This Special Music Helped Preemie Babies’ Brains Develop
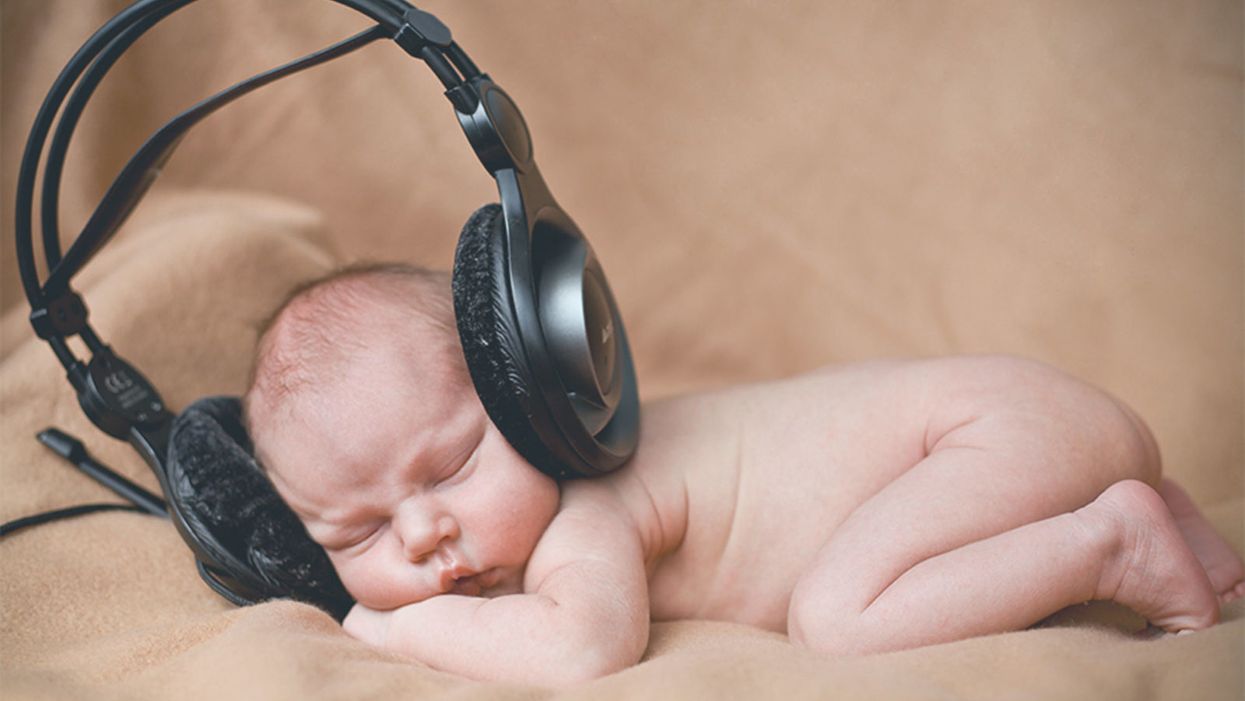
Listening to music helped preterm babies' brains develop, according to the results of a new Swiss study.
Move over, Baby Einstein: New research from Switzerland shows that listening to soothing music in the first weeks of life helps encourage brain development in preterm babies.
For the study, the scientists recruited a harpist and a new-age musician to compose three pieces of music.
The Lowdown
Children who are born prematurely, between 24 and 32 weeks of pregnancy, are far more likely to survive today than they used to be—but because their brains are less developed at birth, they're still at high risk for learning difficulties and emotional disorders later in life.
Researchers in Geneva thought that the unfamiliar and stressful noises in neonatal intensive care units might be partially responsible. After all, a hospital ward filled with alarms, other infants crying, and adults bustling in and out is far more disruptive than the quiet in-utero environment the babies are used to. They decided to test whether listening to pleasant music could have a positive, counterbalancing effect on the babies' brain development.
Led by Dr. Petra Hüppi at the University of Geneva, the scientists recruited Swiss harpist and new-age musician Andreas Vollenweider (who has collaborated with the likes of Carly Simon, Bryan Adams, and Bobby McFerrin). Vollenweider developed three pieces of music specifically for the NICU babies, which were played for them five times per week. Each track was used for specific purposes: To help the baby wake up; to stimulate a baby who was already awake; and to help the baby fall back asleep.
When they reached an age equivalent to a full-term baby, the infants underwent an MRI. The researchers focused on connections within the salience network, which determines how relevant information is, and then processes and acts on it—crucial components of healthy social behavior and emotional regulation. The neural networks of preemies who had listened to Vollenweider's pieces were stronger than preterm babies who had not received the intervention, and were instead much more similar to full-term babies.
Next Up
The first infants in the study are now 6 years old—the age when cognitive problems usually become diagnosable. Researchers plan to follow up with more cognitive and socio-emotional assessments, to determine whether the effects of the music intervention have lasted.
The first infants in the study are now 6 years old—the age when cognitive problems usually become diagnosable.
The scientists note in their paper that, while they saw strong results in the babies' primary auditory cortex and thalamus connections—suggesting that they had developed an ability to recognize and respond to familiar music—there was less reaction in the regions responsible for socioemotional processing. They hypothesize that more time spent listening to music during a NICU stay could improve those connections as well; but another study would be needed to know for sure.
Open Questions
Because this initial study had a fairly small sample size (only 20 preterm infants underwent the musical intervention, with another 19 studied as a control group), and they all listened to the same music for the same amount of time, it's still undetermined whether variations in the type and frequency of music would make a difference. Are Vollenweider's harps, bells, and punji the runaway favorite, or would other styles of music help, too? (Would "Baby Shark" help … or hurt?) There's also a chance that other types of repetitive sounds, like parents speaking or singing to their children, might have similar effects.
But the biggest question is still the one that the scientists plan to tackle next: Whether the intervention lasts as the children grow up. If it does, that's great news for any family with a preemie — and for the baby-sized headphone industry.
This Mom Donated Her Lost Baby’s Tissue to Research
Sarah Gray views data about the use of her deceased son Thomas' retinal tissue in research for retinoblastoma, a cancer of the eye, at the University of Pennsylvania, while her son Callum looks on.
The twin boys growing within her womb filled Sarah Gray with both awe and dread. The sonogram showed that one, Callum, seemed to be the healthy child she and husband Ross had long sought; the other, Thomas, had anencephaly, a fatal developmental disorder of the skull and brain that likely would limit his life to hours. The options were to carry the boys to term or terminate both.
The decision to donate Thomas' tissue to research comforted Sarah. It brought a sense of purpose and meaning to her son's anticipated few breaths.
Sarah learned that researchers prize tissue as essential to better understanding and eventually treating the rare disorder that afflicted her son. And that other tissue from the developing infant might prove useful for transplant or basic research.
Animal models have been useful in figuring out some of the basics of genetics and how the body responds to disease. But a mouse is not a man. The new tools of precision medicine that measure gene expression, proteins and metabolites – the various chemical products and signals that fluctuate in health and illness – are most relevant when studying human tissue directly rather than in animals.
The decision to donate Thomas' tissue to research comforted Sarah. It brought a sense of purpose and meaning to her son's anticipated few breaths.
Thomas Gray
(Photo credit: Mark Walpole)
Later Sarah would track down where some of the donated tissues had been sent and how they were being used. It was a rare initiative that just may spark a new kind of relationship between donor families and researchers who use human tissue.
Organ donation for transplant gets all the attention. That process is simple, direct, life saving, the stories are easy to understand and play out regularly in the media. Reimbursement fully covers costs.
Tissue donation for research is murkier. Seldom is there a direct one-to-one correlation between individual donation and discovery; often hundreds, sometimes thousands of samples are needed to tease out the basic mechanisms of a disease, even more to develop a treatment or cure. The research process can be agonizingly slow. And somebody has to pay for collecting, processing, and getting donations into the hands of appropriate researchers. That story rarely is told, so most people are not even aware it is possible, let alone vital to research.
Gray set out on a quest to follow where Thomas' tissue had gone and how it was being used to advance research and care.
The dichotomy between transplant and research became real for Sarah several months after the birth of her twins, and Thomas' brief life, at a meeting for families of transplant donors. Many of the participants had found closure to their grieving through contact with grateful recipients of a heart, liver, or kidney who had gained a new lease on life. But there was no similar process for those who donated for research. Sarah felt a bit, well, jealous. She wanted that type of connection too.
Gray set out on a quest to follow where Thomas' tissue had gone and how it was being used to advance research and care. Those encounters were as novel for the researchers as they were for Sarah. The experience turned her into an advocate for public education and financial and operational changes to put tissue donation for research on par with donations for transplant.
Thomas' retina had been collected and processed by the National Disease Research Interchange (NDRI), a nonprofit that performs such services for researchers on a cost recovery basis with support from the National Institutes of Health. The tissue was passed on to Arupa Ganguly, who is studying retinoblastoma, a cancer of the eye, at the University of Pennsylvania.
Ganguly was surprised and apprehensive months later when NDRI emailed her saying the mother of donated tissue wanted to learn more about how the retina was being used. That was unusual because research donations generally are anonymous.
The geneticist waited a day or two, then wrote an explanation of her work and forwarded it back through NDRI. Soon the researcher and mother were talking by phone and Sarah would visit the lab. Even then, Ganguly felt very uncomfortable. "Something very bad happened to your son Thomas but it was a benefit for me, so I'm feeling very bad," she told Sarah.
"And Sarah said, Arupa, you were the only ones who wanted his retinas. If you didn't request them, they would be buried in the ground. It gives me a sense of fulfillment to know that they were of some use," Ganguly recalls. And her apprehension melted away. The two became friends and have visited several times.
Sarah Gray visits Dr. Arupa Ganguly at the University of Pennsylvania's Genetic Diagnostic Laboratory.
(Photo credit: Daniel Burke)
Reading Sarah Gray's story led Gregory Grossman to reach out to the young mother and to create Hope and Healing, a program that brings donors and researchers together. Grossman is director of research programs at Eversight, a large network of eye banks that stretches from the Midwest to the East Coast. It supplies tissue for transplant and ocular research.
"Research seems a cold and distant thing," Grossman says, "we need to educate the general public on the importance and need for tissue donations for research, which can help us better understand disease and find treatments."
"Our own internal culture needs to be shifted too," he adds. "Researchers and surgeons can forget that these are precious gifts, they're not a commodity, they're not manufactured. Without people's generosity this doesn't exist."
The initial Hope and Healing meetings between researchers and donor families have gone well and Grossman hopes to increase them to three a year with support from the Lions Club. He sees it as a crucial element in trying to reverse the decline in ocular donations even while research needs continue to grow.
What people hear about is "Tuskegee, Henrietta Lacks, they hear about the scandals, they don't hear about the good news. I would like to change that."
Since writing about her experience in the 2016 book "A Life Everlasting," Gray has come to believe that potential donor families, and even people who administer donation programs, often are unaware of the possibility of donating for research.
And roadblocks are common for those who seek to do so. Just like her, many families have had to be persistent in their quest to donate, and even educate their medical providers. But Sarah believes the internet is facilitating creation of a grassroots movement of empowered donors who are pushing procurement systems to be more responsive to their desires to donate for research. A lot of it comes through anecdote, stories, and people asking, if they have done it in Virginia, or Ohio, why can't we do it here?
Callum Gray and Dr. Arupa Ganguly hug during his family's visit to the lab.
(Photo credit: Daniel Burke)
Gray has spoken at medical and research facilities and at conferences. Some researchers are curious to have contact with the families of donors, but she believes the research system fosters the belief that "you don't want to open that can of worms." And lurking in the background may be a fear of liability issues somehow arising.
"I believe that 99 percent of what happens in research is very positive, and those stories would come out if the connections could be made," says Sarah Gray. But what they hear about is "Tuskegee, Henrietta Lacks, they hear about the scandals, they don't hear about the good news. I would like to change that."
Sloppy Science Happens More Than You Think
Manipulating DNA through gene editing.
The media loves to tout scientific breakthroughs, and few are as toutable – and in turn, have been as touted – as CRISPR. This method of targeted DNA excision was discovered in bacteria, which use it as an adaptive immune system to combat reinfection with a previously encountered virus.
Shouldn't the editors at a Nature journal know better than to have published an incorrect paper in the first place?
It is cool on so many levels: not only is the basic function fascinating, reminding us that we still have more to discover about even simple organisms that we thought we knew so well, but the ability it grants us to remove and replace any DNA of interest has almost limitless applications in both the lab and the clinic. As if that didn't make it sexy enough, add in a bicoastal, male-female, very public and relatively ugly patent battle, and the CRISPR story is irresistible.
And then last summer, a bombshell dropped. The prestigious journal Nature Methods published a paper in which the authors claimed that CRISPR could cause many unintended mutations, rendering it unfit for clinical use. Havoc duly ensued; stocks in CRISPR-based companies plummeted. Thankfully, the authors of the offending paper were responsible, good scientists; they reassessed, then recanted. Their attention- and headline- grabbing results were wrong, and they admitted as much, leading Nature Methods to formally retract the paper this spring.
How did this happen? Shouldn't the editors at a Nature journal know better than to have published this in the first place?
Alas, high-profile scientific journals publish misleading and downright false results fairly regularly. Some errors are unavoidable – that's how the scientific method works. Hypotheses and conclusions will invariably be overturned as new data becomes available and new technologies are developed that allow for deeper and deeper studies. That's supposed to happen. But that's not what we're talking about here. Nor are we talking about obvious offenses like outright plagiarism. We're talking about mistakes that are avoidable, and that still have serious ramifications.
The cultures of both industry and academia promote research that is poorly designed and even more poorly analyzed.
Two parties are responsible for a scientific publication, and thus two parties bear the blame when things go awry: the scientists who perform and submit the work, and the journals who publish it. Unfortunately, both are incentivized for speedy and flashy publications, and not necessarily for correct publications. It is hardly a surprise, then, that we end up with papers that are speedy and flashy – and not necessarily correct.
"Scientists don't lie and submit falsified data," said Andy Koff, a professor of Molecular Biology at Sloan Kettering Institute, the basic research arm of Memorial Sloan Kettering Cancer Center. Richard Harris, who wrote the book on scientific misconduct running the gamut from unconscious bias and ignorance to more malicious fraudulence, largely concurs (full disclosure: I reviewed the book here). "Scientists want to do good science and want to be recognized as such," he said. But even so, the cultures of both industry and academia promote research that is poorly designed and even more poorly analyzed. In Rigor Mortis: How Sloppy Science Creates Worthless Cures, Crushes Hope, and Wastes Millions, Harris describes how scientists must constantly publish in order to maintain their reputations and positions, to get grants and tenure and students. "They are disincentivized from doing that last extra experiment to prove their results," he said; it could prove too risky if it could cost them a publication.
Ivan Oransky and Adam Marcus founded Retraction Watch, a blog that tracks the retraction of scientific papers, in 2010. Oransky pointed out that blinded peer review – the pride and joy of the scientific publishing enterprise – is a large part of the problem. "Pre-publication peer review is still important, but we can't treat it like the only check on the system. Papers are being reviewed by non-experts, and reviewers are asked to review papers only tangentially related to their field. Moreover, most peer reviewers don't look at the underlying or raw data, even when it is available. How then can they tell if the analysis is flawed or the data is accurate?" he wondered.
Mistaken publications also erode the public's opinion of legitimate science, which is problematic since that opinion isn't especially high to begin with.
Koff agreed that anonymous peer review is valuable, but severely flawed. "Blinded review forces a collective view of importance," he said. "If an article disagrees with the reviewer's worldview, the article gets rejected or forced to adhere to that worldview – even if that means pushing the data someplace it shouldn't necessarily go." We have lost the scientific principle behind review, he thinks, which was to critically analyze a paper. But instead of challenging fundamental assumptions within a paper, reviewers now tend to just ask for more and more supplementary data. And don't get him started on editors. "Editors are supposed to arbitrate between reviewers and writers and they have completely abdicated this responsibility, at every journal. They do not judge, and that's a real failing."
Harris laments the wasted time, effort, and resources that result when erroneous ideas take hold in a field, not to mention lives lost when drug discovery is predicated on basic science findings that end up being wrong. "When no one takes the time, care, and money to reproduce things, science isn't stopping – but it is slowing down," he noted. Mistaken publications also erode the public's opinion of legitimate science, which is problematic since that opinion isn't especially high to begin with.
Scientists and publishers don't only cause the problem, though – they may also provide the solution. Both camps are increasingly recognizing and dealing with the crisis. The self-proclaimed "data thugs" Nick Brown and James Heathers use pretty basic arithmetic to reveal statistical errors in papers. The microbiologist Elisabeth Bik scans the scientific literature for problematic images "in her free time." The psychologist Brian Nosek founded the Center for Open Science, a non-profit organization dedicated to promoting openness, integrity, and reproducibility in scientific research. The Nature family of journals – yes, the one responsible for the latest CRISPR fiasco – has its authors complete a checklist to combat irreproducibility, à la Atul Gawande. And Nature Communications, among other journals, uses transparent peer review, in which authors can opt to have the reviews of their manuscript published anonymously alongside the completed paper. This practice "shows people how the paper evolved," said Koff "and keeps the reviewer and editor accountable. Did the reviewer identify the major problems with the paper? Because there are always major problems with a paper."