Can Genetic Testing Help Shed Light on the Autism Epidemic?
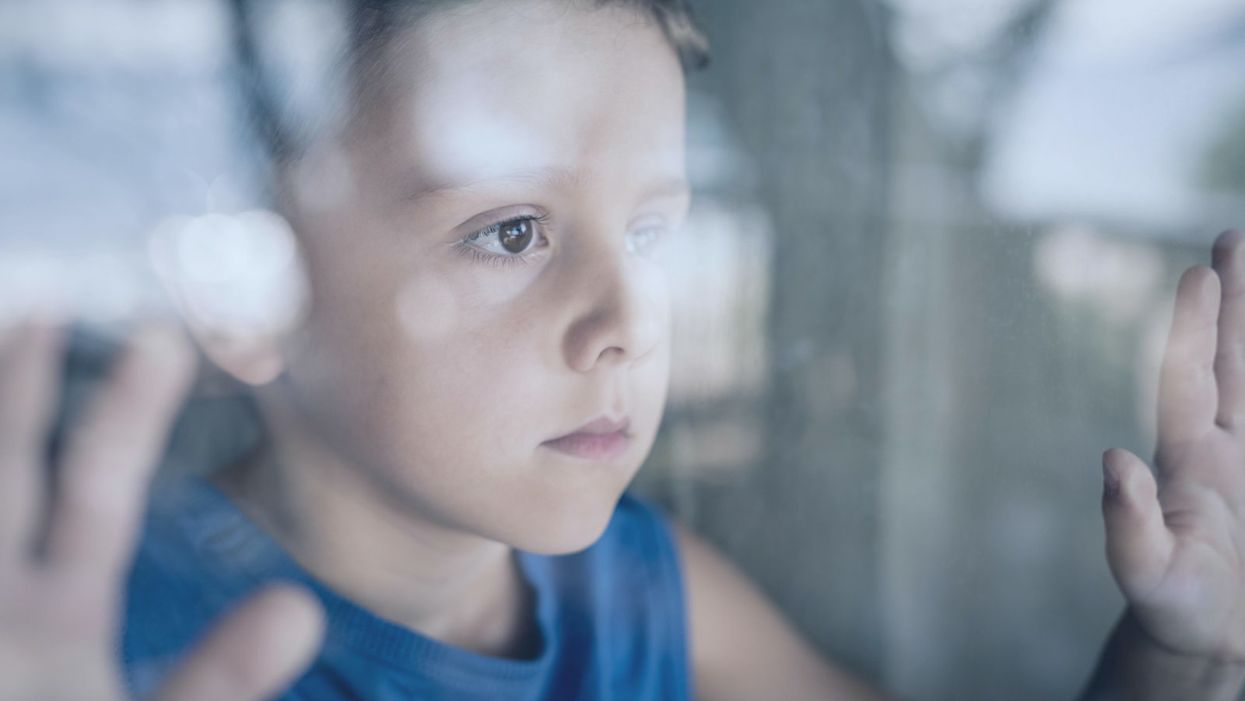
A little boy standing by a window in contemplation. (© altanaka/Fotolia)
Autism cases are still on the rise, and scientists don't know why. In April, the Centers for Disease Control (CDC) reported that rates of autism had increased once again, now at an estimated 1 in 59 children up from 1 in 68 just two years ago. Rates have been climbing steadily since 2007 when the CDC initially estimated that 1 in 150 children were on the autism spectrum.
Some clinicians are concerned that the creeping expansion of autism is causing the diagnosis to lose its meaning.
The standard explanation for this increase has been the expansion of the definition of autism to include milder forms like Asperger's, as well as a heightened awareness of the condition that has improved screening efforts. For example, the most recent jump is attributed to children in minority communities being diagnosed who might have previously gone under the radar. In addition, more federally funded resources are available to children with autism than other types of developmental disorders, which may prompt families or physicians to push harder for a diagnosis.
Some clinicians are concerned that the creeping expansion of autism is causing the diagnosis to lose its meaning. William Graf, a pediatric neurologist at Connecticut Children's Medical Center, says that when a nurse tells him that a new patient has a history of autism, the term is no longer a useful description. "Even though I know this topic extremely well, I cannot picture the child anymore," he says. "Use the words mild, moderate, or severe. Just give me a couple more clues, because when you say autism today, I have no idea what people are talking about anymore."
Genetic testing has emerged as one potential way to remedy the overly broad label by narrowing down a heterogeneous diagnosis to a specific genetic disorder. According to Suma Shankar, a medical geneticist at the University of California, Davis, up to 60 percent of autism cases could be attributed to underlying genetic causes. Common examples include Fragile X Syndrome or Rett Syndrome—neurodevelopmental disorders that are caused by mutations in individual genes and are behaviorally classified as autism.
With more than 500 different mutations associated with autism, very few additional diagnoses provide meaningful information.
Having a genetic diagnosis in addition to an autism diagnosis can help families in several ways, says Shankar. Knowing the genetic origin can alert families to other potential health problems that are linked to the mutation, such as heart defects or problems with the immune system. It may also help clinicians provide more targeted behavioral therapies and could one day lead to the development of drug treatments for underlying neurochemical abnormalities. "It will pave the way to begin to tease out treatments," Shankar says.
When a doctor diagnoses a child as having a specific genetic condition, the label of autism is still kept because it is more well-known and gives the child access to more state-funded resources. Children can thus be diagnosed with multiple conditions: autism spectrum disorder and their specific gene mutation. However, with more than 500 different mutations associated with autism, very few additional diagnoses provide meaningful information. What's more, the presence or absence of a mutation doesn't necessarily indicate whether the child is on the mild or severe end of the autism spectrum.
Because of this, Graf doubts that genetic classifications are really that useful. He tells the story of a boy with epilepsy and severe intellectual disabilities who was diagnosed with autism as a young child. Years later, Graf ordered genetic testing for the boy and discovered that he had a mutation in the gene SYNGAP1. However, this knowledge didn't change the boy's autism status. "That diagnosis [SYNGAP1] turns out to be very specific for him, but it will never be a household name. Biologically it's good to know, and now it's all over his chart. But on a societal level he still needs this catch-all label [of autism]," Graf says.
"It gives some information, but to what degree does that change treatment or prognosis?"
Jennifer Singh, a sociologist at Georgia Tech who wrote the book Multiple Autisms: Spectrums of Advocacy and Genomic Science, agrees. "I don't know that the knowledge gained from just having a gene that's linked to autism," is that beneficial, she says. "It gives some information, but to what degree does that change treatment or prognosis? Because at the end of the day you have to address the issues that are at hand, whatever they might be."
As more children are diagnosed with autism, knowledge of the underlying genetic mutation causing the condition could help families better understand the diagnosis and anticipate their child's developmental trajectory. However, for the vast majority, an additional label provides little clarity or consolation.
Instead of spending money on genetic screens, Singh thinks the resources would be better used on additional services for people who don't have access to behavioral, speech, or occupational therapy. "Things that are really going to matter for this child in their future," she says.
A doctor cradles a newborn who is sick with measles.
Ethan Lindenberger, the Ohio teenager who sought out vaccinations after he was denied them as a child, recently testified before Congress about why his parents became anti-vaxxers. The trouble, he believes, stems from the pervasiveness of misinformation online.
There is evidence that 'educating' people with facts about the benefits of vaccination may not be effective.
"For my mother, her love and affection and care as a parent was used to push an agenda to create a false distress," he told the Senate Committee. His mother read posts on social media saying vaccines are dangerous, and that was enough to persuade her against them.
His story is an example of how widespread and harmful the current discourse on vaccinations is—and more importantly—how traditional strategies to convince people about the merits of vaccination have largely failed.
As responsible members of society, all of us have implicitly signed on to what ethicists call the "Social Contract" -- we agree to abide by certain moral and political rules of behavior. This is what our societal values, norms, and often governments are based upon. However, with the unprecedented rise of social media, alternative facts, and fake news, it is evident that our understanding—and application—of the social contract must also evolve.
Nowhere is this breakdown of societal norms more visible than in the failure to contain the spread of vaccine-preventable diseases like measles. What started off as unexplained episodes in New York City last October, mostly in communities that are under-vaccinated, has exploded into a national epidemic: 880 cases of measles across 24 states in 2019, according to the CDC (as of May 17, 2019). In fact, the Unites States is only eight months away from losing its "measles free" status, joining Venezuela as the second country out of North and South America with that status.
The U.S. is not the only country facing this growing problem. Such constant and perilous reemergence of measles and other vaccine-preventable diseases in various parts of the world raises doubts about the efficacy of current vaccination policies. In addition to the loss of valuable life, these outbreaks lead to loss of millions of dollars in unnecessary expenditure of scarce healthcare resources. While we may be living through an age of information, we are also navigating an era whose hallmark is a massive onslaught on truth.
There is ample evidence on how these outbreaks start: low-vaccination rates. At the same time, there is evidence that 'educating' people with facts about the benefits of vaccination may not be effective. Indeed, human reasoning has a limit, and facts alone rarely change a person's opinion. In a fascinating report by researchers from the University of Pennsylvania, a small experiment revealed how "behavioral nudges" could inform policy decisions around vaccination.
In the reported experiment, the vaccination rate for employees of a company increased by 1.5 percent when they were prompted to name the date when they planned to get their flu shot. In the same experiment, when employees were prompted to name both a date and a time for their planned flu shot, vaccination rate increased by 4 percent.
A randomized trial revealed the subtle power of "announcements" – direct, brief, assertive statements by physicians that assumed parents were ready to vaccinate their children.
This experiment is a part of an emerging field of behavioral economics—a scientific undertaking that uses insights from psychology to understand human decision-making. The field was born from a humbling realization that humans probably do not possess an unlimited capacity for processing information. Work in this field could inform how we can formulate vaccination policy that is effective, conserves healthcare resources, and is applicable to current societal norms.
Take, for instance, the case of Human Papilloma Virus (HPV) that can cause several types of cancers in both men and women. Research into the quality of physician communication has repeatedly revealed how lukewarm recommendations for HPV vaccination by primary care physicians likely contributes to under-immunization of eligible adolescents and can cause confusion for parents.
A randomized trial revealed the subtle power of "announcements" – direct, brief, assertive statements by physicians that assumed parents were ready to vaccinate their children. These announcements increased vaccination rates by 5.4 percent. Lengthy, open-ended dialogues demonstrated no benefit in vaccination rates. It seems that uncertainty from the physician translates to unwillingness from a parent.
Choice architecture is another compelling concept. The premise is simple: We hardly make any of our decisions in vacuum; the environment in which these decisions are made has an influence. If health systems were designed with these insights in mind, people would be more likely to make better choices—without being forced.
This theory, proposed by Richard Thaler, who won the 2017 Nobel Prize in Economics, was put to the test by physicians at the University of Pennsylvania. In their study, flu vaccination rates at primary care practices increased by 9.5 percent all because the staff implemented "active choice intervention" in their electronic health records—a prompt that nudged doctors and nurses to ask patients if they'd gotten the vaccine yet. This study illustrated how an intervention as simple as a reminder can save lives.
To be sure, some bioethicists do worry about implementing these policies. Are behavioral nudges akin to increased scrutiny or a burden for the disadvantaged? For example, would incentives to quit smoking unfairly target the poor, who are more likely to receive criticism for bad choices?
The measles outbreak is a sober reminder of how devastating it can be when the social contract breaks down.
While this is a valid concern, behavioral economics offers one of the only ethical solutions to increasing vaccination rates by addressing the most critical—and often legal—challenge to universal vaccinations: mandates. Choice architecture and other interventions encourage and inform a choice, allowing an individual to retain his or her right to refuse unwanted treatment. This distinction is especially important, as evidence suggests that people who refuse vaccinations often do so as a result of cognitive biases – systematic errors in thinking resulting from emotional attachment or a lack of information.
For instance, people are prone to "confirmation bias," or a tendency to selectively believe in information that confirms their preexisting theories, rather than the available evidence. At the same time, people do not like mandates. In such situations, choice architecture provides a useful option: people are nudged to make the right choice via the design of health delivery systems, without needing policies that rely on force.
The measles outbreak is a sober reminder of how devastating it can be when the social contract breaks down and people fall prey to misinformation. But all is not lost. As we fight a larger societal battle against alternative facts, we now have another option in the trenches to subtly encourage people to make better choices.
Using insights from research in decision-making, we can all contribute meaningfully in controversial conversations with family, friends, neighbors, colleagues, and our representatives — and push for policies that protect those we care about. A little more than a hundred years ago, thousands of lives were routinely lost to preventive illnesses. We've come too far to let ignorance destroy us now.
New Tech Can Predict Breast Cancer Years in Advance
Breast cancer survivors rally in a support group.
Every two minutes, a woman is diagnosed with breast cancer. The question is, can those at high risk be identified early enough to survive?
New AI software has predicted risk equally well in both white and black women for the first time.
The current standard practice in medicine is not exactly precise. It relies on age, family history of cancer, and breast density, among other factors, to determine risk. But these factors do not always tell the whole story, leaving many women to slip through the cracks. In addition, a racial gap persists in breast cancer treatment and survival. African-American women are 42 percent more likely to die from the disease despite relatively equal rates of diagnosis.
But now those grim statistics could be changing. A team of researchers from MIT's Computer Science and Artificial Intelligence Laboratory have developed a deep learning model that can more accurately predict a patient's breast cancer risk compared to established clinical guidelines – and it has predicted risk equally well in both white and black women for the first time.
The Lowdown
Study results published in Radiology described how the AI software read mammogram images from more than 60,000 patients at Massachusetts General Hospital to identify subtle differences in breast tissue that pointed to potential risk factors, even in their earliest stages. The team accessed the patients' actual diagnoses and determined that the AI model was able to correctly place 31 percent of all cancer patients in the highest-risk category of developing breast cancer within five years of the examination, compared to just 18 percent for existing models.
"Each image has hundreds of thousands of pixels identifying something that may not necessarily be detected by the human eye," said MIT professor Regina Barzilay, one of the study's lead authors. "We all have limited visual capacities so it seems some machines trained on hundreds of thousands of images with a known outcome can capture correlations the human eye might not notice."
Barzilay, a breast cancer survivor herself, had abnormal tissue patterns on mammograms in 2012 and 2013, but wasn't diagnosed until after a 2014 image reading, illustrating the limitations of human processing alone.
MIT professor Regina Barzilay, a lead author on the new study and a breast cancer survivor herself.
(Courtesy MIT)
Next up: The MIT team is looking at training the model to detect other cancers and health risks. Barzilay recalls how a cardiologist told her during a conference that women with heart diseases had a different pattern of calcification on their mammograms, demonstrating how already existing images can be used to extract other pieces of information about a person's health status.
Integration of the AI model in standard care could help doctors better tailor screening and prevention programs based on actual instead of perceived risk. Patients who might register as higher risk by current guidelines could be identified as lower risk, helping resolve conflicting opinions about how early and how often women should receive mammograms.
Open Questions: While the results were promising, it's unknown how well the model will work on a larger scale, as the study looked at data from just one institution and used mammograms supplied by just one hospital. Some risk factor information was also unavailable for certain patients during the study, leaving researchers unable to fully compare the AI model's performance to that of the traditional standard.
One incentive to wider implementation and study, however, is the bonus that no new hardware is required to use the AI model. With other institutions now showing interest, this software could lead to earlier routine detection and treatment of breast cancer — resulting in more lives saved.