first place—connecting deeply with patients.
There is a real danger at this juncture, though, that administrators aware of the time-saving aspects of AI will simply push doctors to see more patients, read more tests, and embrace an even more crushing workload.
"We can't leave it to the administrators to just make things worse," says Topol. "Now is the time for doctors to advocate for a restoration of the human touch. We need to stand up for patients and for the patient-doctor relationship."
AI could indeed be a game changer, he says, but rather than squander the huge benefits of more time, "We need a new equation going forward."
7 Things to Know about the U.S.’s Capability to Detect Omicron
A visualization of the original coronavirus causing COVID-19, which has now been detected across the globe in a highly mutated form called Omicron.
If the new variant Omicron isn’t here already – which many experts suspect that it is – it will be soon. While we wait for scientists to conduct the necessary research to characterize its transmissibility, potential fitness at immune evasion, and disease severity, we wanted to give Leaps.org readers a window into how the U.S. is positioned to detect the variant. So we spoke to Kelly Wroblewski, director of infectious diseases at the Association of Public Health Laboratories, a membership organization that represents state and local government health labs in the United States. Here are seven insights she shared.
1) If you test positive for COVID-19 with a standard PCR test, the diagnostic report will not tell you which variant you have. There are no diagnostic tests available for your doctor to order to identify variants. To find out the variant, the specimen must be sent to a commercial, clinical, academic, or public health laboratory for genetic sequencing.
2) Today, the U.S. sequences about 5 to 10 percent of all diagnostic specimens that test positive for SARS-CoV-2 in order to determine which variants are circulating and where. Last week nationally, for example, labs sequenced about 80,000 samples. This represents a massive increase from last year at this time, when labs were only sequencing about 8,000 specimens per week. Currently, 99.5 percent of circulating SARS-CoV-2 virus in the U.S. is the Delta variant.
3) The U.S. is “very well prepared” to detect Omicron, Wroblewski says, “particularly compared to where we were when the Alpha variant, or B117 first emerged.” Of the hunt for Omicron, she adds, “it’s very reminiscent of that time, except we are doing so much more sequencing and we have so much better coverage with our sequencing geographically, and we're doing it in a much more timely way. We have the ability to find emerging variants that are circulating in 0.01 percent of the population.”
4) Deciding which specimens to sample is not totally random. Samples that have more virus are likely to lead to better sequencing results. Labs also look to have a diverse set of representative samples, meaning across geographic regions and across gender, race, ethnicity, and age groups. Clinical diversity is also important, such as including pregnant women, severe in-patient cases, mild cases, etc.
5) Sequencing more is not necessarily better to find Omicron faster. “We will increase the number of sequences to a certain extent,” Wroblewski says. “Where we exhibit some caution is doing that indiscriminately isn’t the most effective use of time and resources. The important thing is to try to find Omicron, and if you increase your testing capacity too much, right now, it's still predominantly Delta in the U.S. by a long shot. So you’re mostly going to sequence Delta and you run the risk of delaying your discovery of Omicron, if you focus solely on increasing sequencing.”
So besides just ramping up the sheer numbers of sequencing, diagnostic labs across the country are now advised to preferentially use a certain PCR test made by Thermo Fisher that can help hasten the detection of Omicron. It turns out that Omicron’s specific mutations in the Spike protein mean that the Spike is not picked up on this PCR test, which yields a type of result called an S-gene target failure. Yet the test will still accurately pick up a COVID-19 diagnosis, because it detects two other gene targets on Omicron that are not mutated. “That S-gene target failure gives you a good indication that you may have Omicron. It’s a good early screen.”
Labs will then still need to sequence the whole genome to confirm it matches the Omicron sequence. “So right now, the new recommendation is to use [the Thermo Fisher test] as much as possible to give us a better chance of detecting Omicron more quickly.”
6) This Thermo Fisher test is “fairly widely used” in the U.S. already, so many labs are already well positioned to make the shift. “In early to mid 2020,” Wroblewski explains, “when the supply chain issue for testing was acute, many public health labs implemented five, six, seven, eight different tests, just so they could get enough supplies to do all the testing. Now that we're in a much better place supply-chain wise, it's very difficult and time consuming and cumbersome to maintain all those different test methods all the time, and many, many labs scaled back to only one or two. And so this [new recommendation] would just be shifting to two for some labs that will be shifting to them.”
7) Once Omicron is found here, labs will be focused on finding as many cases as possible, and the CDC will be conducting a variety of studies to determine the impact of the variant on diagnostics, therapeutics, and vaccines. Epidemiologists at the local, state, and federal level will analyze which populations it is spreading in, as well as the severity of the disease it causes. They will work to sort out different impacts on vaccinated vs. unvaccinated populations. The ultimate goal, Wroblewski concludes, is to “use all of that information to make better public health decisions and inform the public about what’s going on.”
Kira Peikoff was the editor-in-chief of Leaps.org from 2017 to 2021. As a journalist, her work has appeared in The New York Times, Newsweek, Nautilus, Popular Mechanics, The New York Academy of Sciences, and other outlets. She is also the author of four suspense novels that explore controversial issues arising from scientific innovation: Living Proof, No Time to Die, Die Again Tomorrow, and Mother Knows Best. Peikoff holds a B.A. in Journalism from New York University and an M.S. in Bioethics from Columbia University. She lives in New Jersey with her husband and two young sons. Follow her on Twitter @KiraPeikoff.
The Inside Story of Two Young Scientists Who Helped Make Moderna's Covid Vaccine Possible
Scientists Jason Schrum and Kerry Benenato solved crucial challenges in mRNA vaccine development.
In early 2020, Moderna Inc. was a barely-known biotechnology company with an unproven approach. It wanted to produce messenger RNA molecules to carry instructions into the body, teaching it to ward off disease. Experts doubted the Boston-based company would meet success.
Today, Moderna is a pharmaceutical power thanks to its success developing an effective Covid-19 vaccine. The company is worth $124 billion, more than giants including GlaxoSmithKline and Sanofi, and evidence has emerged that Moderna's shots are more protective than those produced by Pfizer-BioNTech and other vaccine makers. Pressure is building on the company to deliver more of its doses to people around the world, especially in poorer countries, and Moderna is working on vaccines against other pathogens, including Zika, influenza and cytomegalovirus.
But Moderna encountered such difficulties over the course of its eleven-year history that some executives worried it wouldn't survive. Two unlikely scientists helped save the company. Their breakthroughs paved the way for Moderna's Covid-19 shots but their work has never been publicized nor have their contributions been properly appreciated.
Derrick Rossi, a scientist at MIT, and Noubar Afeyan, a Cambridge-based investor, launched Moderna in September 2010. Their idea was to create mRNA molecules capable of delivering instructions to the body's cells, directing them to make proteins to heal ailments and cure disease. Need a statin, immunosuppressive, or other drug or vaccine? Just use mRNA to send a message to the body's cells to produce it. Rossi and Afeyan were convinced injecting mRNA into the body could turn it into its own laboratory, generating specific medications or vaccines as needed.
At the time, the notion that one might be able to teach the body to make proteins bordered on heresy. Everyone knew mRNA was unstable and set off the body's immune system on its way into cells. But in the late 2000's, two scientists at the University of Pennsylvania, Katalin Karikó and Drew Weissman, had figured out how to modify mRNA's chemical building blocks so the molecule could escape the notice of the immune system and enter the cell. Rossi and Afeyan couldn't convince the University of Pennsylvania to license Karikó and Weissman's patent, however, stymying Moderna's early ambitions. At the same time, the Penn scientists' technique seemed more applicable to an academic lab than a biotech company that needed to produce drugs or shots consistently and in bulk. Rossi and Afeyan's new company needed their own solution to help mRNA evade the body's defenses.
Some of Moderna's founders doubted Schrum could find success and they worried if their venture was doomed from the start.
The Scientist Who Modified mRNA: Jason Schrum
In 2010, Afeyan's firm subleased laboratory space in the basement of another Cambridge biotech company to begin scientific work. Afeyan chose a young scientist on his staff, Jason Schrum, to be Moderna's first employee, charging him with getting mRNA into cells without relying on Karikó and Weissman's solutions.
Schrum seemed well suited for the task. Months earlier, he had received a PhD in biological chemistry at Harvard University, where he had focused on nucleotide chemistry. Schrum even had the look of someone who might do big things. The baby-faced twenty-eight-year-old favored a relaxed, start-up look: khakis, button-downs, and Converse All-Stars.
Schrum felt immediate strain, however. He hadn't told anyone, but he was dealing with intense pain in his hands and joints, a condition that later would be diagnosed as degenerative arthritis. Soon Schrum couldn't bend two fingers on his left hand, making lab work difficult. He joined a drug trial, but the medicine proved useless. Schrum tried corticosteroid injections and anti-inflammatory drugs, but his left hand ached, restricting his experiments.
"It just wasn't useful," Schrum says, referring to his tender hand.*
He persisted, nonetheless. Each day in the fall of 2010, Schrum walked through double air-locked doors into a sterile "clean room" before entering a basement laboratory, in the bowels of an office in Cambridge's Kendall Square neighborhood, where he worked deep into the night. Schrum searched for potential modifications of mRNA nucleosides, hoping they might enable the molecule to produce proteins. Like all such rooms, there were no windows, so Schrum had to check a clock to know if it was day or night. A colleague came to visit once in a while, but most of the time, Schrum was alone.
Some of Moderna's founders doubted Schrum could find success and they worried if their venture was doomed from the start. An established MIT scientist turned down a job with the start-up to join pharmaceutical giant Novartis, dubious of Moderna's approach. Colleagues wondered if mRNA could produce proteins, at least on a consistent basis.
As Schrum began testing the modifications in January 2011, he made an unexpected discovery. Karikó and Weissman saw that by turned one of the building blocks for mRNA, a ribonucleoside called uridine, into a slightly different form called pseudouridine, the cell's immune system ignored the mRNA and the molecule avoided an immune response. After a series of experiments in the basement lab, Schrum discovered that a variant of pseudouridine called N1- methyl-pseudouridine did an even better job reducing the cell's innate immune response. Schrum's nucleoside switch enabled even higher protein production than Karikó and Weissman had generated, and Schrum's mRNAs lasted longer than either unmodified molecules or the modified mRNA the Penn academics had used, startling the young researcher. Working alone in a dreary basement and through intense pain, he had actually improved on the Penn professors' work.
Years later, Karikó and Weissman who would win acclaim. In September 2021, the scientists were awarded the Lasker-DeBakey Clinical Medical Research Award. Some predict they eventually will win a Nobel prize. But it would be Schrum's innovation that would form the backbone of both Moderna and Pfizer-BioNTech's Covid-19 vaccine, not the chemical modifications that Karikó and Weissman developed. For Schrum, necessity had truly been the mother of invention.
The Scientist Who Solved Delivery: Kerry Benenato
For several years, Moderna would make slow progress developing drugs to treat various diseases. Eventually, the company decided that mRNA was likely better suited for vaccines. By 2017, Moderna and the National Institutes of Health were discussing working together to develop mRNA–based vaccines, a partnership that buoyed Moderna's executives. There remained a huge obstacle in Moderna's way, however. It was up to Kerry Benenato to find a solution.
Benenato received an early hint of the hurdle in front of her three years earlier, when the organic chemist was first hired. When a colleague gave her a company tour, she was introduced to Moderna's chief scientific officer, Joseph Bolen, who seemed unusually excited to meet her.
"Oh, great!" Bolen said with a smile. "She's the one who's gonna solve delivery."
Bolen gave a hearty laugh and walked away, but Benenato detected seriousness in his quip.
Solve delivery?
It was a lot to expect from a 37-year-old scientist already dealing with insecurities and self-doubt. Benenato was an accomplished researcher who most recently had worked at AstraZeneca after completing post-doctoral studies at Harvard University. Despite her impressive credentials, Benenato battled a lack of confidence that sometimes got in her way. Performance reviews from past employers had been positive, but they usually produced similar critiques: Be more vocal. Do a better job advocating for your ideas. Give us more, Kerry.
Benenato was petite and soft-spoken. She sometimes stuttered or relied on "ums" and "ahs" when she became nervous, especially in front of groups, part of why she sometimes didn't feel comfortable speaking up.
"I'm an introvert," she says. "Self-confidence is something that's always been an issue."
To Benenato, Moderna's vaccine approach seemed promising—the team was packaging mRNAs in microscopic fatty-acid compounds called lipid nanoparticles, or LNPs, that protected the molecules on their way into cells. Moderna's shots should have been producing ample and long-lasting proteins. But the company's scientists were alarmed—they were injecting shots deep into the muscle of mice, but their immune systems were mounting spirited responses to the foreign components of the LNPs, which had been developed by a Canadian company.
This toxicity was a huge issue: A vaccine or drug that caused sharp pain and awful fevers wasn't going to prove very popular. The Moderna team was in a bind: Its mRNA had to be wrapped in the fatty nanoparticles to have a chance at producing plentiful proteins, but the body wasn't tolerating the microscopic encasements, especially upon repeated dosing.
The company's scientists had done everything they could to try to make the molecule's swathing material disappear soon after entering the cells, in order to avoid the unfortunate side effects, such as chills and headaches, but they weren't making headway. Frustration mounted. Somehow, the researchers had to find a way to get the encasements—made of little balls of fat, cholesterol, and other substances—to deliver their payload mRNA and then quickly vanish, like a parent dropping a teenager off at a party, to avoid setting off the immune system in unpleasant ways, even as the RNA and the proteins the molecule created stuck around.
Benenato wasn't entirely shocked by the challenges Moderna was facing. One of the reasons she had joined the upstart company was to help develop its delivery technology. She just didn't realize how pressing the issue was, or how stymied the researchers had become. Benenato also didn't know that Moderna board members were among those most discouraged by the delivery issue. In meetings, some of them pointed out that pharmaceutical giants like Roche Holding and Novartis had worked on similar issues and hadn't managed to develop lipid nanoparticles that were both effective and well tolerated by the body. Why would Moderna have any more luck?
Stephen Hoge insisted the company could yet find a solution.
"There's no way the only innovations in LNP are going to come from some academics and a small Canadian company," insisted Hoge, who had convinced the executives that hiring Benenato might help deliver an answer.
Benenato realized that while Moderna might have been a hot Boston-area start- up, it wasn't set up to do the chemistry necessary to solve their LNP problem. Much of its equipment was old or secondhand, and it was the kind used to tinker with mRNAs, not lipids.
"It was scary," she says.
When Benenato saw the company had a nuclear magnetic resonance spectrometer, which allows chemists to see the molecular structure of material, she let out a sigh of relief. Then Benenato inspected the machine and realized it was a jalopy. The hulking, aging instrument had been decommissioned and left behind by a previous tenant, too old and banged up to bring with them.
Benenato began experimenting with different chemical changes for Moderna's LNPs, but without a working spectrometer she and her colleagues had to have samples ready by noon each day, so they could be picked up by an outside company that would perform the necessary analysis. After a few weeks, her superiors received an enormous bill for the outsourced work and decided to pay to get the old spectrometer running again.
After months of futility, Benenato became impatient. An overachiever who could be hard on herself, she was eager to impress her new bosses. Benenato felt pressure outside the office, as well. She was married with a preschool-age daughter and an eighteen-month-old son. In her last job, Benenato's commute had been a twenty-minute trip to Astra-Zeneca's office in Waltham, outside Boston; now she was traveling an hour to Moderna's Cambridge offices. She became anxious—how was she going to devote the long hours she realized were necessary to solve their LNP quandary while providing her children proper care? Joining Moderna was beginning to feel like a possible mistake.
She turned to her husband and father for help. They reminded her of the hard work she had devoted to establishing her career and said it would be a shame if she couldn't take on the new challenge. Benenato's husband said he was happy to stay home with the kids, alleviating some of her concerns.
Back in the office, she got to work. She wanted to make lipids that were easier for the body to chop into smaller pieces, so they could be eliminated by the body's enzymes. Until then, Moderna, like most others, relied on all kinds of complicated chemicals to hold its LNP packaging together. They weren't natural, though, so the body was having a hard time breaking them down, causing the toxicity.
Benenato began experimenting with simpler chemicals. She inserted "ester bonds"—compounds referred to in chemical circles as "handles" because the body easily grabs them and breaks them apart. Ester bonds had two things going for them: They were strong enough to help ensure the LNP remained stable, acting much like a drop of oil in water, but they also gave the body's enzymes something to target and break down as soon as the LNP entered the cell, a way to quickly rid the body of the potentially toxic LNP components. Benenato thought the inclusion of these chemicals might speed the elimination of the LNP delivery material.
This idea, Benenato realized, was nothing more than traditional, medicinal chemistry. Most people didn't use ester bonds because they were pretty unsophisticated. But, hey, the tricky stuff wasn't working, so Benenato thought she'd see if the simple stuff worked.
Benenato also wanted to try to replace a group of unnatural chemicals in the LNP that was contributing to the spirited and unwelcome response from the immune system. Benenato set out to build a new and improved chemical combination. She began with ethanolamine, a colorless, natural chemical, an obvious start for any chemist hoping to build a more complex chemical combination. No one relied on ethanolamine on its own.
Benenato was curious, though. What would happen if she used just these two simple modifications to the LNP: ethanolamine with the ester bonds? Right away, Benenato noticed her new, super-simple compound helped mRNA create some protein in animals. It wasn't much, but it was a surprising and positive sign. Benenato spent over a year refining her solution, testing more than one hundred variations, all using ethanolamine and ester bonds, showing improvements with each new version of LNP. After finishing her 102nd version of the lipid molecule, which she named SM102, Benenato was confident enough in her work to show it to Hoge and others.
They immediately got excited. The team kept tweaking the composition of the lipid encasement. In 2017, they wrapped it around mRNA molecules and injected the new combination in mice and then monkeys. They saw plentiful, potent proteins were being produced and the lipids were quickly being eliminated, just as Benenato and her colleagues had hoped. Moderna had its special sauce.
That year, Benenato was asked to deliver a presentation to Stephane Bancel, Moderna's chief executive, Afeyan, and Moderna's executive committee to explain why it made sense to use the new, simpler LNP formulation for all its mRNA vaccines. She still needed approval from the executives to make the change. Ahead of the meeting, she was apprehensive, as some of her earlier anxieties returned. But an unusual calm came over her as she began speaking to the group. Benenato explained how experimenting with basic, overlooked chemicals had led to her discovery.
She said she had merely stumbled onto the company's solution, though her bosses understood the efforts that had been necessary for the breakthrough. The board complimented her work and agreed with the idea of switching to the new LNP. Benenato beamed with pride.
"As a scientist, serendipity has been my best friend," she told the executives.
Over the next few years, Benenato and her colleagues would improve on their methods and develop even more tolerable and potent LNP encasement for mRNA molecules. Their work enabled Moderna to include higher doses of vaccine in its shots. In early 2020, Moderna developed Covid-19 shots that included 100 micrograms of vaccine, compared with 30 micrograms in the Pfizer-BioNTech vaccine. That difference appears to help the Moderna vaccine generate higher titers and provide more protection.
"You set out in a career in drug discovery to want to make a difference," Benenato says. "Seeing it come to reality has been surreal and emotional."
Editor's Note: This essay is excerpted from A SHOT TO SAVE THE WORLD: The Inside Story of the Life-or-Death Race for a COVID-19 Vaccine by Gregory Zuckerman, now on sale from Portfolio/Penguin.
*Jason Schrum's arthritis is now in complete remission, thanks to Humira (adalimumab), a TNF-alpha blocker.